Data-driven spatiotemporal modeling for structural dynamics on irregular domains by stochastic dependency neural estimation
Computer Methods in Applied Mechanics and Engineering(2023)
摘要
Numerical simulations for spatiotemporal processes involving material, geometrical and contact nonlinearities might be computationally prohibitive for many-evaluation applications. Considering the strong nonlinearity of dynamic processes, the prediction of high-dimensional spatiotemporal responses remains challenging for most surrogate-based models. To address such issues, a Stochastic Dependency Neural Estimator (SDNE) is proposed to construct the mapping from the input parameter domain to the high-dimensional spatiotemporal response domain, revealing the underlying relationship between the high-dimensional regression estimation and manifold distribution hypothesis. Moreover, Graph Neural Networks (GNN) and Temporal Convolutional Neural Networks (TCN) are integrated to capture nonlinear features from irregular geometries and time series simultaneously. Two high-speed impact cases involving large deformation and highly nonlinear transients are investigated to validate the proposed method. The proposed SDNE achieves highly accurate prediction compared with Finite Element (FE) evaluations. To further validate the practical application of the proposed method, multi-objective crashworthiness optimizations are performed with the proposed SDNE. The results show that the proposed SDNE provides reliable predictions for spatiotemporal dynamics
更多查看译文
关键词
Data-driven modeling,Spatiotemporal dynamics,Deep learning,Graph neural networks
AI 理解论文
溯源树
样例
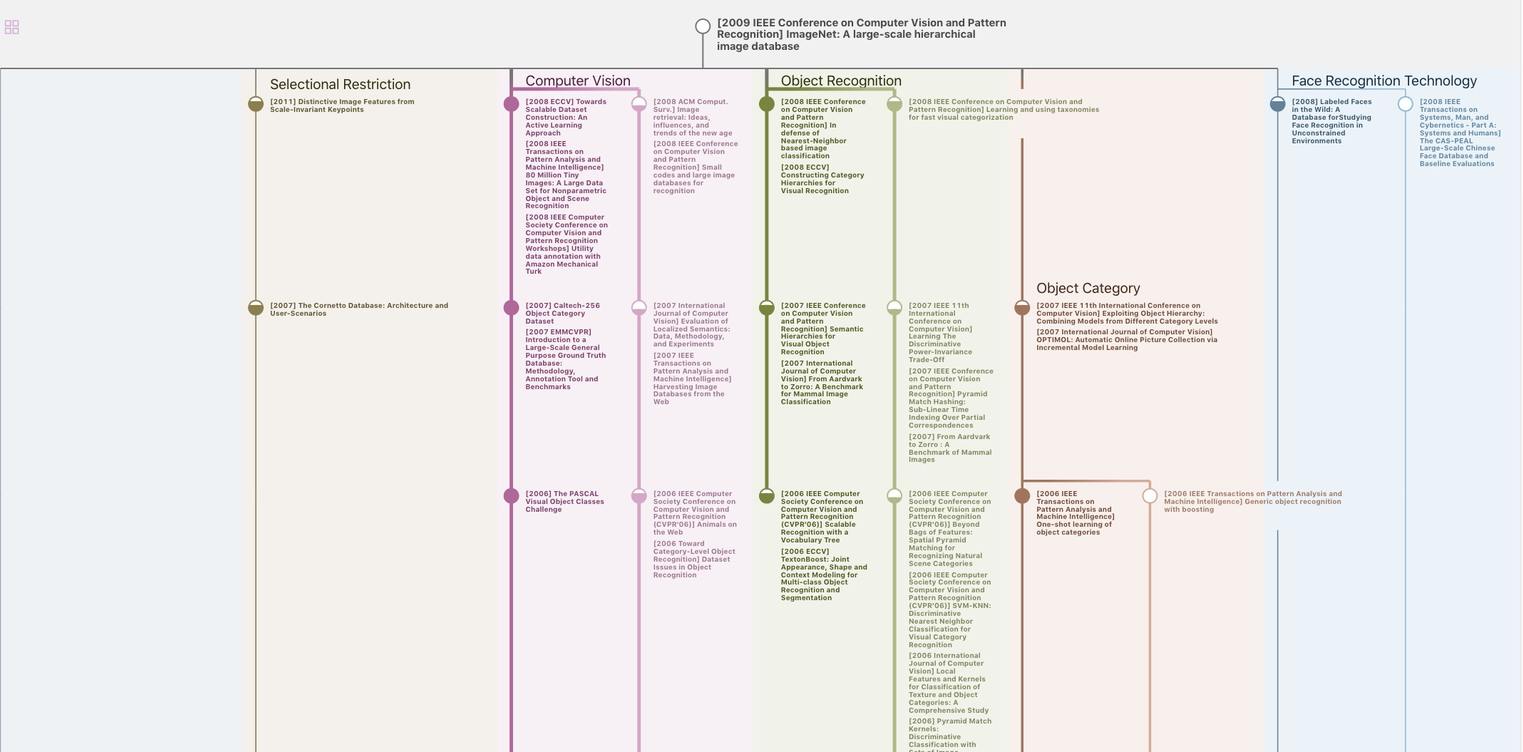
生成溯源树,研究论文发展脉络
Chat Paper
正在生成论文摘要