Differential attention net: Multi-directed differential attention based hybrid deep learning model for solar power forecasting
Energy(2023)
摘要
Solar power plants provide a clean alternative to conventional thermal power plants. However, solar plant dependency on environmental factors threatens existing energy grids in terms of power quality and management. So, accurate solar power prediction is indispensable for designing and managing solar power plants. This work introduces a novel multi-directed differential attention-based differential attention net for accurate solar power prediction. The proposed model in the first step utilizes the feature of convolution neural network (CNN) and bidirectional long-short memory (BiLSTM) based hybrid deep learning model in parallel branches to extract the local dependencies in the latent space. However, long-term temporal dependencies in this time-series problem deteriorate prediction performance. So, an attention-based deep learning model is applied in the parallel branches to tap the long-term dependencies. Moreover, Bayesian optimization is used to fine-tune the hyperparameter of the proposed model, as it provides better results with the gaussian backend process. The proposed model is tested on two different datasets, one is real-time solar plant dataset located in the Australian desert, and other one is from Delhi, India. The proposed net is compared with other hybrid deep learning models (e.g., comprising of the recurrent neural network, gated recurrent unit, and LSTM). And the findings of the work confirms the effectiveness of the proposed model.
更多查看译文
关键词
Solar photovoltaic power,Short-term prediction,Deep learning,Attention net,Bayesian optimization
AI 理解论文
溯源树
样例
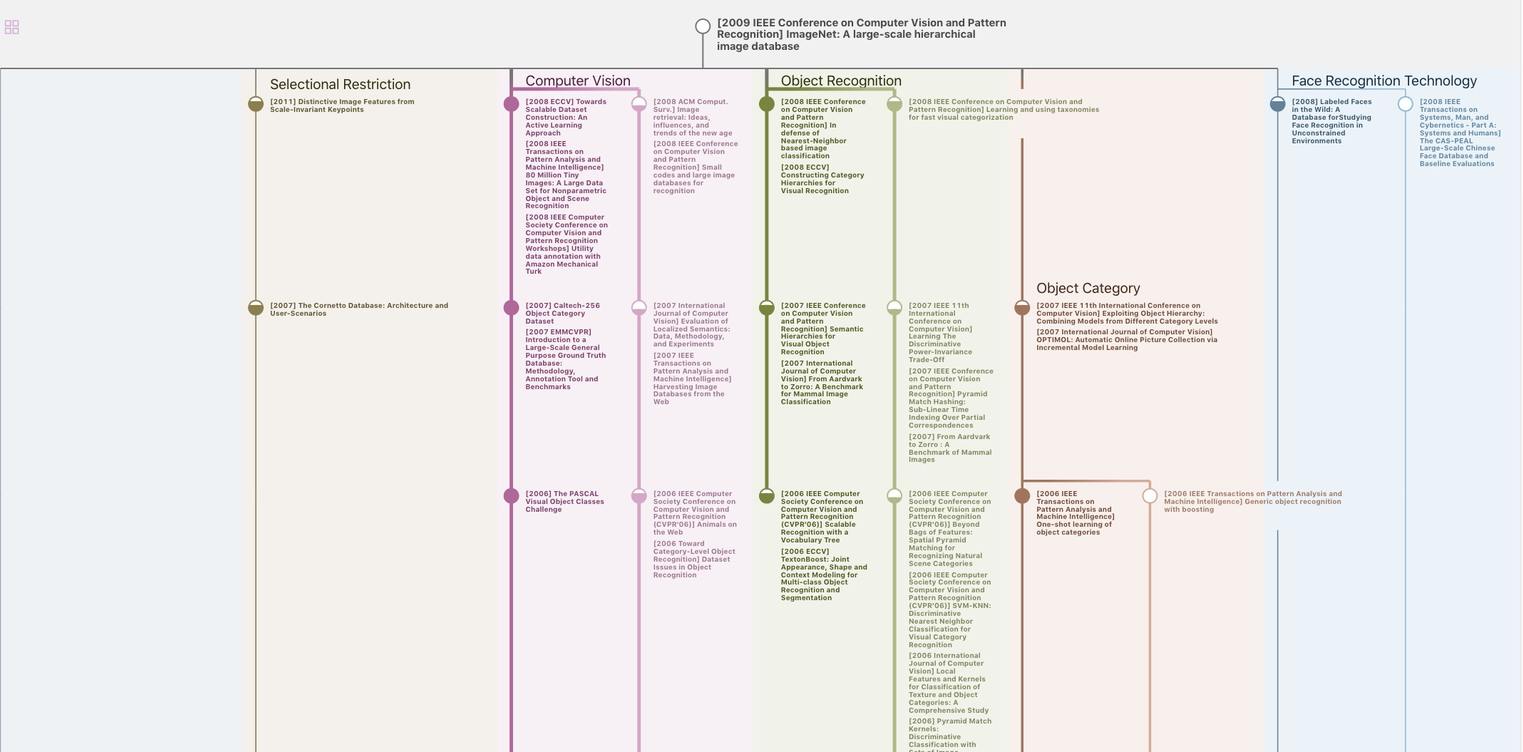
生成溯源树,研究论文发展脉络
Chat Paper
正在生成论文摘要