Quantum-enhanced Regression Analysis Using State-of-the-art QLSAs and QIPMs
2022 IEEE/ACM 7th Symposium on Edge Computing (SEC)(2022)
摘要
Quantum computing has the potential to speed up machine learning methods. One major direction is using quantum linear algebra to solve linear system problems or optimization problems in the machine learning area. Quantum approaches in the literature for different types of least squares problems demonstrate speedups w.r.t. dimension but have disadvantages w.r.t. precision and condition number. In this paper, we discuss how an iterative refinement scheme can deliver an accurate solution without the excessive cost of Quantum Linear System Algorithms (QLSAs). In addition, we propose an adaptive regularization approach that can mitigate the effect of condition number on solution time. In the second part of this paper, we investigate how state-of-the-art Quantum Interior Point Methods (QIPMs) can solve more sophisticated regression problems such as Lasso regression and support vector machine problems, which can be reformulated as quadratic optimization problems.
更多查看译文
关键词
Quantum Machine Learning,Quantum Interior Point Method,Quantum Linear System Algorithm,Least Squares Method,Support Vector Machine,Lasso Regression,Iterative Refinement
AI 理解论文
溯源树
样例
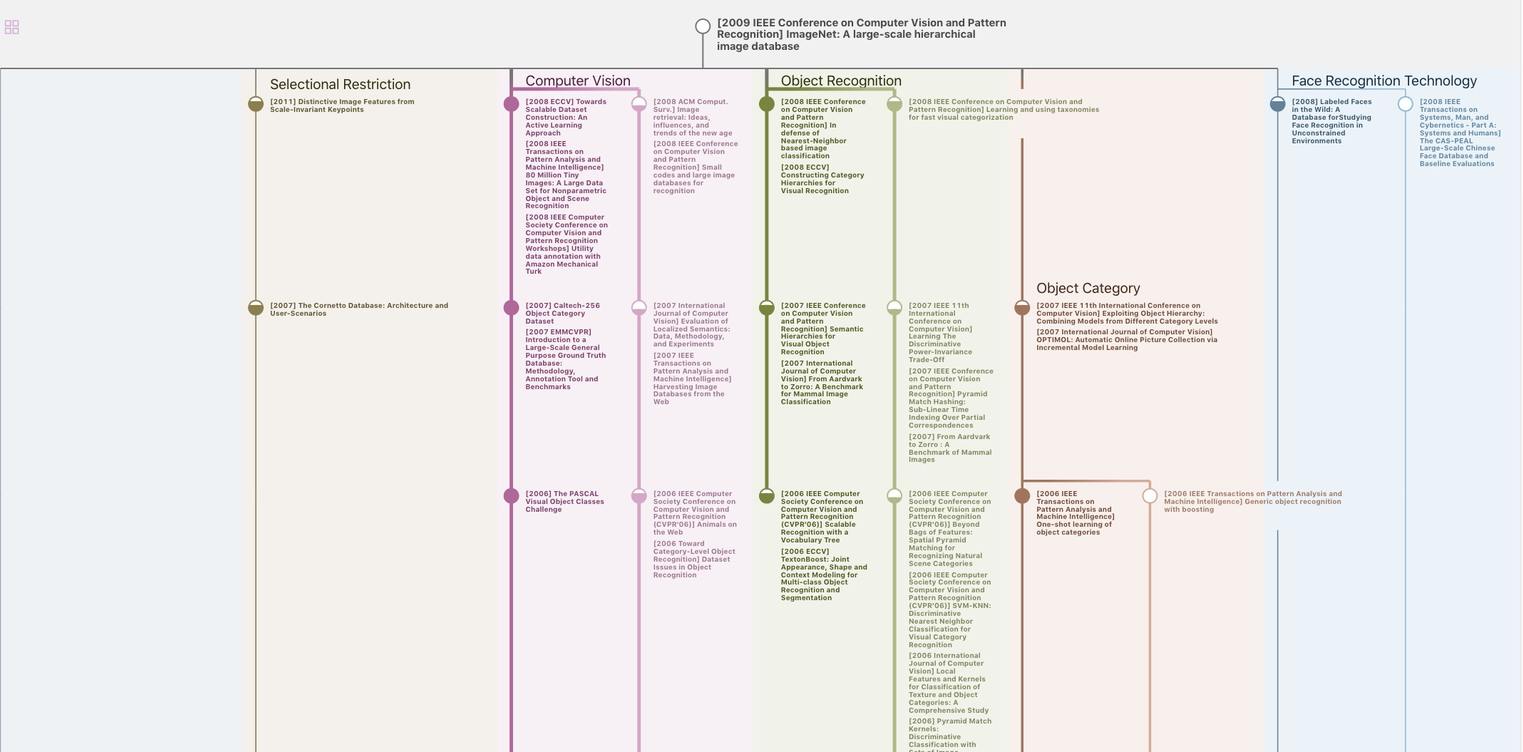
生成溯源树,研究论文发展脉络
Chat Paper
正在生成论文摘要