A Parallel Hybrid Neural Network With Integration of Spatial and Temporal Features for Remaining Useful Life Prediction in Prognostics
IEEE Transactions on Instrumentation and Measurement(2023)
摘要
Prediction of remaining useful life (RUL) is an indispensable part of prognostics health management (PHM) in complex systems. Considering the parallel integration of the spatial and temporal features implicated in measurement data, this article proposes a novel parallel hybrid neural network that consists of 1-D convolutional neural network (1-DCNN) and bidirectional gated recurrent unit (BiGRU) to predict RUL. Specifically, the spatial and temporal information from historical data is parallel extracted with the aid of 1-DCNN and BiGRU, respectively. On this basis, the trained network can be applied for RUL prediction in real time. The proposed parallel hybrid neural network is evaluated by two public datasets, in detail, an aircraft turbofan engine dataset and a milling dataset. Experimental results demonstrate that the proposed parallel hybrid network can effectively predict the RUL, which outperforms the existing literature.
更多查看译文
关键词
1-D convolutional neural network (1-DCNN),bidirectional gated recurrent unit (BiGRU),parallel hybrid neural network,prediction,prognostics health management (PHM),remaining useful life (RUL)
AI 理解论文
溯源树
样例
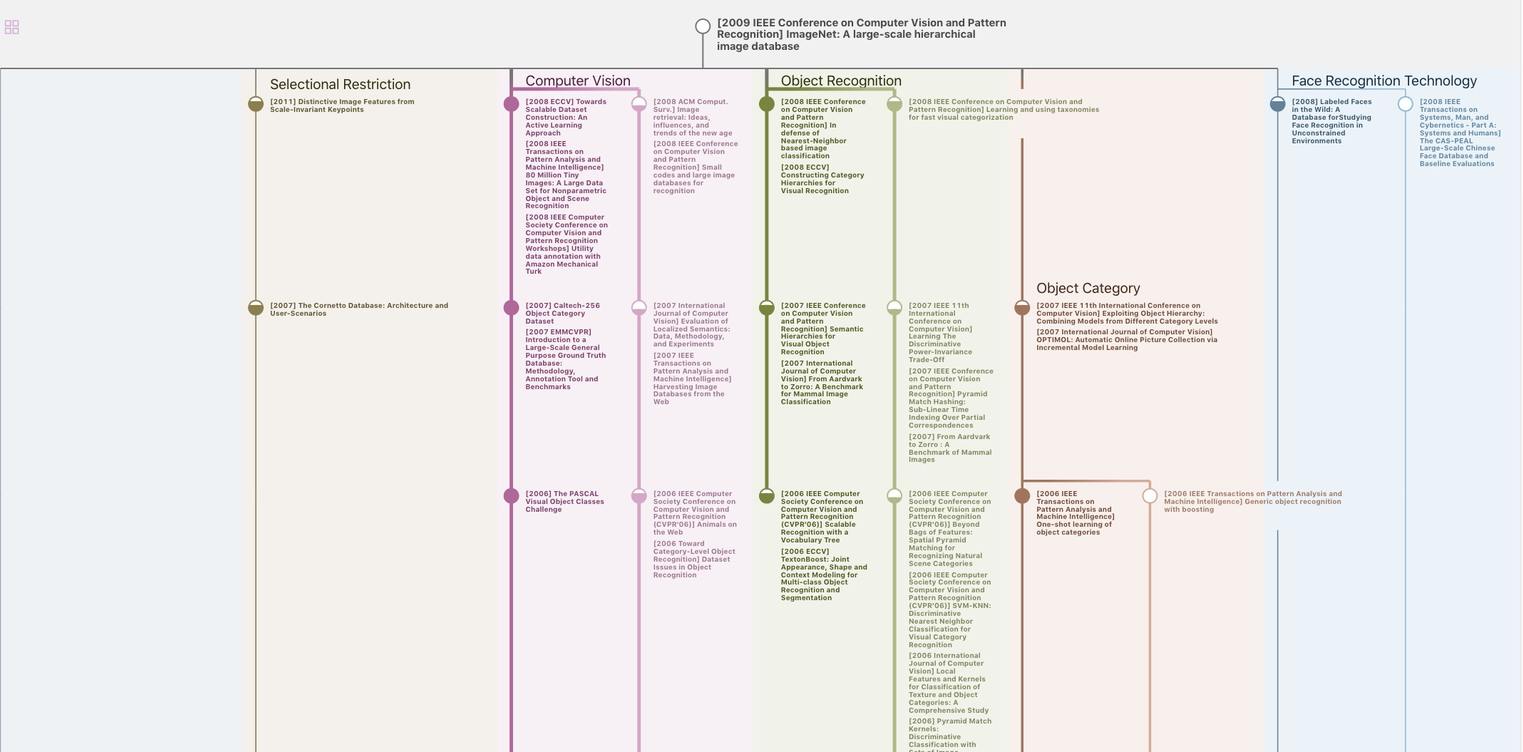
生成溯源树,研究论文发展脉络
Chat Paper
正在生成论文摘要