A machine learning driven multiple criteria decision analysis using LS-SVM feature elimination: Sustainability performance assessment with incomplete data
Engineering Applications of Artificial Intelligence(2023)
摘要
The move towards an era of big data structured problems has led to the development of state-of-the-art solutions, mainly in decision sciences. Large Scale Decision-Making (LSDM) and Data-Driven Decision-Making (DDDM) approaches have emerged from a rapidly evolving research field. A common challenge in LSDM problems formed from various heterogeneous databases is incomplete data. Multiple criteria decision analysis approaches have been utilized as the primary element of LSDM techniques to solve similar complex problems, and these methods cannot function under incomplete data. Large number of dimensions in LSDM problems are usually problematic, which has previously been addressed by machine learning driven methods. Moreover, while in traditional multiple criteria decision analysis methods, the criteria are based on beneficial and non-beneficial attributes, in LSDM problems, decision-makers define specific objectives for attributes adjusting the problem with their specific preferences. This study proposed a machine learning-driven DDDM method for solving LSDM problems with incomplete data and a substantial number of decision attributes. Incomplete data imputation was conducted using the Expectation–Maximization (EM) algorithm, and Recursive Feature Elimination (RFE) with Least Square Support Vector (LS-SVM) was used to extract the core criteria. Target-based COmbined COmpromise Solution (T-CoCoSo) and Target-based Multi-Attributive Border Approximation Area Comparison (T-MABAC) methods combined with Shannon’s Entropy weighting method were used for the performance assessment stage. Ultimately, to validate the reliability of the proposed method, sustainability performance of all countries worldwide under Sustainable Development Goals (SDGs) with incomplete data was evaluated. The outcomes show that the proposed machine learning-driven data-driven decision-making method is a reliable tool for such LSDM problems.
更多查看译文
关键词
Incomplete data imputation,Expectation–Maximization,Recursive feature elimination,Least square support vector machine,Target-based COmbined COmpromise SOlution,Target-based Multi-Attributive Border Approximation Area Comparison,Sustainable development goals
AI 理解论文
溯源树
样例
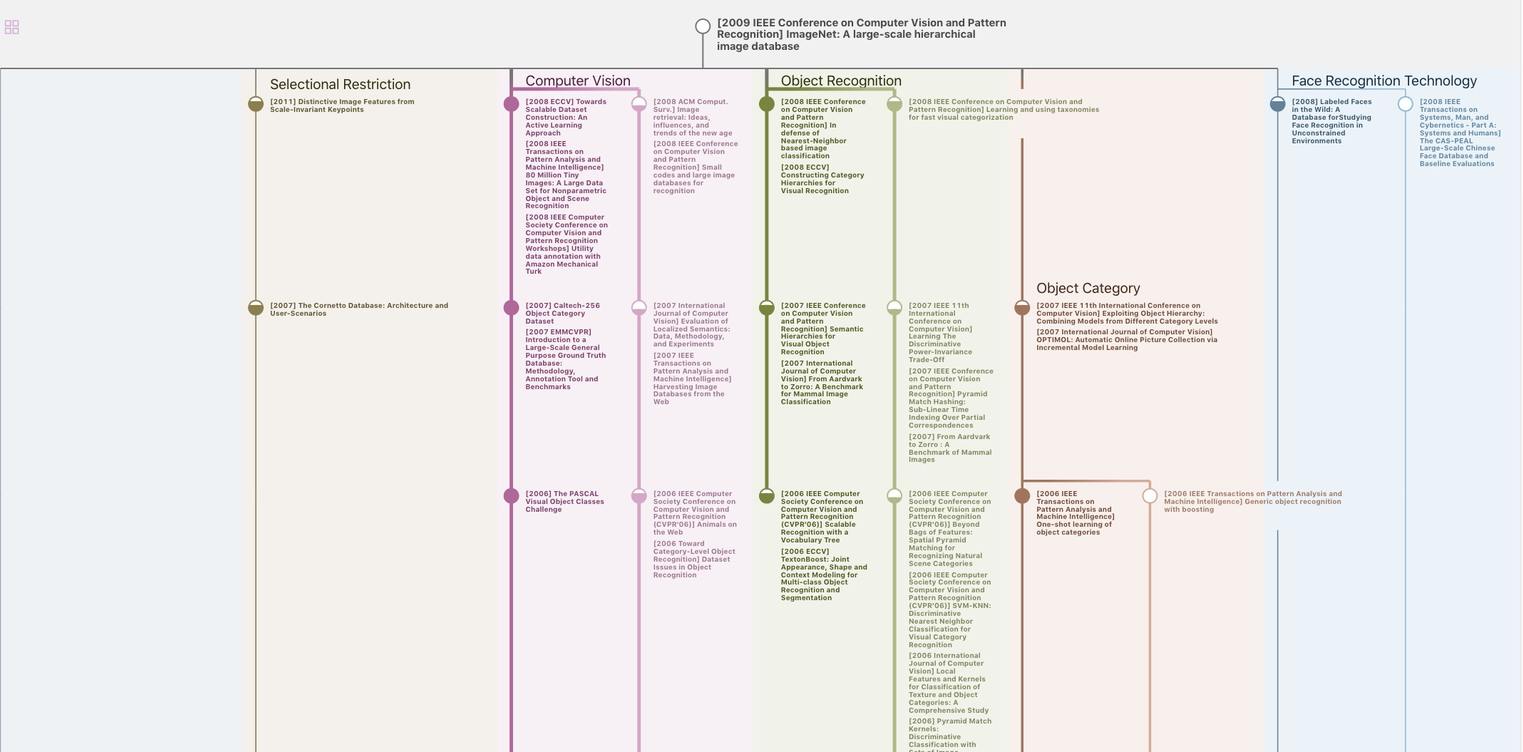
生成溯源树,研究论文发展脉络
Chat Paper
正在生成论文摘要