A 65nm 8-bit All-Digital Stochastic-Compute-In-Memory Deep Learning Processor
2022 IEEE Asian Solid-State Circuits Conference (A-SSCC)(2022)
摘要
High compute density improves the data reuse and is the key to reducing off-chip memory access and achieving high energy efficiency in ML accelerators. Compute-in-Memory (CIM) promises high compute density but requires ADCs, DACs that add to the macro’s energy and area [1] [2] limiting its compute density. Besides, CIM’s analog compute is sensitive to process variability and mismatches. The transistor nonlinearity also significantly degrades the compute accuracy. Stochastic Computing (SC), which represents numbers as probability of 1s in random binary streams, is a digital-compute scheme that uses tiny MACs and offers high compute density without ADC/DAC (Fig. 1). Simulations show 4x reduction of memory access compared to 8b fixed-point digital accelerators due to the massive parallelism it can achieve using the tiny digital logic gates (AND/OR). However, SC suffers from high cost of binary to stochastic number conversion and compute error.
更多查看译文
关键词
deep learning,all-digital,stochastic-compute-in-memory
AI 理解论文
溯源树
样例
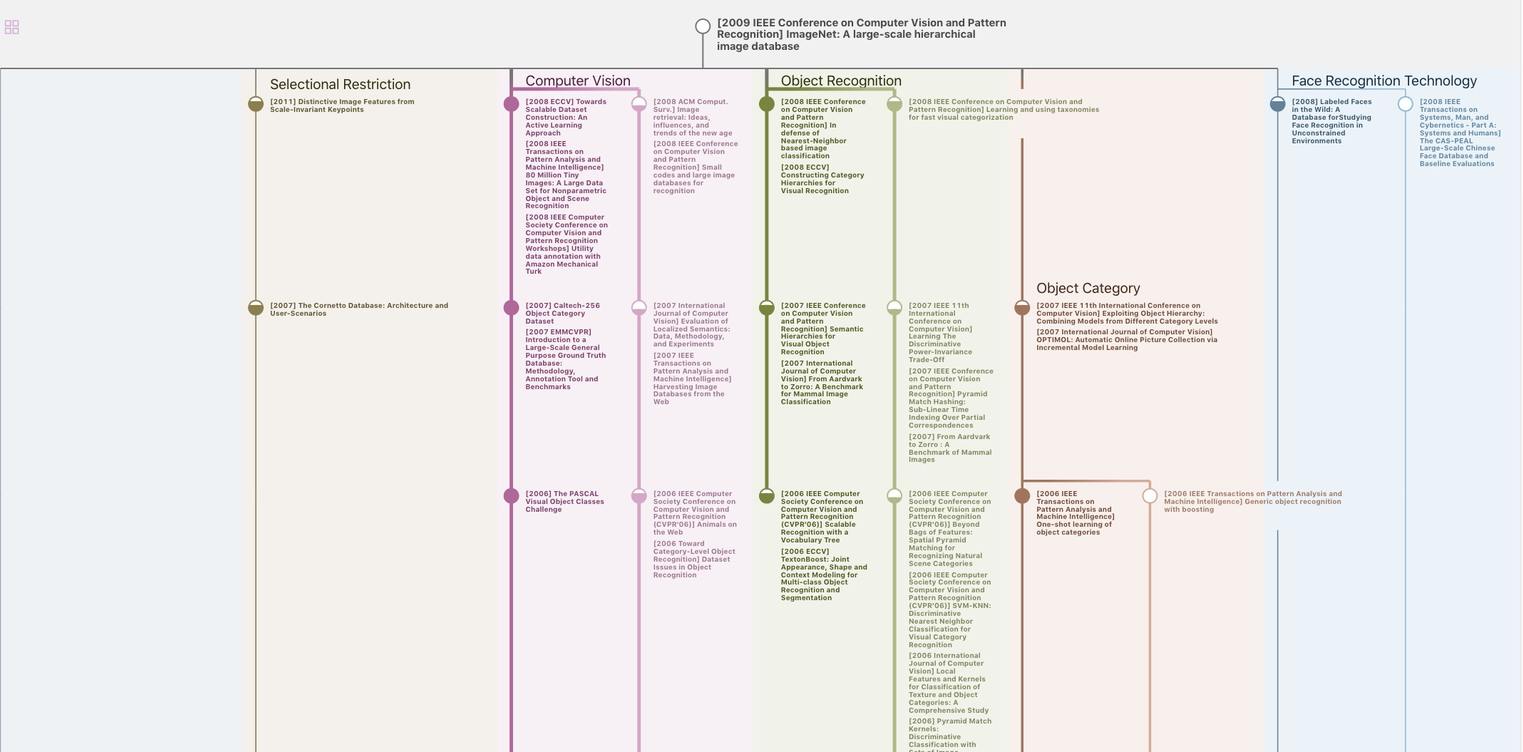
生成溯源树,研究论文发展脉络
Chat Paper
正在生成论文摘要