Fractional Order Backpropagation Neural Network for Battery Capacity Estimation with Realistic Vehicle Data
2022 18th IEEE/ASME International Conference on Mechatronic and Embedded Systems and Applications (MESA)(2022)
摘要
For battery capacity estimation, machine learning (ML) algorithm has drawn much attention in the intelligent battery management field. The state-of-art ML algorithm cannot describe the inside reactions of LIBs, while electrochemical model with complicated partial differential equations cannot be deployed to realistic applications. On the basis of fractional-order calculus, this paper proposes a fractional-order backpropagation neural network (BPNN) for the capacity estimation. As an enhanced ML algorithm, the proposed fractional-order BPNN combines the fractional-order ML theory with the fractional-order modeling of LIBs, which can reflect the battery diffusion dynamics. The fractional-order gradient is introduced to the gradient descent method, constructing a fractional-order gradient descent (FOGD) method for weight update in backpropagation process. A set of 17 electric vehicles (EVs) data are collected and preprocessed to verify the capacity estimation effects of the proposed algorithm. For the realistic battery data without capacity labels, this paper firstly deduces and provides “pseudo” labels for the algorithm training, then the proposed fractional-order BPNN is trained with FOGD method to learn battery capacity changes. The experiment results show that the fractional-order BPNN can learn the battery degradation trend and maintain estimation accuracy within 4.5% for the whole capacity curve during battery lifetime.
更多查看译文
关键词
fractional-order gradient,battery degradation,capacity estimation,machine learning,backpropagation
AI 理解论文
溯源树
样例
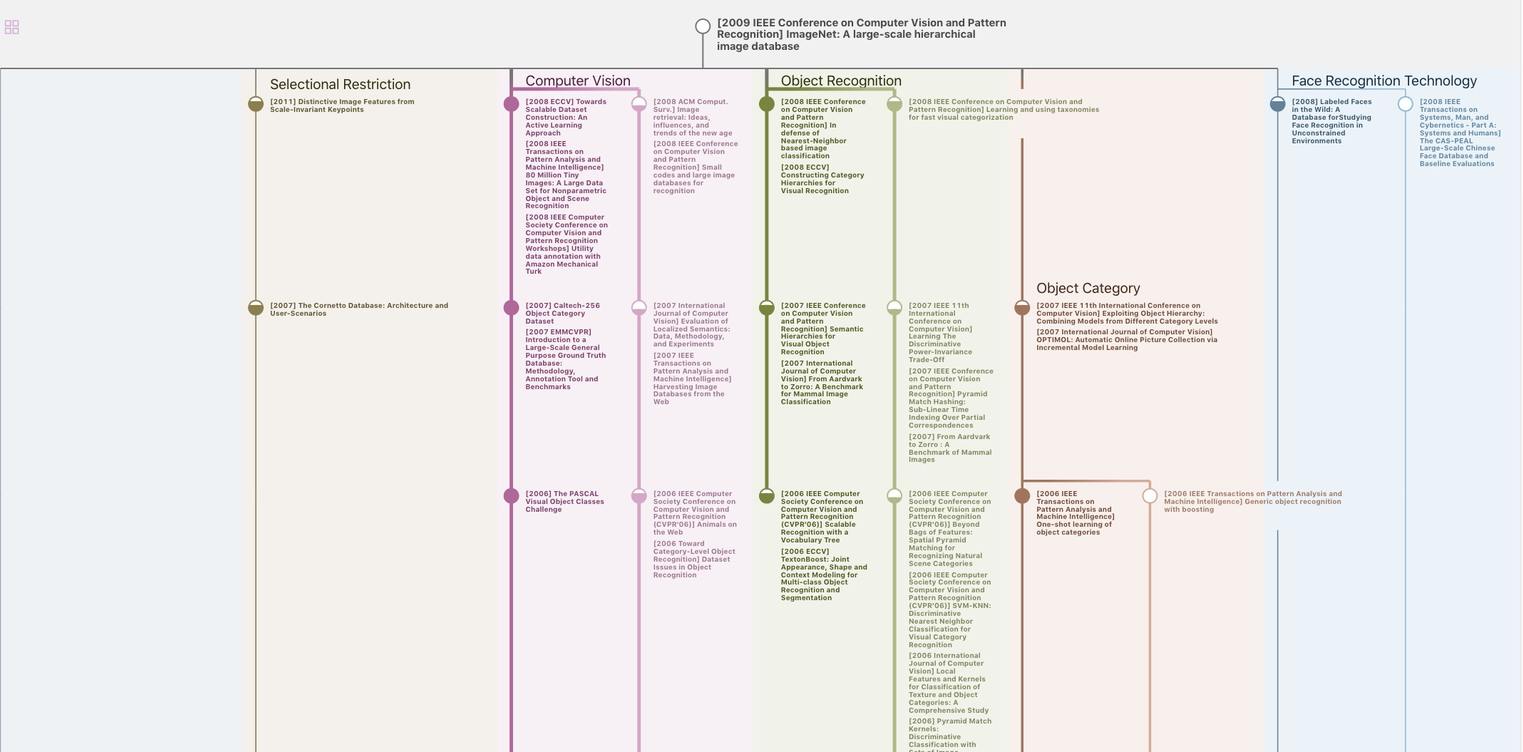
生成溯源树,研究论文发展脉络
Chat Paper
正在生成论文摘要