Learned image compression via multiscale prior for machine recognition
Journal of Electronic Imaging(2023)
摘要
The conventional image compression framework is pixel fidelity-driven, which can generate compressed images with considerable visual quality even at low bit rates. However, these methods emphasize the human visual experience and ignore the need for machine recognition-driven tasks. To this end, we propose an image compression framework that utilizes multiscale prior information extracted from the machine perceptual model to improve the machine recognition accuracy of compressed images. Specifically, the interaction refinement module (IRM) is designed to interact multiscale prior information with each other, adaptively retaining machine recognition-relevant features to enhance its expression on compact features. To further improve the accuracy of machine recognition, machine vision perceptual loss is designed on semantic variation weight, which is the weight of semantic variation degree of deep adjacent layers in multiscale priors. Machine vision perceptual loss is used to optimize the semantic distortion of compressed images for retaining important semantic information. Experimental results show that compared with compression methods including BPG, WebP, Mentzer, NIC, IUWD, and RCIS, the Top-1 recognition accuracy of the proposed method is improved by 10.9%, 19%, 11.6%, 12.9%, 6%, and 2.7% at a lower bit rate (0.2 bpp). In addition, the performance improvement on other machine recognition networks and machine vision tasks shows the versatility of the proposed method.
更多查看译文
关键词
image compression,multiscale,recognition
AI 理解论文
溯源树
样例
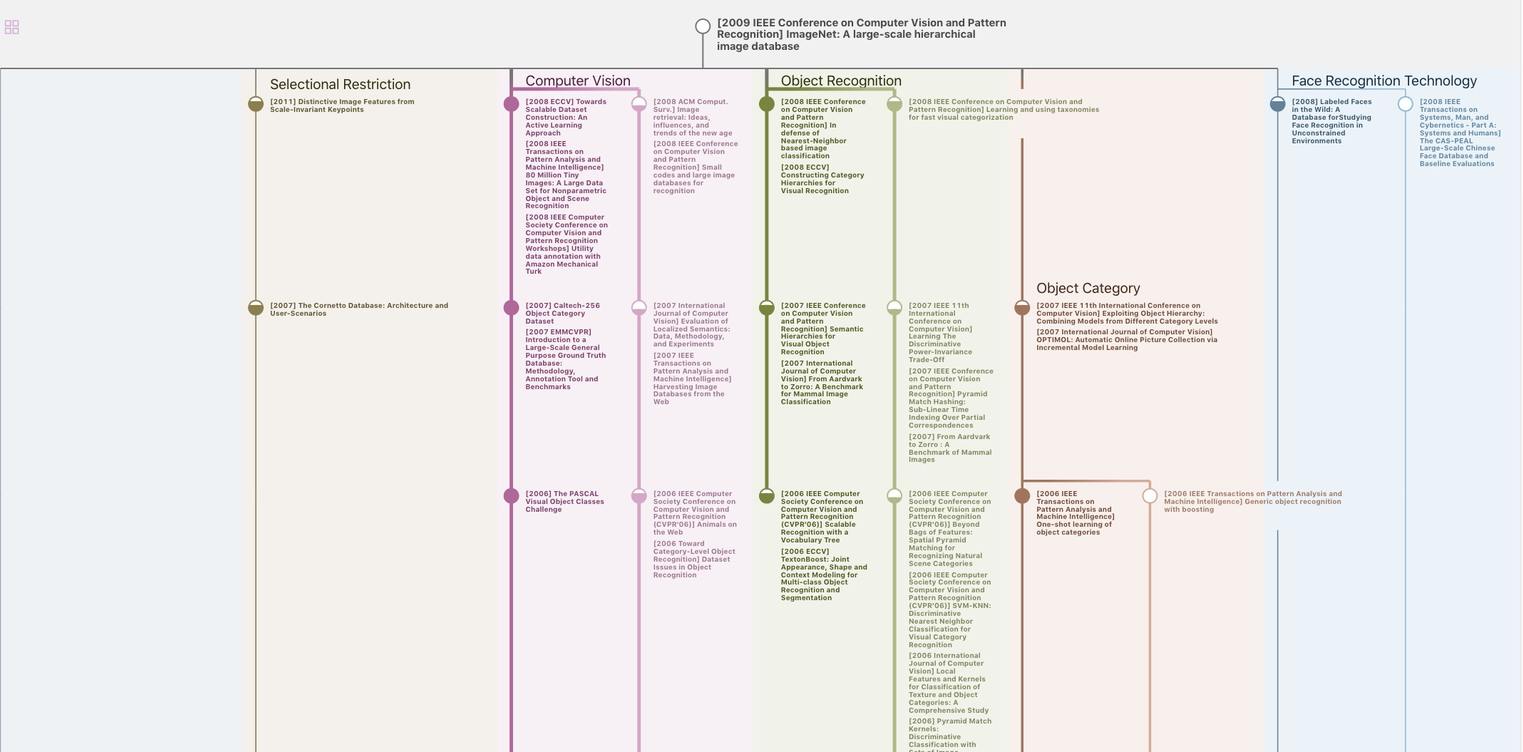
生成溯源树,研究论文发展脉络
Chat Paper
正在生成论文摘要