Learning-Based Design and Control for Quadrupedal Robots With Parallel-Elastic Actuators
IEEE Robotics and Automation Letters(2023)
摘要
Parallel-elastic joints can improve the efficiency and strength of robots by assisting the actuators with additional torques. For these benefits to be realized, a spring needs to be carefully designed. However, designing robots is an iterative and tedious process, often relying on intuition and heuristics. We introduce a design optimization framework that allows us to co-optimize a parallel elastic knee joint and locomotion controller for quadrupedal robots with minimal human intuition. We design a parallel elastic joint and optimize its parameters with respect to the efficiency in a model-free fashion. In the first step, we train a design-conditioned policy using model-free Reinforcement Learning, capable of controlling the quadruped in the predefined range of design parameters. Afterwards, we use Bayesian Optimization to find the best design using the policy. We use this framework to optimize the parallel-elastic spring parameters for the knee of our quadrupedal robot ANYmal together with the optimal controller. We evaluate the optimized design and controller in real-world experiments over various terrains. Our results show that the new system improves the torque-square efficiency of the robot by 33% compared to the baseline and reduces maximum joint torque by 30% without compromising tracking performance. The improved design resulted in 11% longer operation time on flat terrain.
更多查看译文
关键词
Compliant joints and mechanisms,legged robots,mechanism design,reinforcement learning
AI 理解论文
溯源树
样例
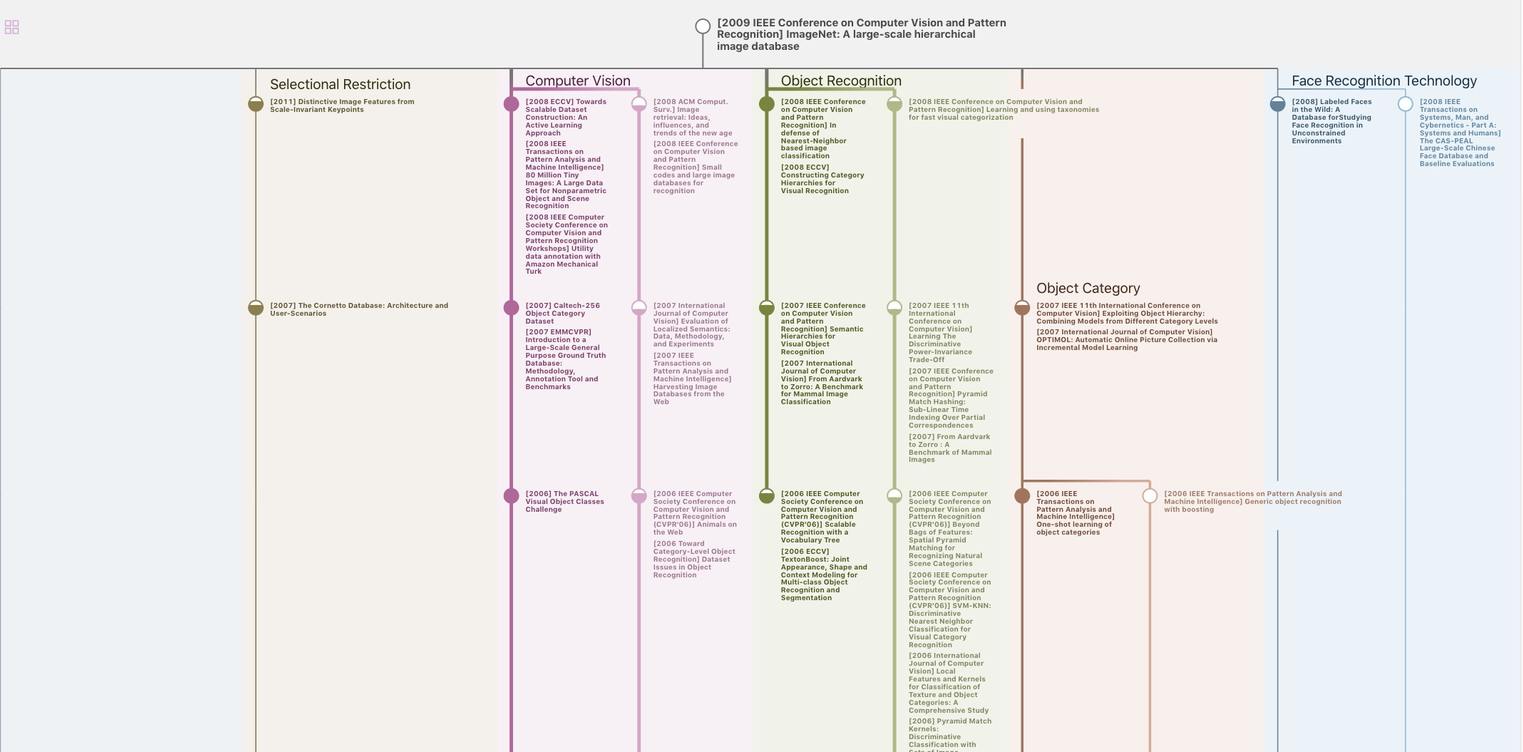
生成溯源树,研究论文发展脉络
Chat Paper
正在生成论文摘要