Empirical Analysis of Sentence Embedding Techniques for Answer Retrieval in Marathi Question Answering
2022 Sixth International Conference on I-SMAC (IoT in Social, Mobile, Analytics and Cloud) (I-SMAC)(2022)
摘要
It is vital that any type of text can be portrayed in a machine-readable language so that it can be processed and interpreted. Text embeddings, which allow a machine to interpret any type of text by mathematically expressing it, naturally minimise this work. To extract the concept of interdependence across words or sentences, the degree of connection, synonym recognition, idea segmentation, selectional preferences, and analogies, text embedding are very useful. Using closest neighbor lookup of vectors, a vector representation of a text in a semantic vector space may be utilized for answer retrieval according to the vectors’ similarity and proximity. Traditional QA systems require a huge number of high-quality language-specific resources to perform multiple activities. The precision of several embedding techniques presently supporting Marathi, an Indo-Aryan language, is explored in this research. The performance of several sentence embedding algorithms is evaluated to select the most relevant and related answer text within the corpus given a natural language query in Marathi. Pragmatic tests are performed across supervised machine learning models to perform categorization and sentence embeddings in order to evaluate and compare the veracity of various embedding techniques. The proposed research findings concentrate on identifying the most effective embedding approaches from the restricted resources available for the Marathi language, laying the groundwork for future study in this language.
更多查看译文
关键词
Natural Language Processing,Document and Text Processing,Machine Learning
AI 理解论文
溯源树
样例
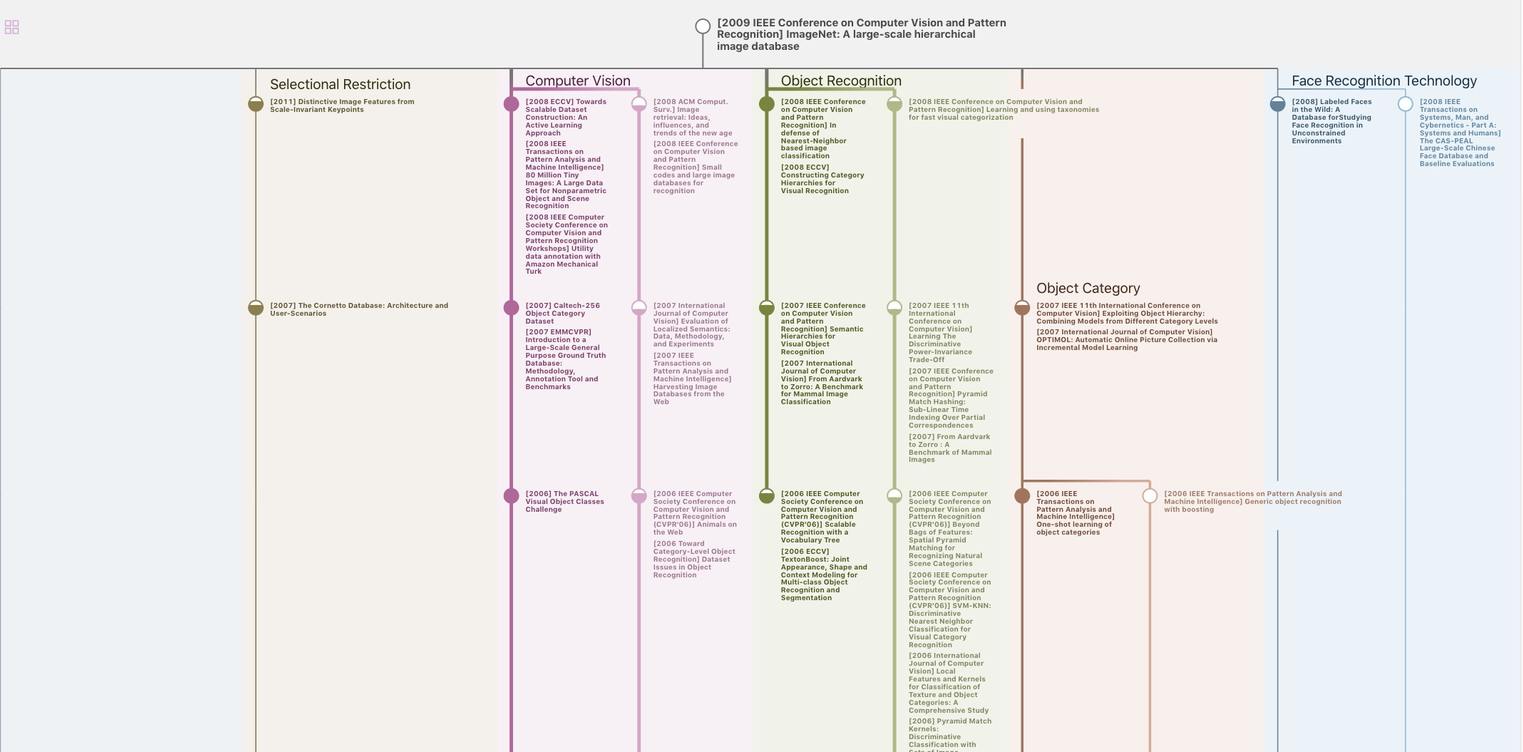
生成溯源树,研究论文发展脉络
Chat Paper
正在生成论文摘要