Smoothly varying regularization
Computational Statistics & Data Analysis(2023)
摘要
A basis expansion with regularization methods is much appealing to the flexible or robust nonlinear regression models for data with complex structures. When the underlying function has inhomogeneous smoothness, it does not perform well when one tries to estimate it with regularization methods that do not require intensive computational load. Specifically, when the underlying function has both smooth and non-smooth parts, the conventional regularization methods tend to over-fit in the smooth part or under-fit in the non-smooth part. Therefore, a new efficient basis expansion is considered by proposing a smoothly varying regularization method which is constructed by some special penalties. These should be called adaptive penalties. In the modeling, adaptive-type penalties play key roles and it has been successful in giving good estimation for inhomogeneous smoothness functions. A crucial issue in the modeling process is the choice of a suitable model among candidates. To select the suitable model, an approximated generalized information criterion (GIC) is derived. The proposed method is investigated through Monte Carlo simulations and real data analysis. Numerical results suggest that the proposed method performs well in various situations.
更多查看译文
关键词
Basis expansion,Curve and surface fitting,Information criterion,Model selection,Smoothness,Tuning parameter
AI 理解论文
溯源树
样例
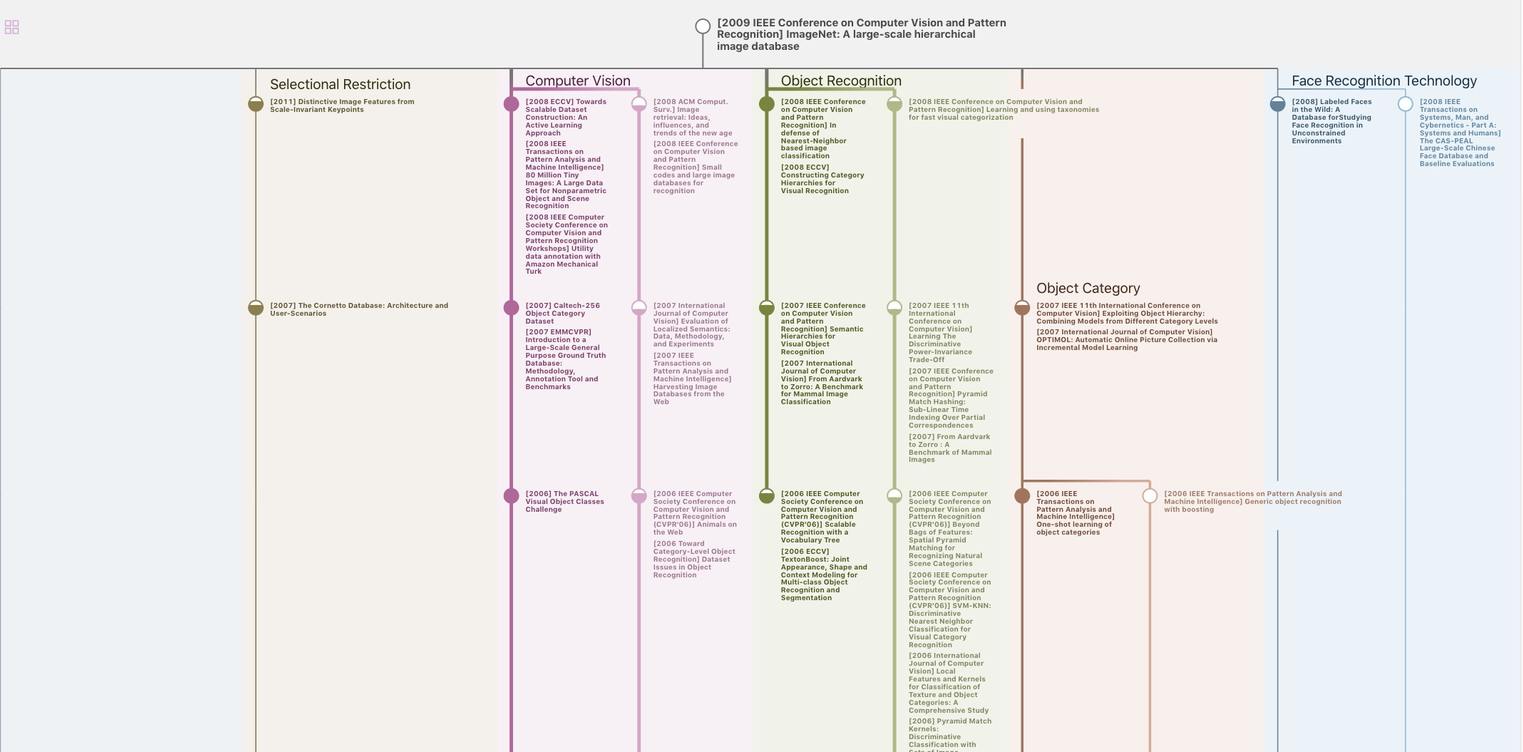
生成溯源树,研究论文发展脉络
Chat Paper
正在生成论文摘要