Time-frequency Representation Optimization using InfoGAN Latent Codes
2022 30th Telecommunications Forum (TELFOR)(2022)
摘要
Time-frequency analysis can provide an understanding how the spectral properties of a signal vary with time from a physical and mathematical point of view, thus it has drawn increasing attention to acoustic, sonar, radar signals, etc. However, for multiple component signals, or nonlinear modulated signals it is always a trade-off between the energy concentration and cross terms. Despite numerous time-frequency analysis techniques to alleviate this contradiction, they still require careful manipulation of their parameters in calculating optimal time-frequency representation. In this paper, we utilize a novel generative model with latent codes, information maximization generative adversarial network (InfoGAN), to learn the relation between concentration and cross terms in an unsupervised manner. It is the first attempt to apply InfoGAN in synthesizing optimal time-frequency representation and the experimental results demonstrate the feasibility to obtain the optimal time-frequency representation by adjusting latent codes in InfoGAN.
更多查看译文
关键词
Time-frequency analysis,Generative Adversarial Networks,InfoGAN
AI 理解论文
溯源树
样例
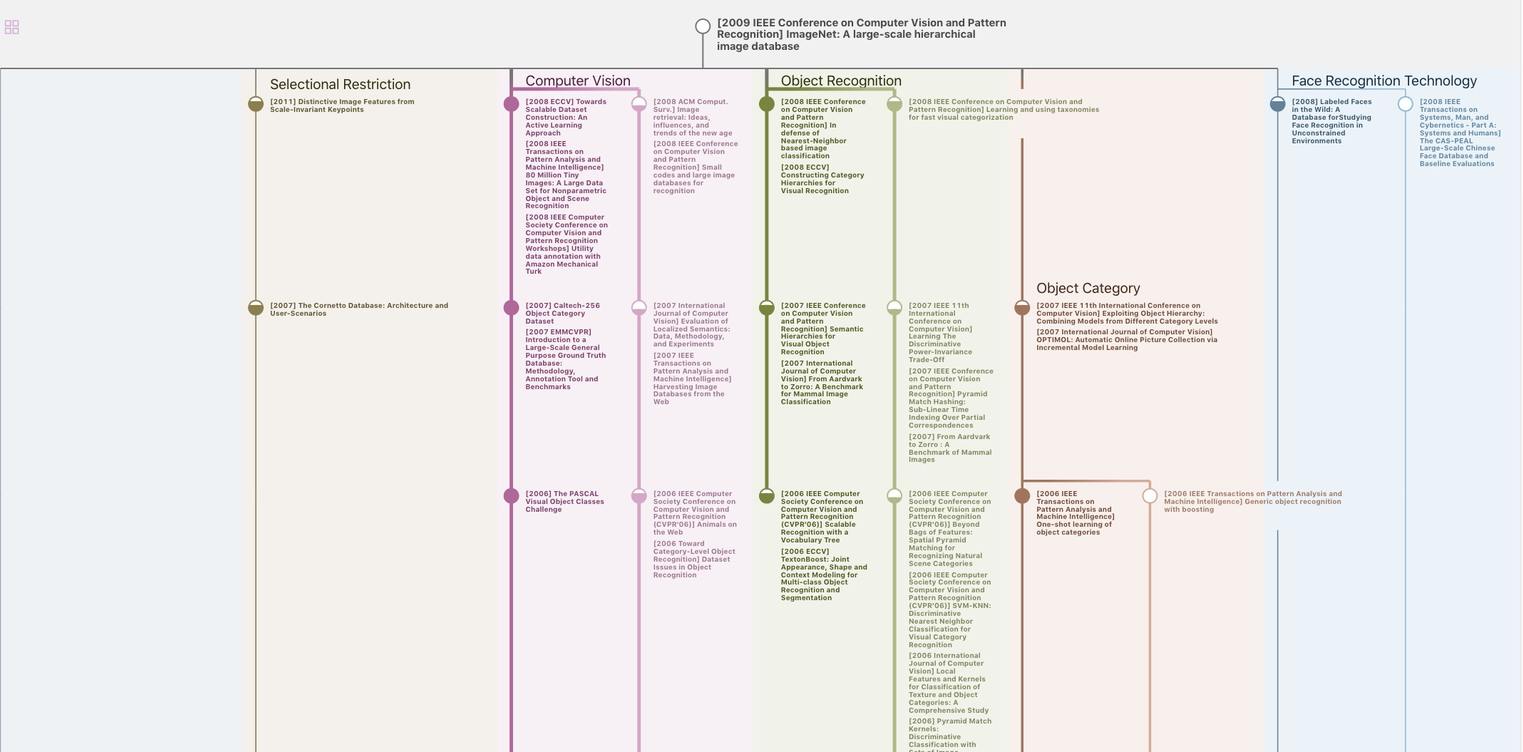
生成溯源树,研究论文发展脉络
Chat Paper
正在生成论文摘要