Tool Wear Prediction in the End Milling Process of Ti-6Al-4V using Bayesian Learning
2022 International Conference on Advanced Mechatronic Systems (ICAMechS)(2022)
摘要
Titanium alloy is one of the most popularly used materials in various industries because of its desirable mechanical properties. However, it is also a difficult-to-cut material because of its low specific heat and thermal conductivity. This influences an end milling process of titanium alloy through tool wear that affects not only product quality but also productivity. Therefore, accurate tool wear prediction is required to improve titanium alloy quality and replace the tool at an appropriate time. Furthermore, since the effects of tool wear prediction on the overall machining process are significant in terms of cost and time, the uncertainty of tool wear prediction should be taken into account. In this work, a deep learning-based uncertainty-aware tool wear prediction model using Bayesian learning approach is proposed. The proposed probabilistic model could produce a predictive distribution over estimated tool wear with uncertainty awareness. Experiments with real-world end milling processes have proven the effectiveness of the proposed method. The results indicate that the proposed method outperforms existing comparative tool wear prediction models.
更多查看译文
关键词
tool wear prediction,Bayesian learning,deep learning,machining process
AI 理解论文
溯源树
样例
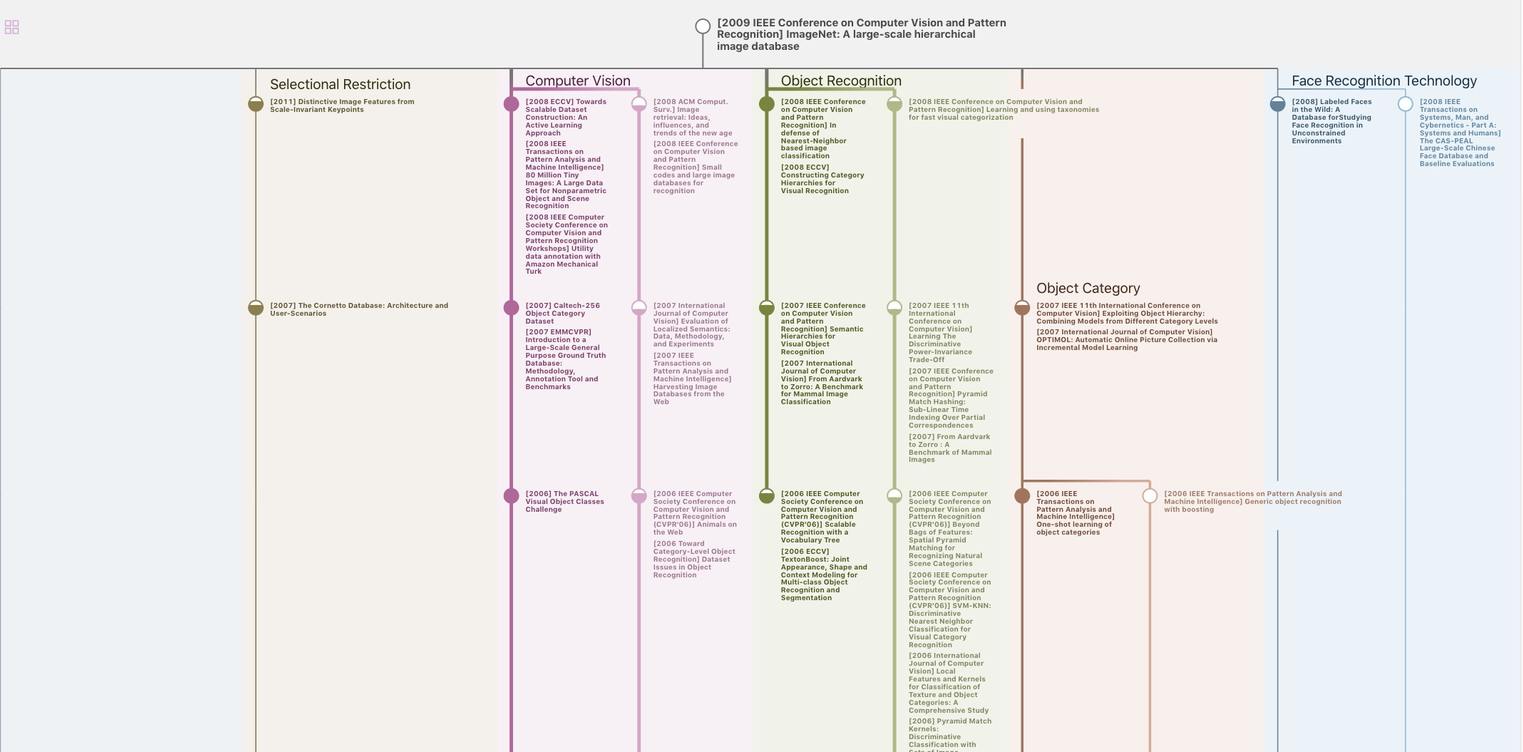
生成溯源树,研究论文发展脉络
Chat Paper
正在生成论文摘要