Unsupervised Heterogeneous Cryo-EM Projection Image Classification Using Autoencoder
2022 IEEE International Conference on Bioinformatics and Biomedicine (BIBM)(2022)
摘要
Heterogeneous three-dimensional (3D) reconstruction in single-particle cryo-electron microscopy (cryo-EM) is a significant but very challenging technique for recovering conformational heterogeneity of proteins or other biological macromolecules and their complexes in different functional states. Heterogeneous projection image classification is an effective way for solving the heterogeneity problem in single-particle cryo-EM. Most existing heterogeneous projection image classification methods are based on supervised learning or require a large amount of a priori knowledge, such as the common lines or orientations of the projection images, which has many limitations in practical applications. In this paper, we propose an unsupervised heterogeneous cryo-EM projection image classification algorithm based on autoencoders that only needs to know the number of heterogeneous conformations in the dataset and does not require any labeling information of the projection images as well as other prior knowledge. We implement a simple autoencoder with a multi-layer perceptron that is trained in iterative mode and a complex autoencoder with a residual network that is trained in one-pass learning mode to convert heterogeneous projection images into latent variables. The extracted high-dimensional features are reduced to two dimensions by the uniform manifold approximate and projection dimensionality reduction algorithm and then cluster them using the spectral clustering algorithm. The proposed algorithm is applied to two heterogeneous cryo-EM datasets to demonstrate its classification performance. Experimental results show that the proposed algorithm can effectively extract category features of heterogeneous projection images and can classify them with high accuracy.
更多查看译文
关键词
cryo-electron microscopy,single-particle reconstruction,structural heterogeneity,autoencoder,unsupervised classification
AI 理解论文
溯源树
样例
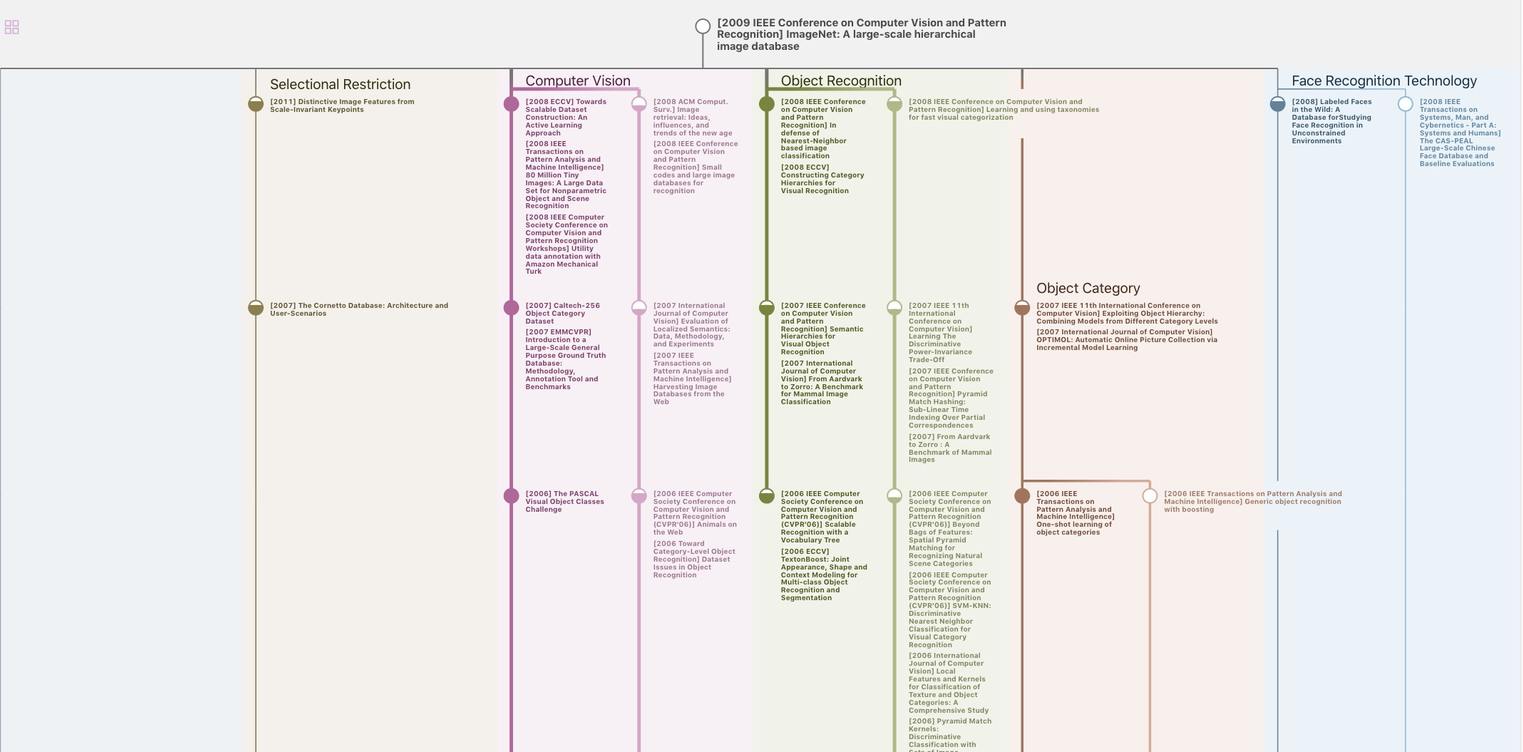
生成溯源树,研究论文发展脉络
Chat Paper
正在生成论文摘要