UWB Positioning System Based on LSTM Classification With Mitigated NLOS Effects
IEEE Internet of Things Journal(2023)
摘要
It is known that an ultrawideband (UWB)-based indoor positioning system (IPS) has superior positioning performance and can meet the requirements of location-based services (LBSs) as the Internet of Things (IoT) applications. However, there is a limitation of UWB positioning when it is conducted at the nonline-of-sight (NLOS) channels degrading the UWB ranging accuracy at indoor environments. In this article, we propose an artificial intelligence (AI) applied UWB positioning system that can enhance the positioning performance by classifying channel conditions with channel impulse response (CIR) of the received UWB signal. The proposed system mitigates the positioning degradation caused by the NLOS situations by performing extended Kalman filter (EKF) localization and long short-term memory (LSTM) training of the observed channel status. The main feature of the proposed UWB positioning method is that it can be used even at unknown locations not trained with the LSTM model learning the channel status, because of our training strategy of not the position coordinates, but the UWB ranging error between UWB devices corresponding to CIR of the received UWB signal. This article provides the experimental setup and performance evaluation results of the proposed system. The evaluation results showed that the proposed AI-applied UWB positioning method significantly improved its accuracy performance compared with the existing positioning methods.
更多查看译文
关键词
Deep learning (DL),extended Kalman filter (EKF),indoor positioning system (IPS),location-based service (LBS),long short-term memory (LSTM),ultrawideband (UWB)
AI 理解论文
溯源树
样例
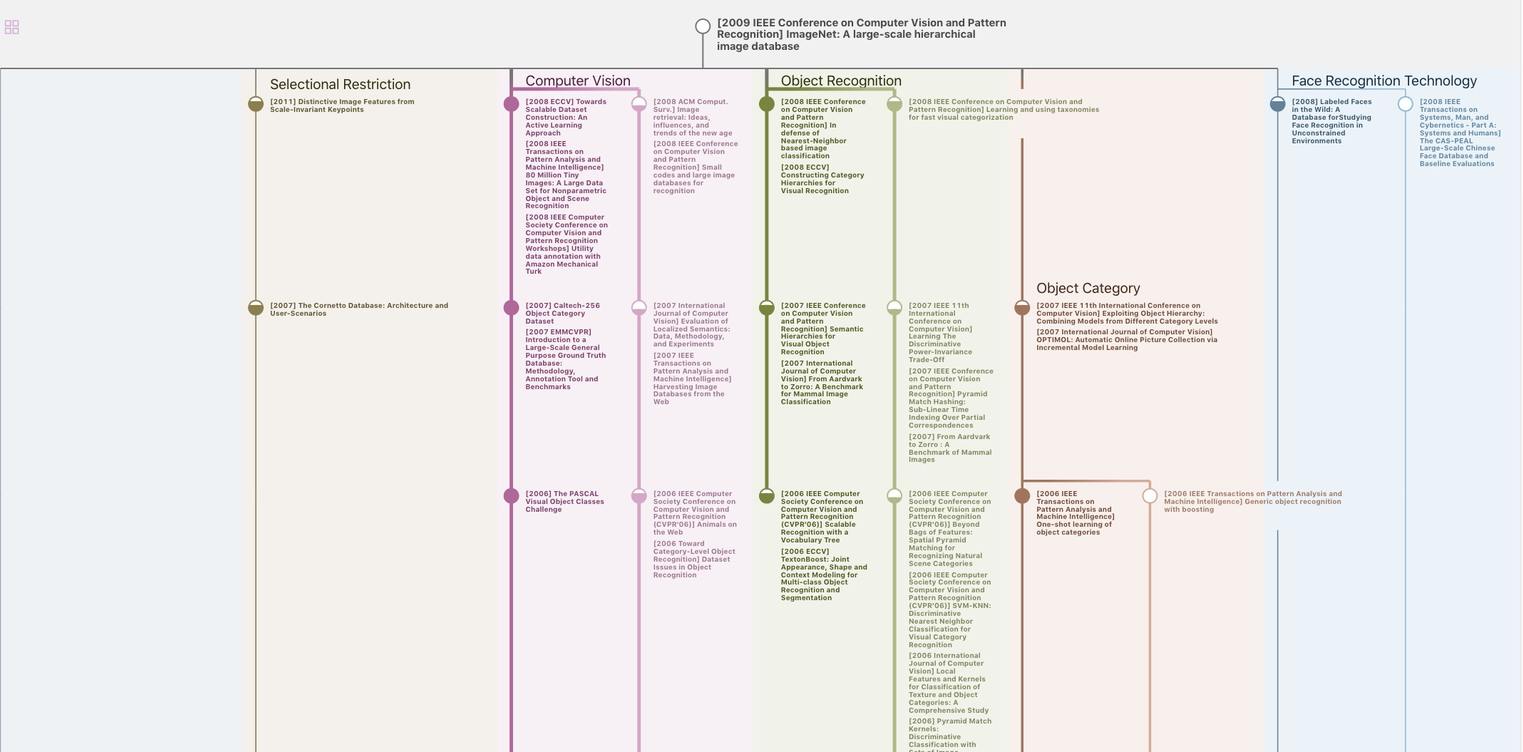
生成溯源树,研究论文发展脉络
Chat Paper
正在生成论文摘要