An end-end deep learning framework for lesion segmentation on multi-contrast MR images—an exploratory study in a rat model of traumatic brain injury
Medical & Biological Engineering & Computing(2023)
摘要
Traumatic brain injury (TBI) engenders traumatic necrosis and penumbra—areas of secondary neural injury which are crucial targets for therapeutic interventions. Segmenting manually areas of ongoing changes like necrosis, edema, hematoma, and inflammation is tedious, error-prone, and biased. Using the multi-parametric MR data from a rodent model study, we demonstrate the effectiveness of an end-end deep learning global-attention-based UNet (GA-UNet) framework for automatic segmentation and quantification of TBI lesions. Longitudinal MR scans (2 h, 1, 3, 7, 14, 30, and 60 days) were performed on eight Sprague–Dawley rats after controlled cortical injury was performed. TBI lesion and sub-regions segmentation was performed using 3D-UNet and GA-UNet. Dice statistics (DSI) and Hausdorff distance were calculated to assess the performance. MR scan variations-based (bias, noise, blur, ghosting) data augmentation was performed to develop a robust model. Training/validation median DSI for U-Net was 0.9368 with T2w and MPRAGE inputs, whereas GA-UNet had 0.9537 for the same. Testing accuracies were higher for GA-UNet than U-Net with a DSI of 0.8232 for the T2w-MPRAGE inputs. Longitudinally, necrosis remained constant while oligemia and penumbra decreased, and edema appearing around day 3 which increased with time. GA-UNet shows promise for multi-contrast MR image-based segmentation/quantification of TBI in large cohort studies. Graphical Abstract
更多查看译文
关键词
Traumatic brain injury, U-Net, Global attention, Self-attention, Deep learning, Segmentation, Controlled cortical impact
AI 理解论文
溯源树
样例
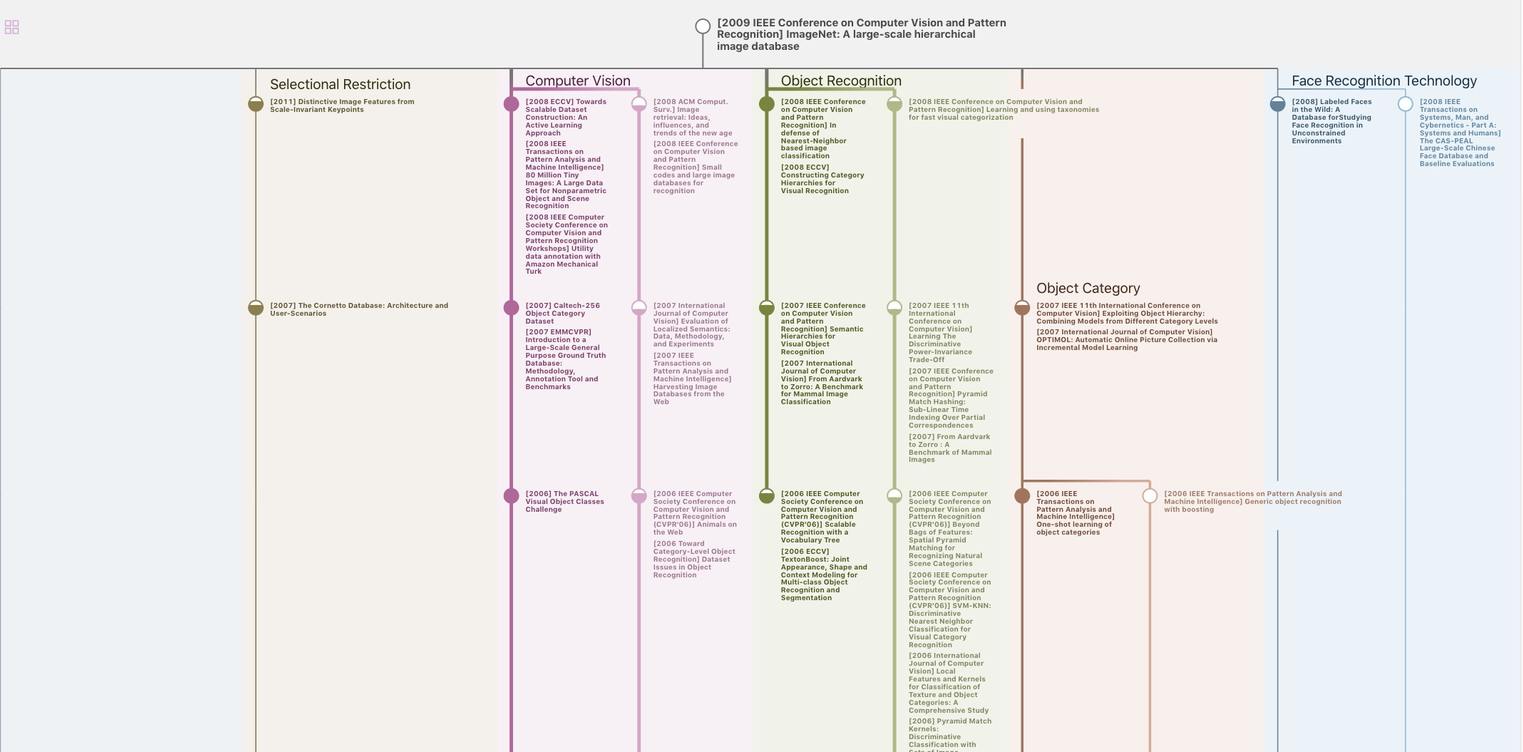
生成溯源树,研究论文发展脉络
Chat Paper
正在生成论文摘要