Learning to Sharpen Partially Blurred Image via Iterative Blurred Region Mining and Recovery
2022 Asia-Pacific Signal and Information Processing Association Annual Summit and Conference (APSIPA ASC)(2022)
摘要
Despite progress on image deblurring, existing advanced deep-net models are difficult to directly process partially blurred images due to the mismatch in the distribution of training (full-scaled blurred) and test (partially blurred) images. To address this problem, we presents an iterative blurred region mining and recovery (iBRMR) approach, which can automatically and progressively mine and sharpen the blurred regions. Starting with a collaborative mechanism of two coupled deep-net models, our approach first learns a blur detector to locally discover evidence of blurred regions. We name this the blurred region mining process. Then, we construct a blur refiner to improve sharpness of the most blurred region. We name this the blurred region recovery process. We iterate the mining and recovery processes to sharpen the image. Our iBRMR approach takes advantage of the two processes' complementary nature. It functions as a bridge between the blur detector and the blur refiner, yielding the performance synergy in better solving the deblurring of partially blurred image. Experimental results on benchmark datasets demonstrate that the proposed iBRMR approach not only achieves superior deblurring performance in partially blurred images, but also performs well in full-scaled blurred images. The video demos can be found at https://sites.google.com/view/ibrmr.
更多查看译文
关键词
iterative blurred region mining,blurred image,learning
AI 理解论文
溯源树
样例
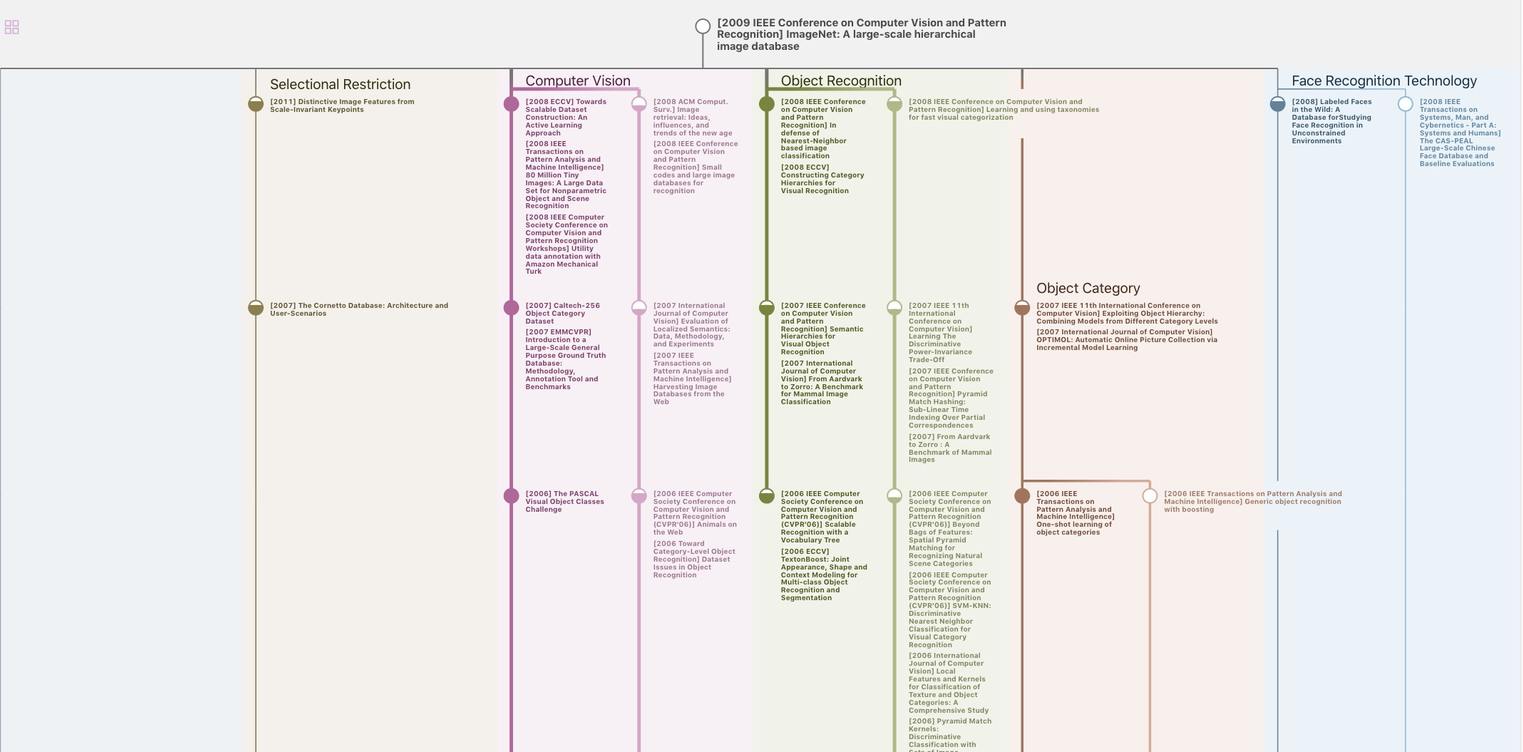
生成溯源树,研究论文发展脉络
Chat Paper
正在生成论文摘要