Efficient Attack Detection in IoT Devices using Feature Engineering-Less Machine Learning
arxiv(2023)
摘要
Through the generalization of deep learning, the research community has addressed critical challenges in the network security domain, like malware identification and anomaly detection. However, they have yet to discuss deploying them on Internet of Things (IoT) devices for day-to-day operations. IoT devices are often limited in memory and processing power, rendering the compute-intensive deep learning environment unusable. This research proposes a way to overcome this barrier by bypassing feature engineering in the deep learning pipeline and using raw packet data as input. We introduce a feature engineering-less machine learning (ML) process to perform malware detection on IoT devices. Our proposed model, "Feature engineering-less-ML (FEL-ML)," is a lighter-weight detection algorithm that expends no extra computations on "engineered" features. It effectively accelerates the low-powered IoT edge. It is trained on unprocessed byte-streams of packets. Aside from providing better results, it is quicker than traditional feature-based methods. FEL-ML facilitates resource-sensitive network traffic security with the added benefit of eliminating the significant investment by subject matter experts in feature engineering.
更多查看译文
关键词
iot devices,machine learning,feature,engineering-less
AI 理解论文
溯源树
样例
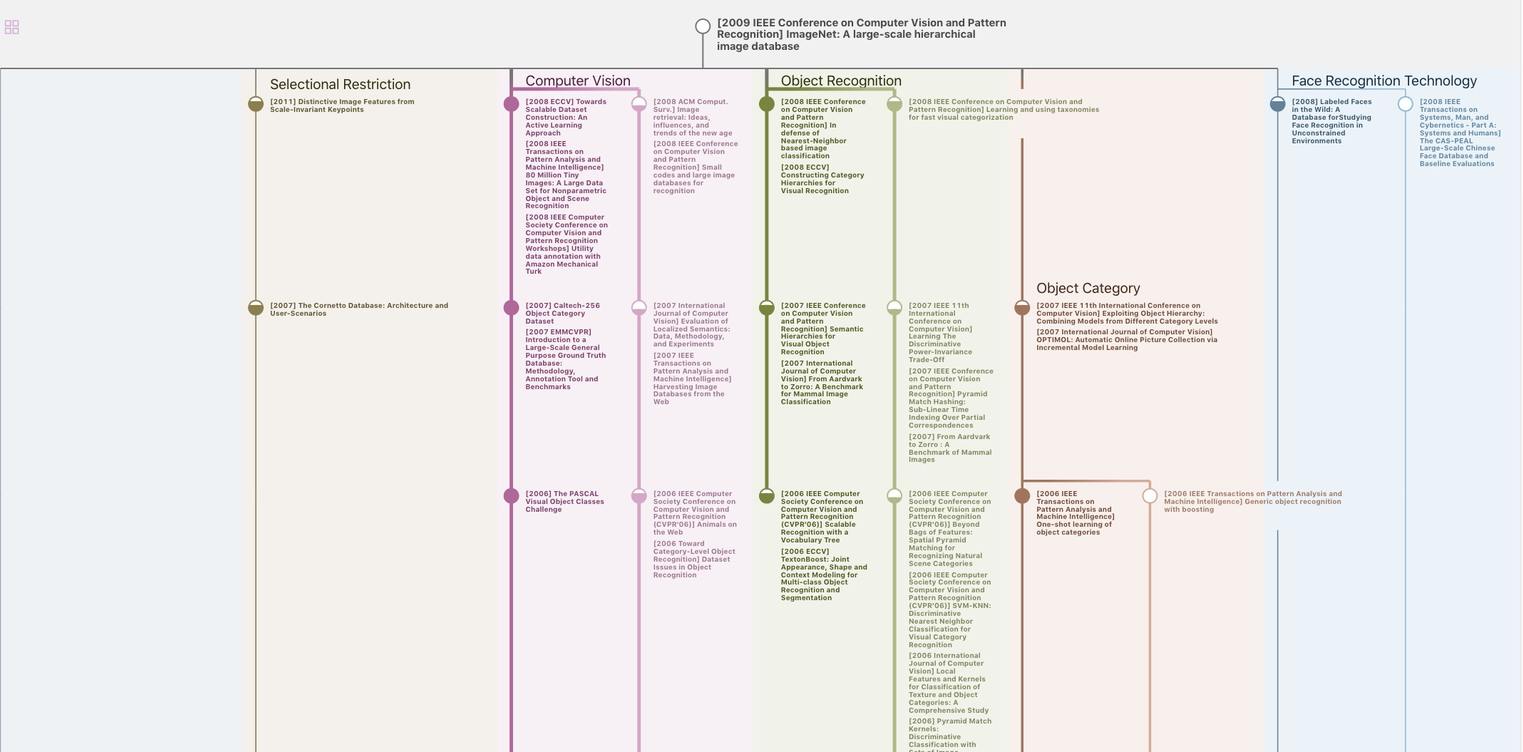
生成溯源树,研究论文发展脉络
Chat Paper
正在生成论文摘要