Deep Learning-based Point Cloud Joint Geometry and Color Coding: Designing a Perceptually-Driven Differentiable Training Distortion Metric
2022 IEEE Eighth International Conference on Multimedia Big Data (BigMM)(2022)
摘要
Deep learning (DL)-based coding has recently become very popular for multimedia data, notably images and point clouds (PCs). Training a DL coding model using the backpropagation algorithm requires a differentiable loss function. Thus, for PC joint geometry and color coding, both the PC geometry and color distortion metrics must be differentiable. Since the distortion/quality metrics commonly used for the final PC quality assessment do not meet this criterion, new PC distortion metrics have to be designed for DL-based training purposes. Moreover, for PC joint geometry and color coding, it is critical to define the balance between the geometry and color distortions in a meaningful way, ideally driven by the human perception and subjective quality assessment. In this context, this paper proposes a perceptually-driven design for a differentiable PC joint geometry and color distortion metric to be used for training purposes in DL-based coding, notably to define the relative weights for the geometry and color distortions. The obtained perceptually-driven weights achieve a rate reduction of around 3 % regarding the default balanced weights at no complexity cost. This is the first proposal in the literature with this purpose and this perceptual approach.
更多查看译文
关键词
point cloud,learning-based coding,distortion metric,perceptually-driven
AI 理解论文
溯源树
样例
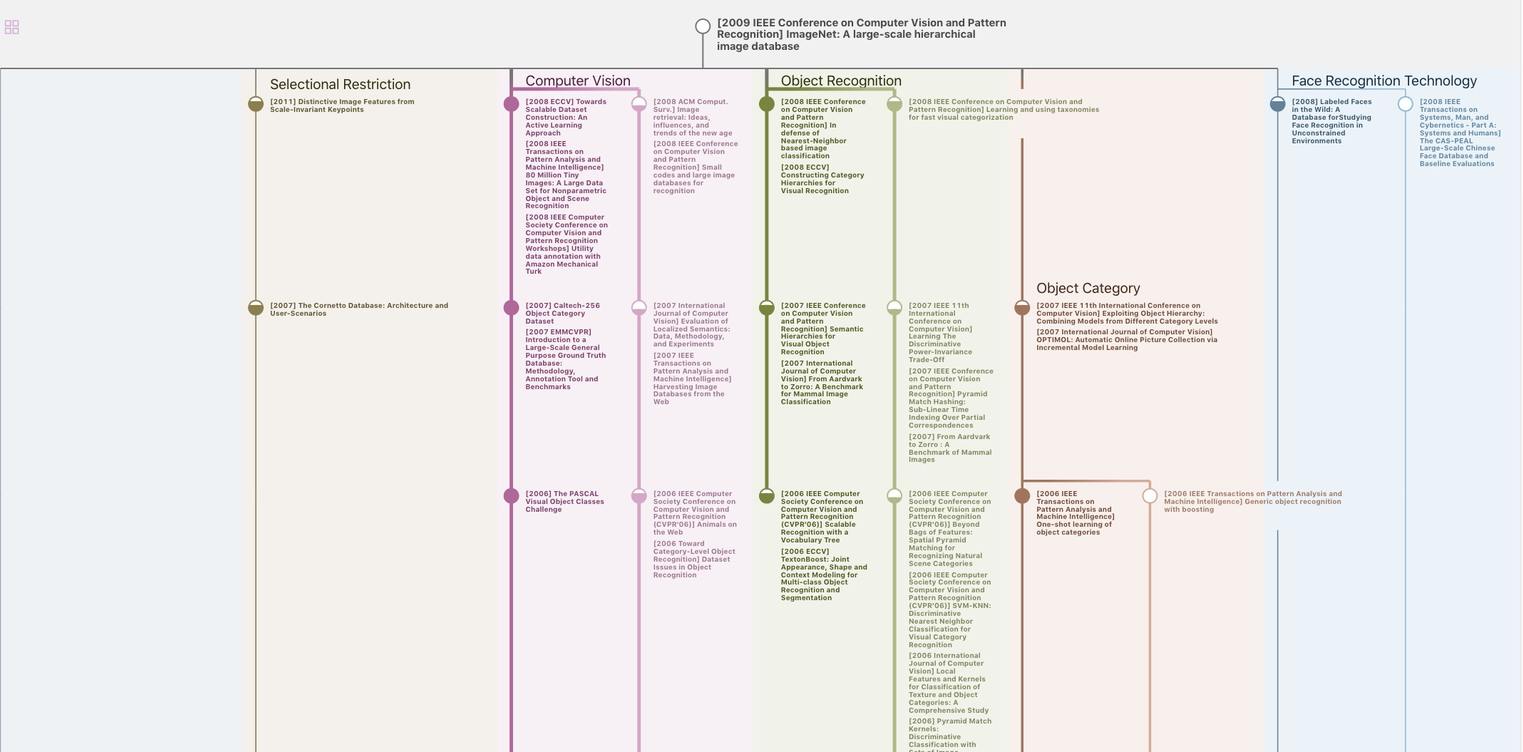
生成溯源树,研究论文发展脉络
Chat Paper
正在生成论文摘要