Majority re-sampling via sub-class clustering for imbalanced datasets
Journal of Experimental & Theoretical Artificial Intelligence(2023)
摘要
Many real world domain problem datasets are class imbalanced where the number of data in a given class is much less than in the other classes. In related literatures, under- and over-sampling techniques are widely used techniques to re-balance the class imbalanced datasets. However, their limitations include the risk of removing representative majority class data samples and the overfitting problem because of generating a large number of synthetic minority class data samples. Therefore, a novel approach, namely Majority Re-sampling visa Sub-class Clustering (MRSC) is introduced. It uses a clustering algorithm to group the majority class data into several clusters, i.e. sub-classes. Then, a new training set containing multiple sub-classes and a minority class is produced, after which the classifier is trained using this new multi-class dataset which has a lower imbalance ratio than the original dataset. The experimental results obtained using 44 two-class imbalanced datasets show that MRSC combined with the k-NN classifiers, including single and ensemble classifiers, significantly outperforms the other classifiers as well as seven state-of-the-art re-sampling approaches. Moreover, for the clustering algorithms based on affinity propagation and k-means, very similar results can be produced, without significant differences in performance, which indicate the stability of MRSC.
更多查看译文
关键词
Clustering,data mining,imbalanced datasets,machine learning,under-sampling
AI 理解论文
溯源树
样例
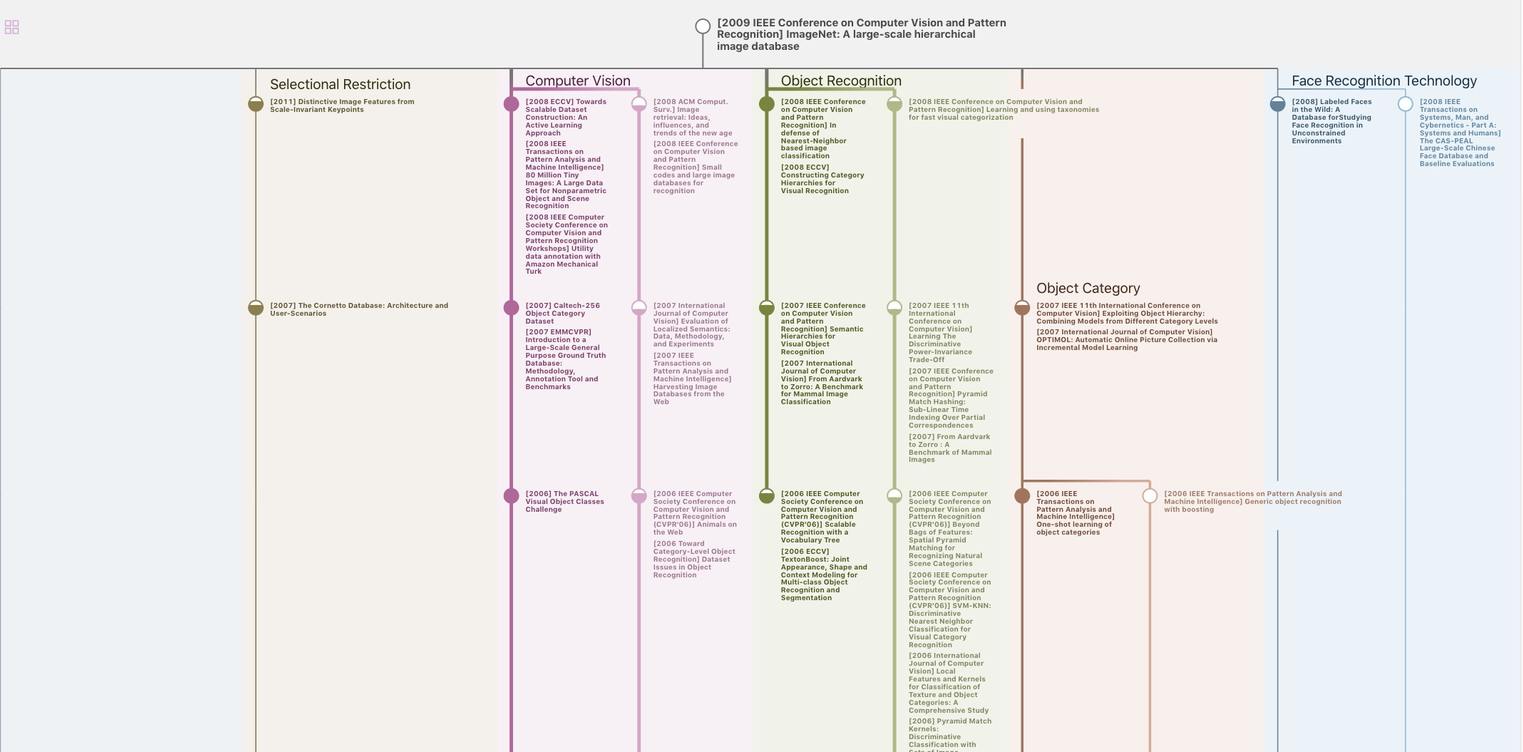
生成溯源树,研究论文发展脉络
Chat Paper
正在生成论文摘要