Assessing seismic-like events prediction in model knits with unsupervised machine learning
Extreme Mechanics Letters(2023)
摘要
Knitted fabric exhibits avalanche-like events when deformed, which we are interested in predicting, by analogy with earthquakes. However, as in most analogous seismic models, the time intermittence and scale-invariance of these events severely jeopardize this endeavor. But more importantly, such predictions are hard to assess and not easily compared. Here we introduce a framework that allows not only to predict seismic-like, rebalanced time series, but to also evaluate and compare the relevance of competing predictions. It relies on a reinforcement learning environment that learns risk management in a model, seismically active city subjected to the crackling dynamics observed in the mechanical response of knitted fabric. Relying on extensive experimental data, we show that this mechanism allows to assess the finite predictability of seismic-like activity, and to compare the performance of different approaches in the operational usage of such predictions. (c) 2022 Elsevier Ltd. All rights reserved.
更多查看译文
关键词
Crackling noise,Knitted fabric,Seismicity,Machine learning
AI 理解论文
溯源树
样例
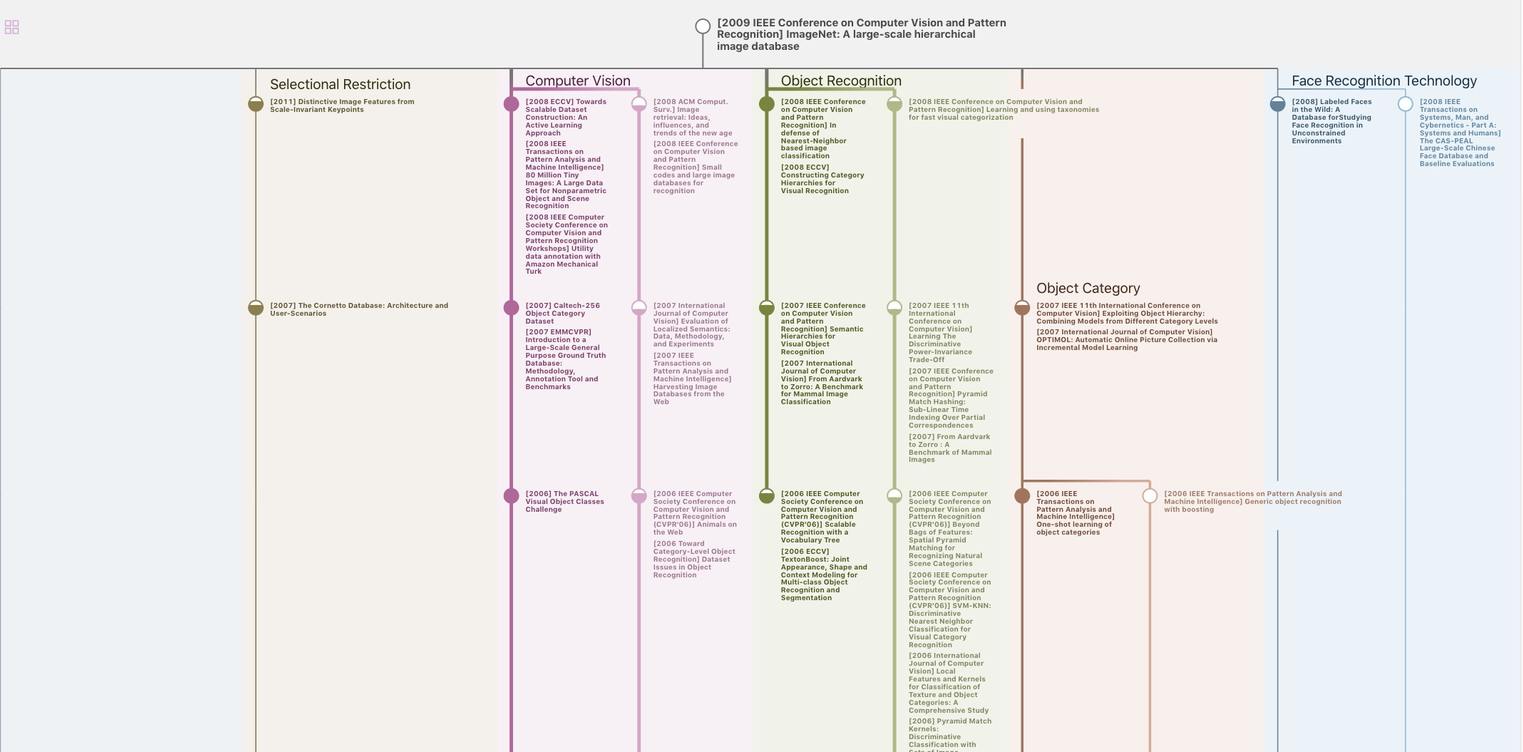
生成溯源树,研究论文发展脉络
Chat Paper
正在生成论文摘要