Private Data Preprocessing for Privacy-preserving Federated Learning
2022 IEEE 5th International Conference on Knowledge Innovation and Invention (ICKII )(2022)
摘要
Privacy-preserving federated learning can accomplish model aggregation without leaking to a local model and avoid the problem of sensitive data exposure caused by model leakage. Even though it protects privacy in the training process, any data analysis task proposed by the initiator and the type of data required for the task contains the research or trade secrets of the organization. Being intercepted in the transmission process or known by other data providers, the disclosure of essential research secrets occurs, leading to the theft of research or business ideas. Therefore, it is a critical issue to achieve data matching between the initiator and the participant under the premise of privacy protection. In this study, we propose a federated learning framework that considers the above security issues. A privacy-preserving federated learning architecture based on homomorphic encryption is designed to protect each participant's data and local model. In addition, encrypted query technology is used in this architecture to provide data privacy matching. The data provider searches the data in ciphertext, finds the encrypted data that meets the conditions, and completes the training process without disclosing any requirements of the task initiator.
更多查看译文
关键词
privacy-preserving federated learning,data privacy-matching,encrypted query
AI 理解论文
溯源树
样例
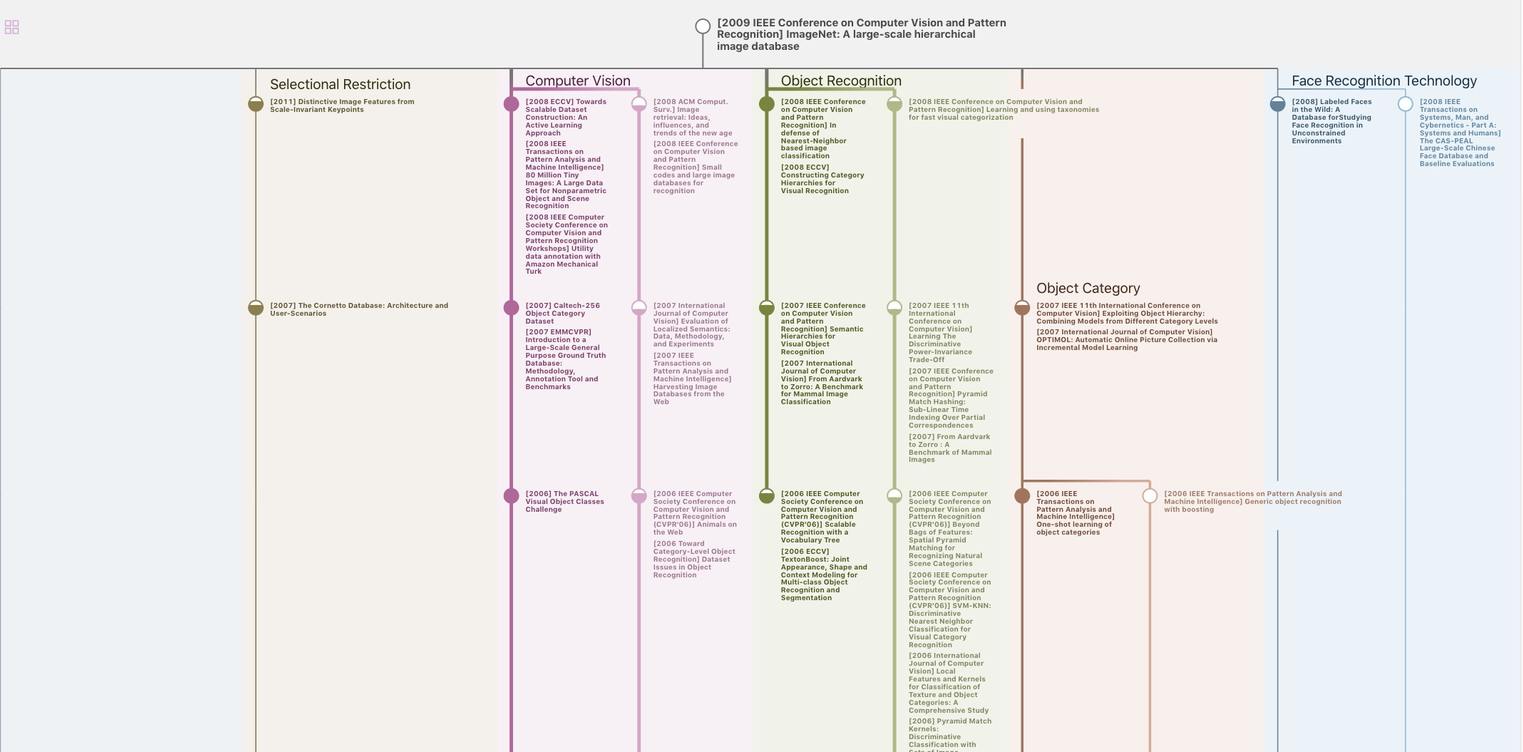
生成溯源树,研究论文发展脉络
Chat Paper
正在生成论文摘要