Semi-supervised learning with pseudo-negative labels for image classification
Knowledge-Based Systems(2023)
摘要
Semi-supervised learning frameworks usually adopt mutual learning approaches with multiple submodels to learn from different perspectives. Usually, a high threshold is used to filter out numerous low-confidence predictions for unlabeled data to avoid transferring erroneous pseudo-labels between the submodels. However, such filtering cannot fully exploit unlabeled data with low prediction confidence. In this study, we propose a mutual learning framework based on pseudo-negative labels to overcome this problem. Negative labels are those to which a corresponding data item does not belong. In each iteration, one submodel generates pseudo-negative labels for each data item, and the other submodel learns from these labels. The roles of the two submodels interchange after each iteration until convergence. The dual model can improve its prediction ability by reducing the prediction probability of the pseudo-negative labels. We also propose a mechanism for selecting a few pseudo-negative labels to be fed into the submodels. In experiments, our framework achieved state-of-the-art results on several main benchmarks. Specifically, with our framework, the error rates of the 13-layer CNN model were 9.35% and 7.94% for CIFAR-10 with 1000 and 4000 labels, respectively. In addition, our framework has an error rate of 0.81% for the non-augmented MNIST with only 20 labels, which is much smaller than that of the other approaches. Our approach also demonstrated a significant performance improvement in domain adaptation.
更多查看译文
关键词
Semi-supervised learning,Image classification,Mutual learning
AI 理解论文
溯源树
样例
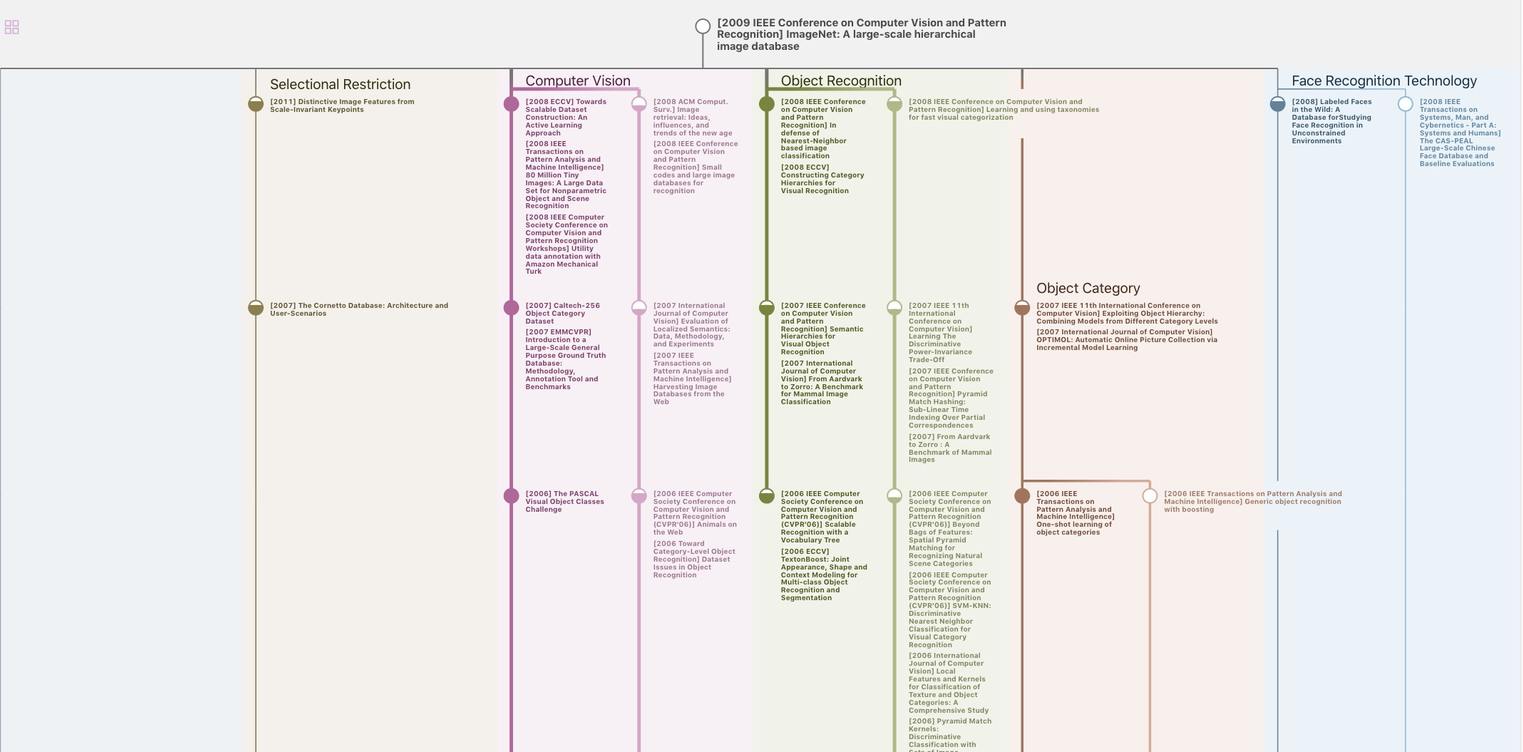
生成溯源树,研究论文发展脉络
Chat Paper
正在生成论文摘要