Effects of chemical potential differences on methane hydrate formation kinetics
Chemical Engineering Journal(2023)
摘要
To underpin the increasing interest in practical applications of gas hydrates, for gas storage and separation for instance, the formation and growth of hydrates at liquid-gas interfaces are of fundamental importance. Although the thermodynamics of hydrate formation has been widely studied and is well understood, the kinetics of these processes is not well characterised. In this work, a high-pressure, low-temperature stirred reactor was used to conduct hydrate formation kinetic studies in a temperature range from 276.5 to 283.5 K and a pressure range from 5 to 10.5 MPa, with a special focus on 1) the impact of agitation conditions on the available water-gas interfacial surface area for mass transfer and growth rate during hydrate formation, and 2) the effect of the chemical potential driving force on the formation rate. Five hydrate growth regimes were identified, with varying degrees of gas mass transfer control across the gas-water interface depending on the extent to which hydrate layers built up at this interface, gas needed to move through solid hydrate layers, and the extent to which the gas was entrained within the water phase. The formation rate in the initial linear growth regime, before the onset of solid hydrate gas mass transfer effects, was found to depend in an essentially exponential manner on the chemical potential difference from the equilibrium state. Semi-empirical models related to Arrhenius-type kinetic models were used to correlate the data, the best of which reproduced the formation rates from the chemical potential differences to within +/- 5 %. The approach has general applicability to help determine the balance between kinetic and thermodynamic factors in identifying the optimum pressure-temperature conditions for processes for gas storage, gas separation and other hydrate applications.
更多查看译文
关键词
Methane hydrates,Hydrate formation kinetics,Gas hydrate formation empirical model,Chemical potential driving force
AI 理解论文
溯源树
样例
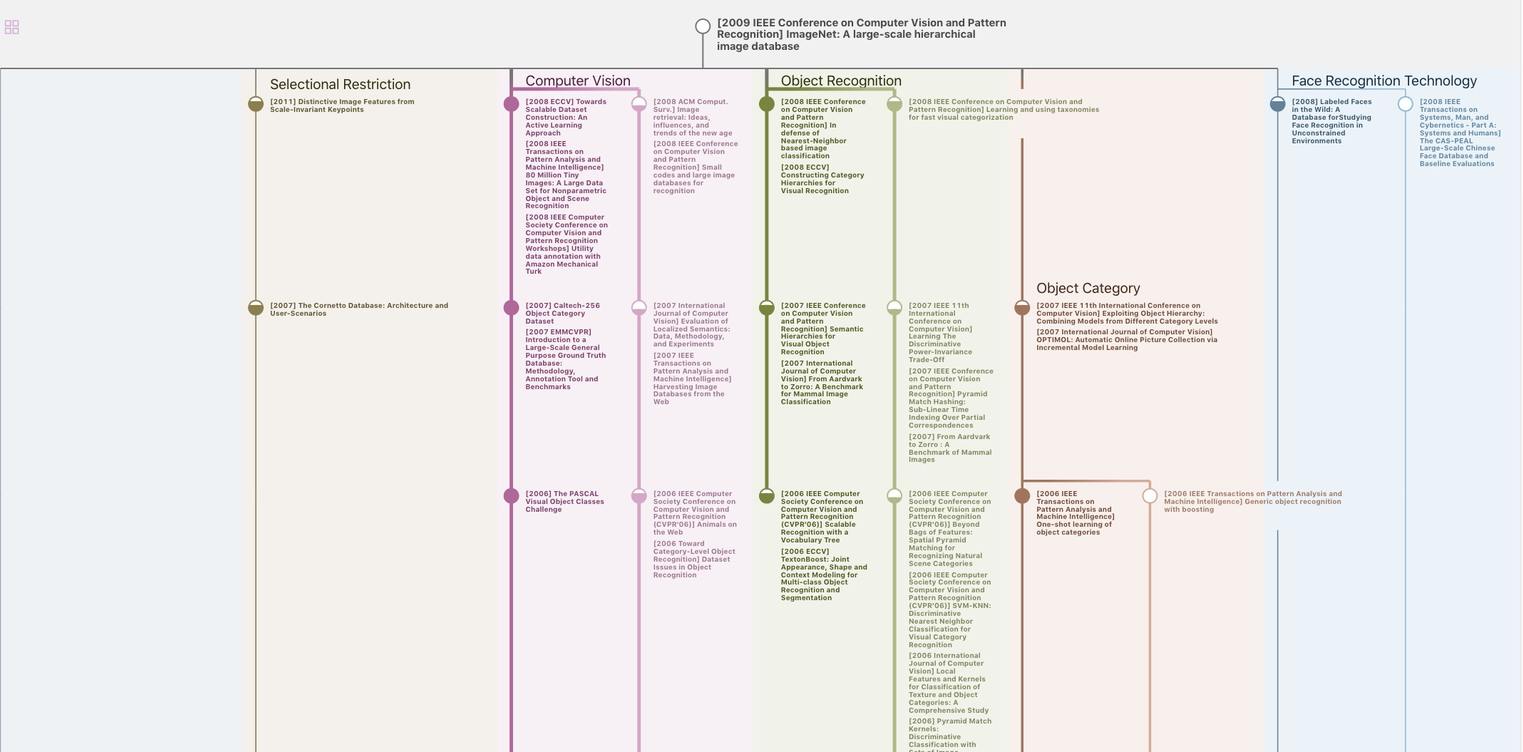
生成溯源树,研究论文发展脉络
Chat Paper
正在生成论文摘要