Master-CAM: Multi-scale fusion guided by Master map for high-quality class activation maps
Displays(2022)
摘要
Class Activation Map (CAM) is one of the most popular approaches to visually explain the convolutional neural networks (CNNs). To obtain fine-grained saliency maps, some works fuse saliency signals of the same image at larger scales. However, existing methods based on multi-scale fusion cannot effectively remove the noise from larger-scale images. In this paper, we propose Master-CAM, which uses Master map to guide multi-scale fusion process to obtain a high-quality class activation map. Master-CAM utilizes the general localization ability of the Master map to reduce the noise of the maps. We call the one with the general localization ability among the saliency maps from the same image as Master map, which is the saliency map of the original-scale input in the multi-scale scenario. In addition, we also present a simple yet effective fusion strategy, Master-Fusion, which is derived from the fusion operation in Master-CAM. Master-Fusion strategy can be easily attached to some saliency methods to improve the performance of these methods. We show through qualitative and quantitative experiments that the proposed Master-CAM outperforms the state-of-the-art methods in different CNN frameworks and datasets.
更多查看译文
关键词
Class activation maps,Saliency maps,Visual explanations,Visualizations
AI 理解论文
溯源树
样例
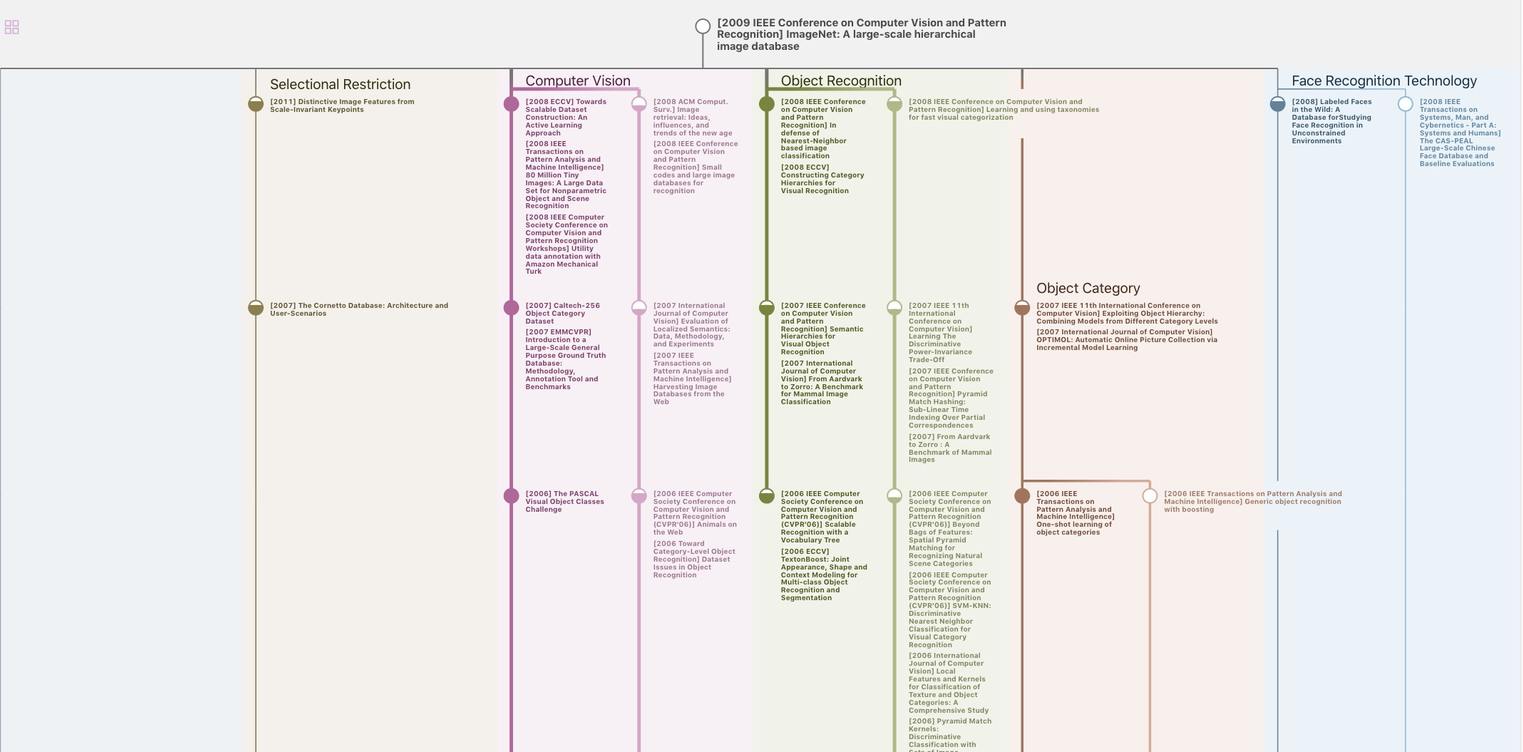
生成溯源树,研究论文发展脉络
Chat Paper
正在生成论文摘要