An overview of non-Gaussian state-space models for wind speed data
Energy(2023)
摘要
Over the years, several studies have investigated the stochastic phenomenon that generates wind energy. Many studies have focused on descriptive analyses to verify the most appropriate probabilistic distribution of this variable, while have examined the mathematical transformations in order to meet specific modelling requirements of well-known time series models. The wind variable is characterized by asymmetric behaviour and the usual time series models can result in poor predictive behaviour. This work presents a flexible class of time series models that account for asymmetry while being analytically and iteratively solved for inference and prediction, overcoming the need for mathematical transformations. The models are defined in the non-Gaussian state-space class, where asymmetric distributions are assumed for the phenomenon of interest. Therefore, several continuous probabilistic models were evaluated to model this variable, in addition to the more consolidated ones known as the Weibull and Gamma distributions. The results are promising for flexible long-term forecasts, generalizing the distributions consolidated in the literature on wind speed modelling.
更多查看译文
关键词
Bayesian inference,Wind power,State space model,Wind speed,Bayesian forecasting
AI 理解论文
溯源树
样例
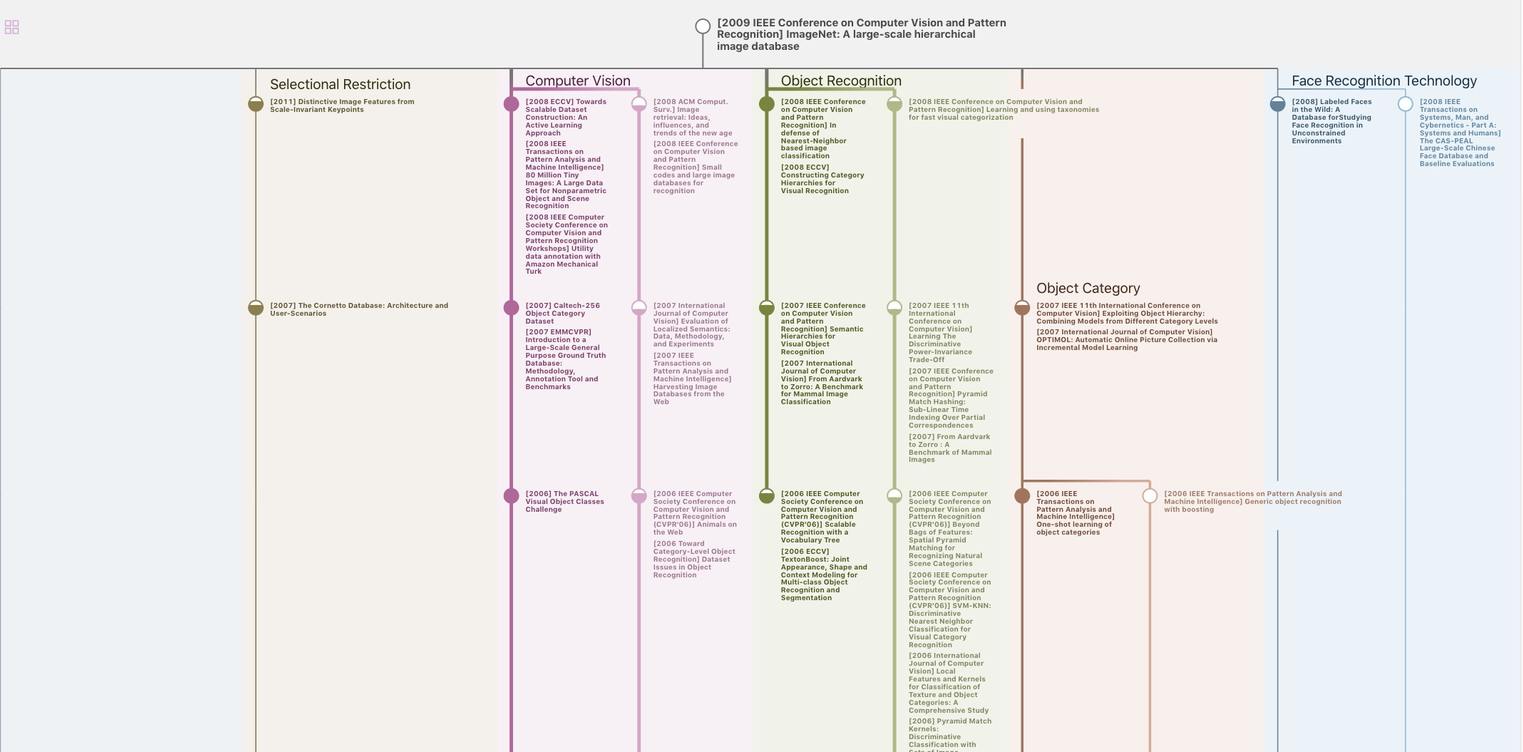
生成溯源树,研究论文发展脉络
Chat Paper
正在生成论文摘要