Time-aware Hyperbolic Graph Attention Network for Session-based Recommendation
2022 IEEE International Conference on Big Data (Big Data)(2022)
摘要
Session-based Recommendation (SBR) is to predict users’ next interested items based on their previous browsing sessions. Existing methods model sessions as graphs or sequences to estimate user interests based on their interacted items to make recommendations. In recent years, graph-based methods have achieved outstanding performance on SBR. However, none of these methods consider temporal information, which is a crucial feature in SBR as it indicates timeliness or currency. Besides, the session graphs exhibit a hierarchical structure and are demonstrated to be suitable in hyperbolic geometry. But few papers design the models in hyperbolic spaces and this direction is still under exploration.In this paper, we propose Time-aware Hyperbolic Graph Attention Network (TA-HGAT) — a novel hyperbolic graph neural network framework to build a session-based recommendation model considering temporal information. More specifically, there are three components in TA-HGAT. First, a hyperbolic projection module transforms the item features into hyperbolic space. Second, the time-aware graph attention module models time intervals between items and the users’ current interests. Third, an evolutionary loss at the end of the model provides an accurate prediction of the recommended item based on the given timestamp. TA-HGAT is built in a hyperbolic space to learn the hierarchical structure of session graphs. Experimental results show that the proposed TA-HGAT has the best performance compared to ten baseline models on two real-world datasets.
更多查看译文
关键词
recommender system,graph neural network,hyperbolic embedding
AI 理解论文
溯源树
样例
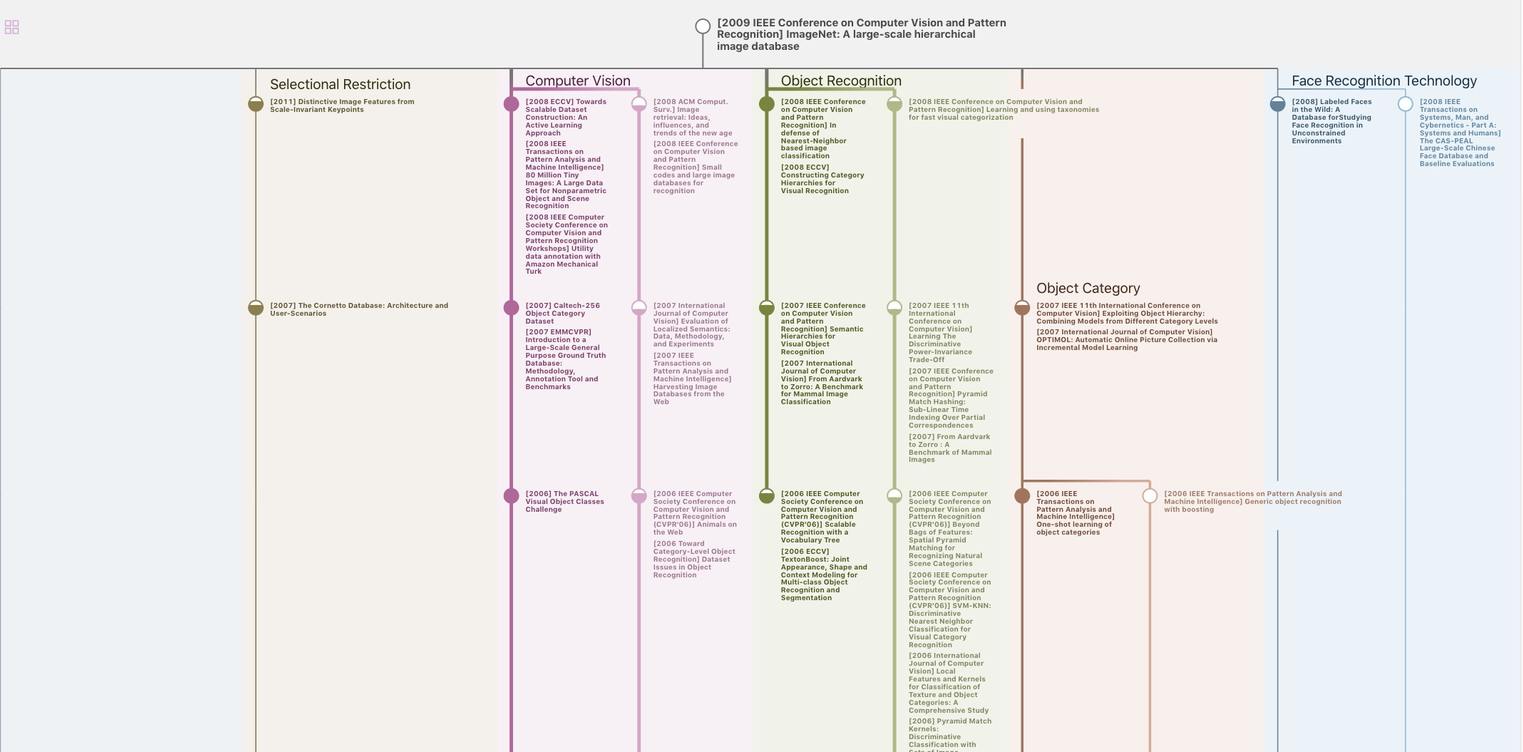
生成溯源树,研究论文发展脉络
Chat Paper
正在生成论文摘要