Differentiable Simulations for Enhanced Sampling of Rare Events
arXiv (Cornell University)(2023)
摘要
Simulating rare events, such as the transformation of a reactant into a product in a chemical reaction typically requires enhanced sampling techniques that rely on heuristically chosen collective variables (CVs). We propose using differentiable simulations (DiffSim) for the discovery and enhanced sampling of chemical transformations without a need to resort to preselected CVs, using only a distance metric. Reaction path discovery and estimation of the biasing potential that enhances the sampling are merged into a single end-to-end problem that is solved by path-integral optimization. This is achieved by introducing multiple improvements over standard DiffSim such as partial backpropagation and graph mini-batching making DiffSim training stable and efficient. The potential of DiffSim is demonstrated in the successful discovery of transition paths for the Muller-Brown model potential as well as a benchmark chemical system - alanine dipeptide.
更多查看译文
关键词
enhanced sampling,differentiable simulations,events
AI 理解论文
溯源树
样例
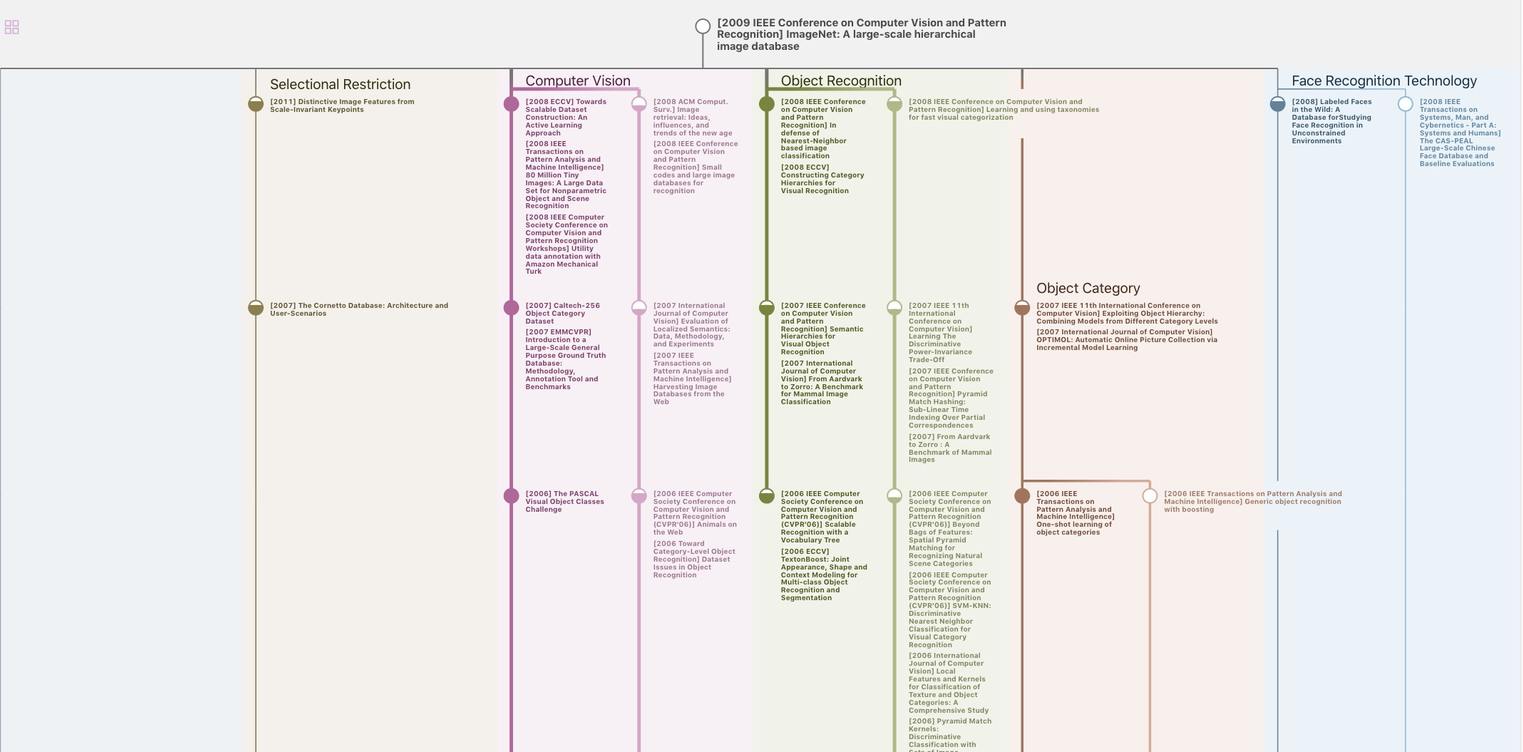
生成溯源树,研究论文发展脉络
Chat Paper
正在生成论文摘要