Deep learning for Ground Penetration Radar Reflection Images in Civil Structures Investigation
2022 IEEE International Conference on Aerospace Electronics and Remote Sensing Technology (ICARES)(2022)
摘要
Ground Penetrating Radar (GPR) is one of the NDT (Non-Destructive Techniques) in the geophysics field. Most Geoscientists accept the instrument's capability to conduct near-surface mapping. On the other side, the technology in deep learning vastly developed in many sectors, affecting the perspective of radar-images post-processing. The problem occurred when a lot of identical objects were detected in the GPR section. Hence, the interpreter will face difficulties when performing manual object detection on a large scale of the dataset. In this study, the deep learning algorithm attempted to be employed to forage the civil structures and deal with overtired work interpretations. This study specifies five structures from the dataset: Pile, Pipe, Powerline, Rebar, and Void/Collapse Structure. All objects are confirmed buried in the subsurface when field measurement is conducted. This study introduces a new approach to improving accuracy called IC-CNN (Integrated Contouring in Convolutional Neural Network). The IC-CNN method is expected to become an advanced technique to achieve solid identifications for GPR data through an object contour and object localization. The B-Scan of GPR Images was employed for the analysis. However, the primary processing of GPR data has been conducted to make it adequate as relevant input. As a result, it presented a correlation with a 95% confidence level. Furthermore, IC-CNN gave significance
$\pm 3.5$
% rather than CNN for the GPR B-scan data, which was concluded after 2,500 iterations. In final, the IC-CNN is promising as long as it is well-processed.
更多查看译文
关键词
Ground Penetrating Radar,Convolutional Neural Network,Object Contour,Wiggle,Civil Structures
AI 理解论文
溯源树
样例
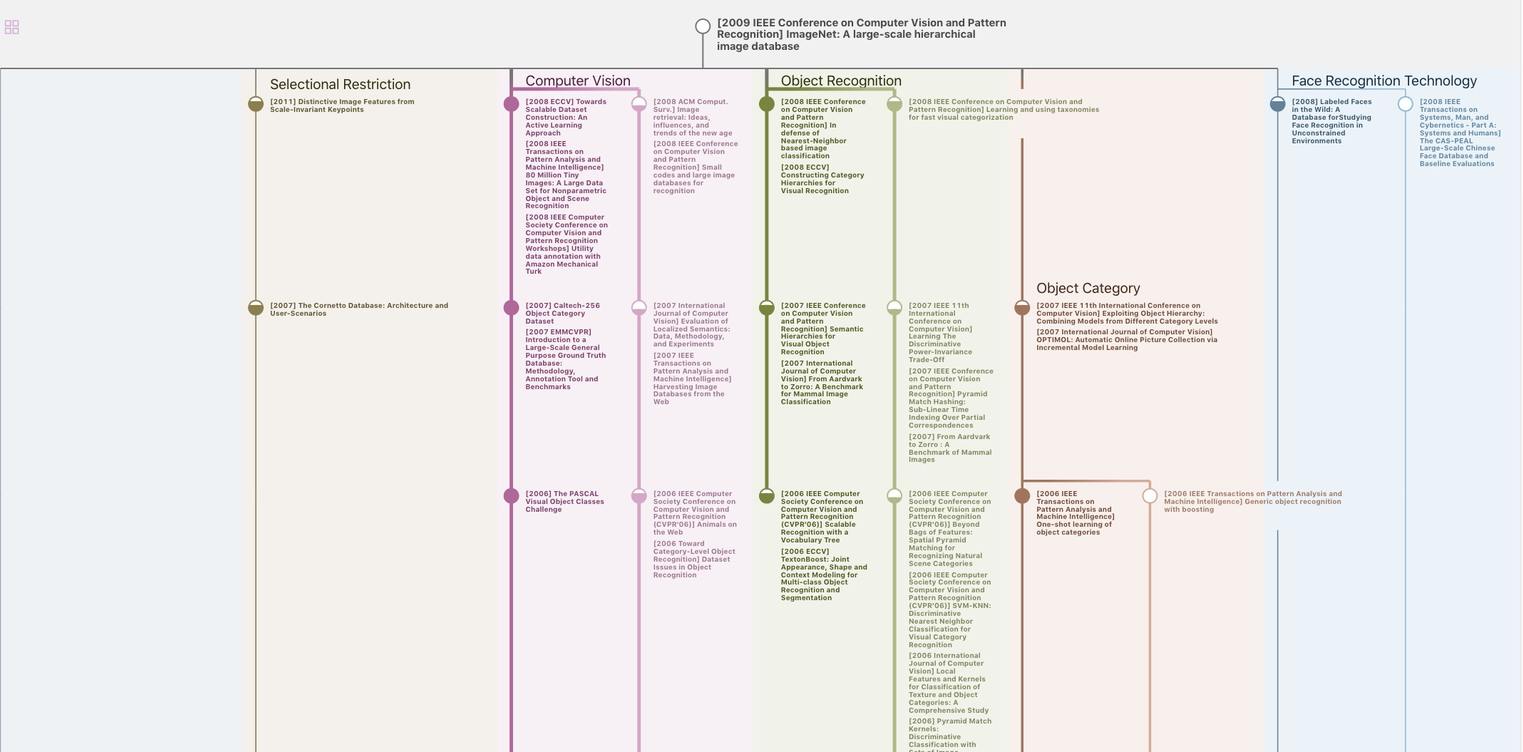
生成溯源树,研究论文发展脉络
Chat Paper
正在生成论文摘要