Network Traffic Anomaly Prediction for Beyond 5G Networks
2022 IEEE 33rd Annual International Symposium on Personal, Indoor and Mobile Radio Communications (PIMRC)(2022)
摘要
Network traffic anomalies can have a detrimental effect on end-to-end network performance and reliability, compromising severely (demanding) services and applications, such as those offered by the fifth-generation (5G) mobile networks and beyond. To prevent the network drift towards increasingly inefficient operating modes, such traffic anomalies need to be detected first, so that the proper actions be taken to avoid such undesirable network drift. This paper proposes a novel framework focusing on proactively detecting such traffic anomalies, that is, predicting upcoming network traffic anomalies before they actually occur. To this end, two mechanisms are developed: a mechanism aiming at identifying different network traffic behaviors in an automated manner; and a mechanism for predicting network traffic behaviors for the next time interval spanning several seconds. The automated network traffic anomaly identification is realized via the application of clustering and decision tree-based learning. A time series model (specifically, a Bidirectional Long Short Term Memory (BiLSTM) Autoencoder) is employed for the proactive detection of forthcoming network traffic behaviors. Evaluation results are derived showing a prediction accuracy of up to 90.02%, demonstrating the effectiveness and viability of the proposed framework, as well as its potential for higher effectiveness compared to a state-of-the-art solution.
更多查看译文
关键词
5G,Anomaly Prediction,Machine Learning,Artificial Intelligence,6G
AI 理解论文
溯源树
样例
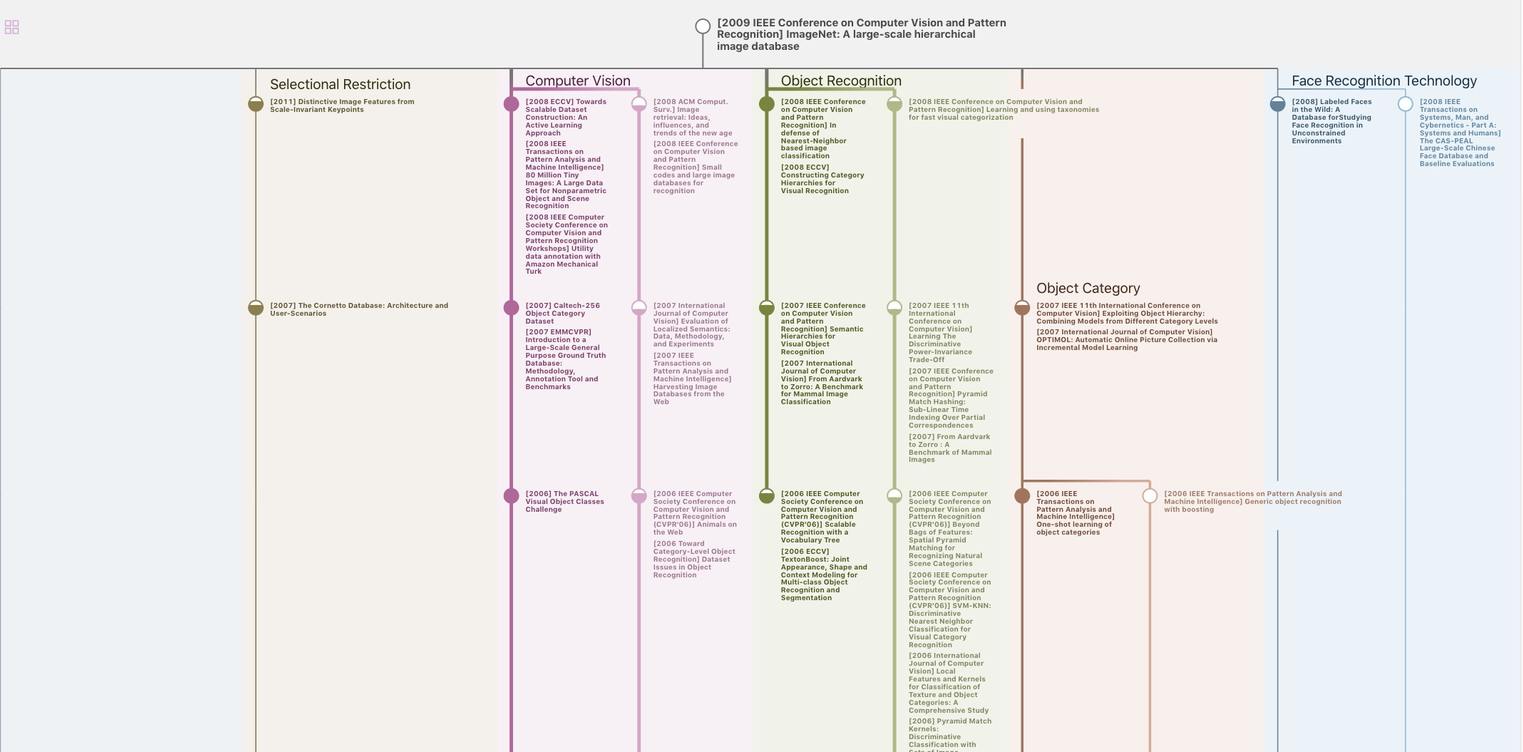
生成溯源树,研究论文发展脉络
Chat Paper
正在生成论文摘要