Graph-based detection for false data injection attacks in power grid
Energy(2023)
摘要
False data injection attack (FDIA) is the main network attack type threatening power system. FDIA affect the accuracy of data by modifying the measured values of measuring equipment. When the power grid topology has not changed, the data-driven detection method has high detection accuracy. However, the power grid topology changes when faults, maintenance or adjustment in power flow distribution occur in the power system. The data-driven detection method can not capture the spatial feature changes, and the detection accuracy decreases. Considering the changes of power grid topology, a false data detection method based on graph neural network is proposed in this paper, which extracts the spatial features of power grid topology information and operation data through gated graph neural network (GGNN). To enhance node representation in this method, the attention mechanism is adopted to assign aggregation weights to neighbor nodes. This method is tested on IEEE 14-bus and IEEE 118-bus systems. The experimental simulation results show that compared with other data-driven detection methods, the proposed method can significantly improve the detection accuracy under the changes of power grid topology.
更多查看译文
关键词
Attention mechanism,False data injection attacks detection,Graph neural networks,Power system security,State estimation
AI 理解论文
溯源树
样例
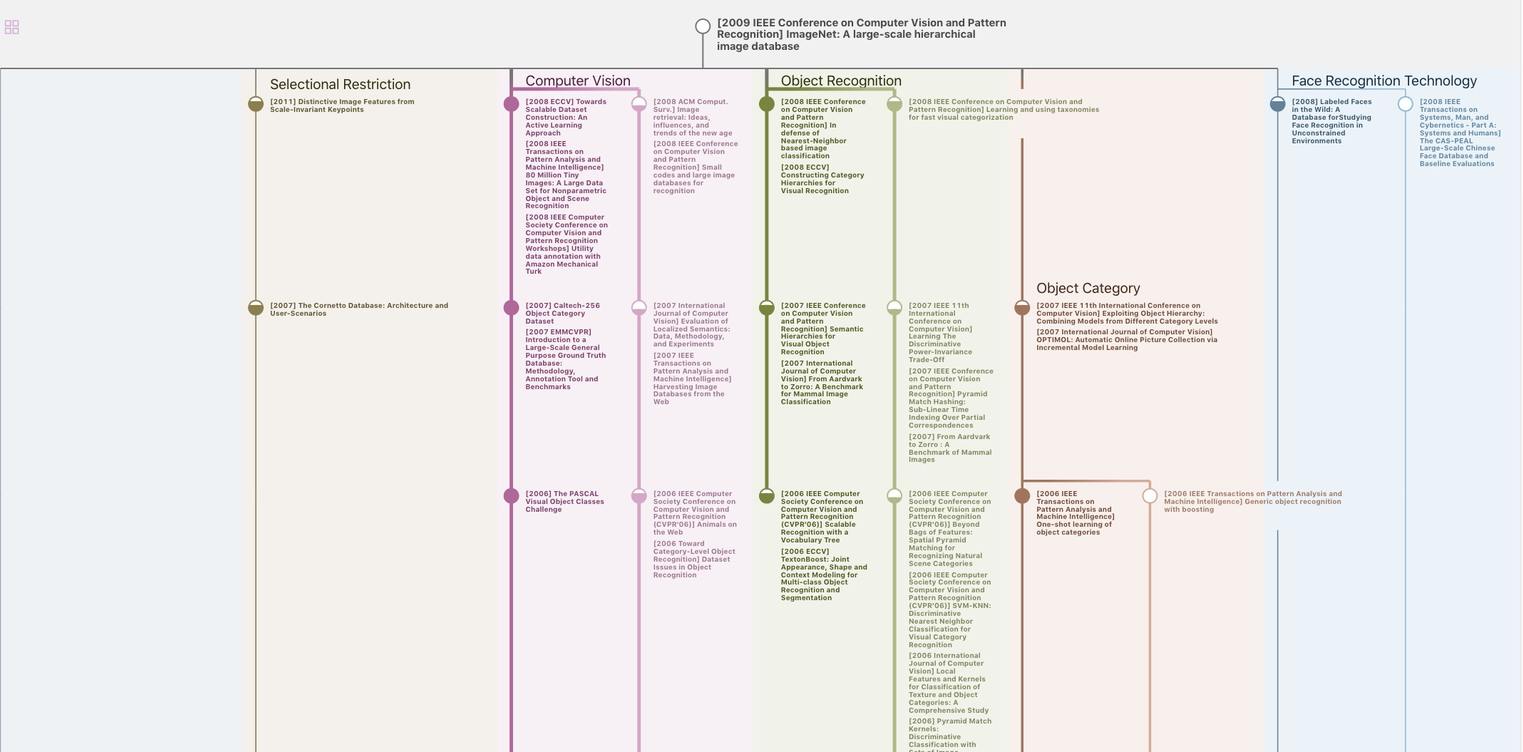
生成溯源树,研究论文发展脉络
Chat Paper
正在生成论文摘要