Supervised learning with probability interpretation in airfoil transition judgment
Chinese Journal of Aeronautics(2023)
摘要
Transition prediction has always been a frontier issue in the field of aerodynamics. A supervised learning model with probability interpretation for transition judgment based on experimental data was developed in this paper. It solved the shortcomings of the point detection method in the experiment, that which was often only one transition point could be obtained, and comparison of multi-point data was necessary. First, the Variable-Interval Time Average (VITA) method was used to transform the fluctuating pressure signal measured on the airfoil surface into a sequence of states which was described by Markov chain model. Second, a feature vector consisting of one-step transition matrix and its stationary distribution was extracted. Then, the Hidden Markov Model (HMM) was used to pre-classify the feature vectors marked using the traditional Root Mean Square (RMS) criteria. Finally, a classification model with probability interpretation was established, and the cross-validation method was used for model validation. The research results show that the developed model is effective and reliable, and it has strong Reynolds number generalization ability. The developed model was theoretically analyzed in depth, and the effect of parameters on the model was studied in detail. Compared with the traditional RMS criterion, a reasonable transition zone can be obtained using the developed classification model. In addition, the developed model does not require comparison of multi-point data. The developed supervised learning model provides new ideas for the transition detection in flight experiments and other experiments.
更多查看译文
关键词
Classification model,Hidden Markov model,Markov chain model,Supervised learning,Transition judgment
AI 理解论文
溯源树
样例
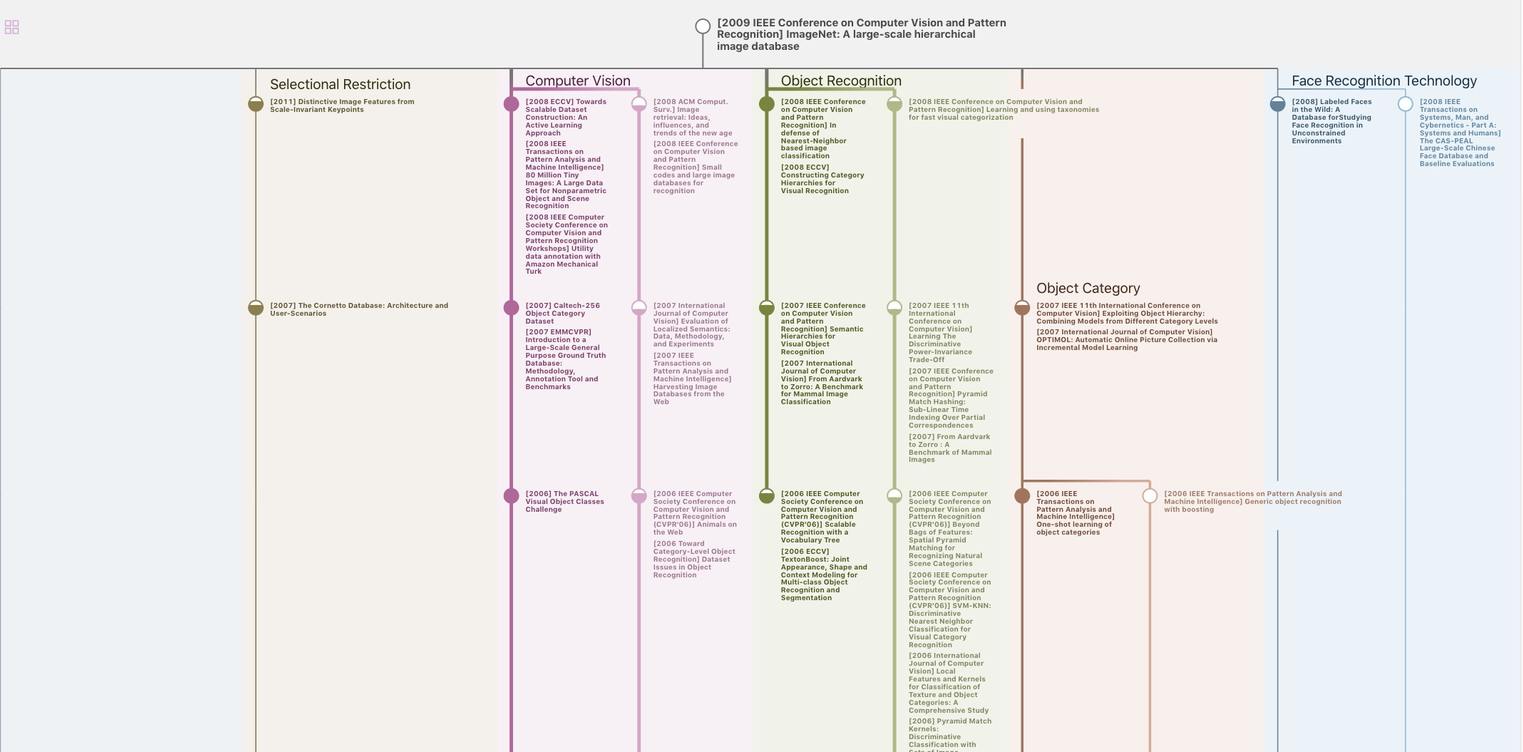
生成溯源树,研究论文发展脉络
Chat Paper
正在生成论文摘要