Multi-agent quantum-inspired deep reinforcement learning for real-time distributed generation control of 100% renewable energy systems
Engineering Applications of Artificial Intelligence(2023)
摘要
With promoting peaking carbon emissions and achieving carbon neutrality, the real-time distributed control of the prosumers of 100% renewable energy systems (RESs) is challenging. This paper proposes multi-agent quantum-inspired deep reinforcement learning (QDRL) approaches for real-time distributed generation control of 100% RESs. Quantum-inspired operation is introduced into deep reinforcement learning (DRL) as quantum-inspired Q-learning, quantum-inspired state–action–reward-state–action, quantum-inspired deep Q-network, quantum-inspired policy gradient, quantum-inspired deep deterministic policy gradient, quantum-inspired twin-delayed deep deterministic policy gradient, quantum-inspired actor–critic, quantum-inspired proximal policy optimization, and quantum-inspired soft actor–critic. These proposed nine QDRL approaches are compared with DRL approaches under two 100% RESs. The numeric results show that the QDRL obtains more minor carbon emissions and frequency deviations under complex 100% RESs. Moreover, the quantum states of QDRL match the uncertain states of the prosumers of 100% RESs. Besides, the exploration and exploitation of the QDRL for the real-time control problems of multi-agent systems are verified and analyzed.
更多查看译文
关键词
Quantum technology,Deep reinforcement learning,Exploration and exploitation,Real-time distributed generation control,100% renewable energy systems
AI 理解论文
溯源树
样例
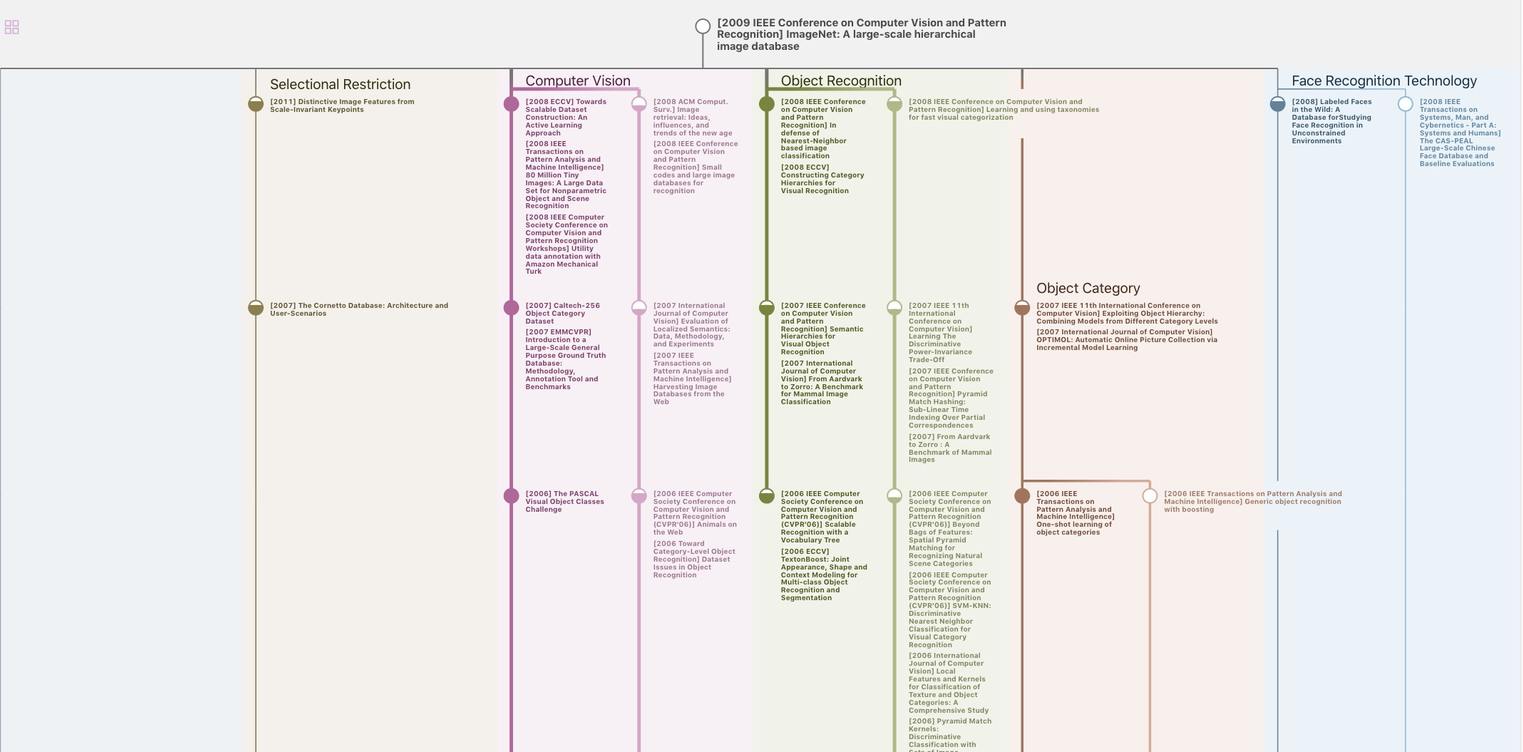
生成溯源树,研究论文发展脉络
Chat Paper
正在生成论文摘要