Utilizing Uncertainty of Time Series Prediction in Spectrum Sharing with Radar Systems
2022 30th Telecommunications Forum (TELFOR)(2022)
摘要
Interference prediction with neural networks (NNs) can help in proactive resource management for spectrum sharing in 5.6 GHz radar bands. This can achieve high data rates for secondary users (SUs) of the shared spectrum and enhance the protection of the incumbent radar systems. The recently introduced efficient sharing and radar protection (ESRP) system with interference prediction used NN-based long short-term memory (LSTM) and Monte Carlo (MC) dropout to utilize the uncertainties in the interference from the access point (APs). Due to the random nature of radio propagation, the permissible probability of harmful interference at the radar (ε
p
) for the ESRP system varies depending on the MC dropout and prediction intervals (PIs) which represents the amount of uncertainty captured in the system. In this work, we use a gated recurrent unit (GRU) which is simpler and faster than LSTM for interference prediction. We also investigate how the different MC dropout values can vary the parameter ε
p
and improve the radar protection performance of the ESRP system. The results show that radar protection performance can increase by using GRU and the values of MC dropout play an important role in the ESRP system ensuring better radar protection with a small trade-off for throughput of the SUs which are the APs.
更多查看译文
关键词
Dropout,Forecasting,GRU,LSTM,Neural Networks,Radars,Real Network Data,Time Series,WLAN
AI 理解论文
溯源树
样例
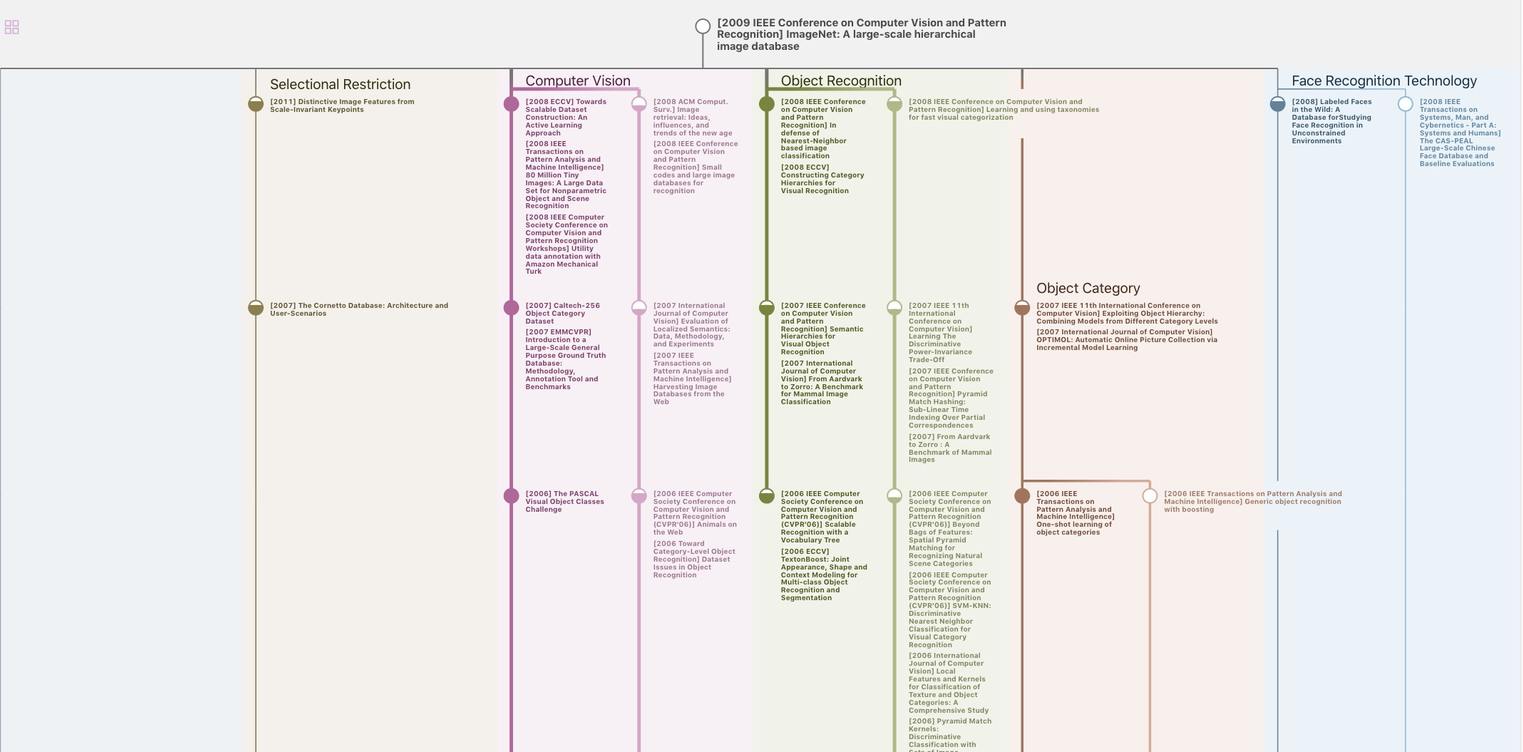
生成溯源树,研究论文发展脉络
Chat Paper
正在生成论文摘要