MHSnet: Multi-head and Spatial Attention Network with False-Positive Reduction for Lung Nodule Detection
2022 IEEE International Conference on Bioinformatics and Biomedicine (BIBM)(2022)
摘要
Mortality from lung cancer has ranked high among cancers for many years. Early detection of lung cancer is critical for disease prevention, cure, and mortality rate reduction. Many existing detection methods on lung nodules can achieve high sensitivity but meanwhile introduce an excessive number of false-positive proposals, which is clinically unpractical. In this paper, we propose the multi-head detection and spatial attention network, shortly MHSnet, to address this crucial false-positive issue. Specifically, we first introduce multi-head detectors and skip connections to capture multi-scale features so as to customize for the variety of nodules in sizes, shapes, and types. Then, inspired by how experienced clinicians screen CT images, we implemented a spatial attention module to enable the network to focus on different regions, which can successfully distinguish nodules from noisy tissues. Finally, we designed a lightweight but effective false-positive reduction module to cut down the number of false-positive proposals, without any constraints on the front network. Compared with the state-of-the-art models, our extensive experimental results show the superiority of this MHSnet not only in the average FROC but also in the false discovery rate (2.64% improvement for the average FROC, 6.39% decrease for the false discovery rate). The false-positive reduction module takes a further step to decrease the false discovery rate by 14.29%, indicating its very promising utility of reducing distracted proposals for the downstream tasks relied on detection results.
更多查看译文
关键词
Lung nodule detection,Spatial attention mechanism,False-positive reduction
AI 理解论文
溯源树
样例
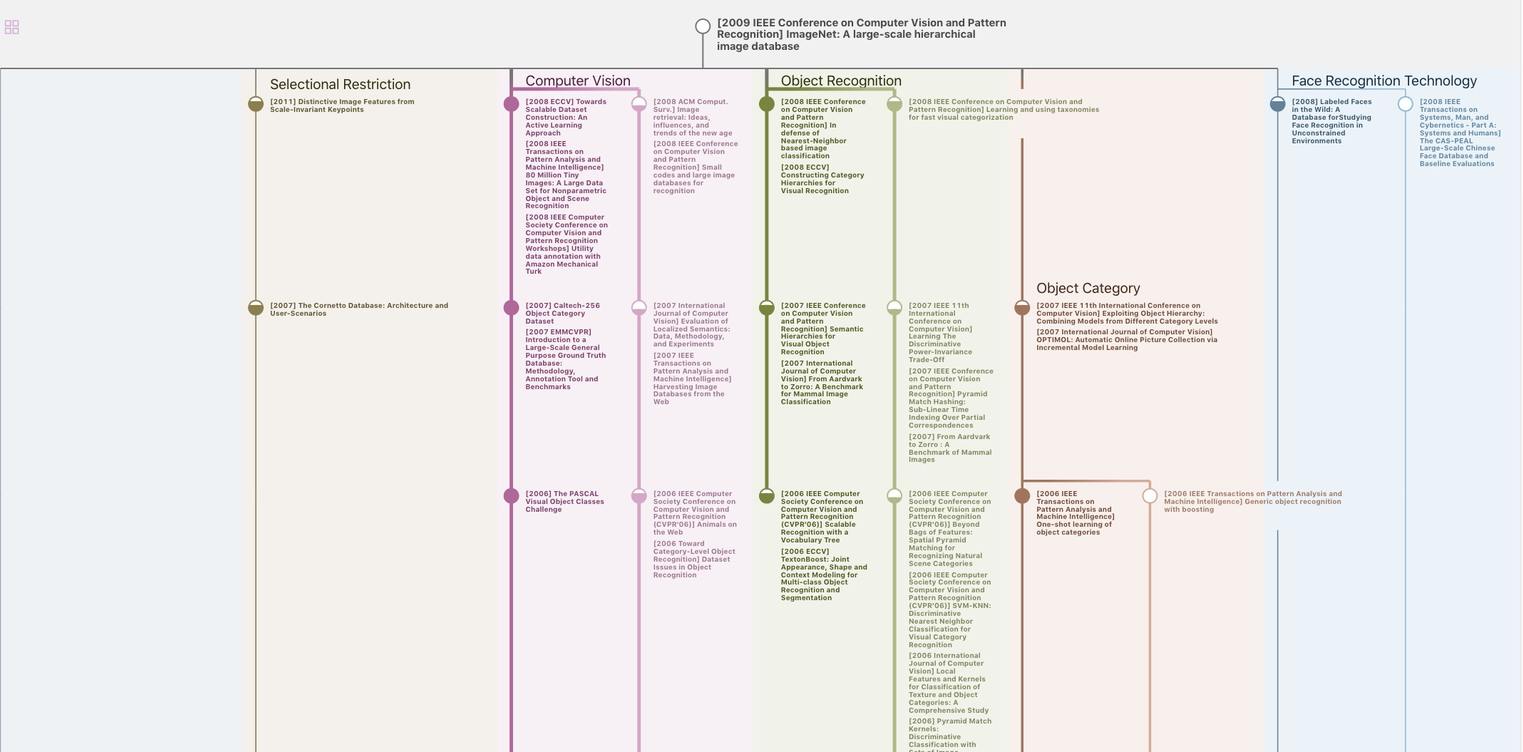
生成溯源树,研究论文发展脉络
Chat Paper
正在生成论文摘要