ERNIE 3.0 Tiny: Frustratingly Simple Method to Improve Task-Agnostic Distillation Generalization
arxiv(2023)
摘要
Task-agnostic knowledge distillation attempts to address the problem of deploying large pretrained language model in resource-constrained scenarios by compressing a large pretrained model called teacher into a smaller one called student such that the student can be directly finetuned on downstream tasks and retains comparable performance. However, we empirically find that there is a generalization gap between the student and the teacher in existing methods. In this work, we show that we can leverage multi-task learning in task-agnostic distillation to advance the generalization of the resulted student. In particular, we propose Multi-task Infused Task-agnostic Knowledge Distillation (MITKD). We first enhance the teacher by multi-task training it on multiple downstream tasks and then perform distillation to produce the student. Experimental results demonstrate that our method yields a student with much better generalization, significantly outperforms existing baselines, and establishes a new state-of-the-art result on in-domain, out-domain, and low-resource datasets in the setting of task-agnostic distillation. Moreover, our method even exceeds an 8x larger BERT$_{\text{Base}}$ on SQuAD and four GLUE tasks. In addition, by combining ERNIE 3.0, our method achieves state-of-the-art results on 10 Chinese datasets.
更多查看译文
关键词
distillation generalization,task-agnostic
AI 理解论文
溯源树
样例
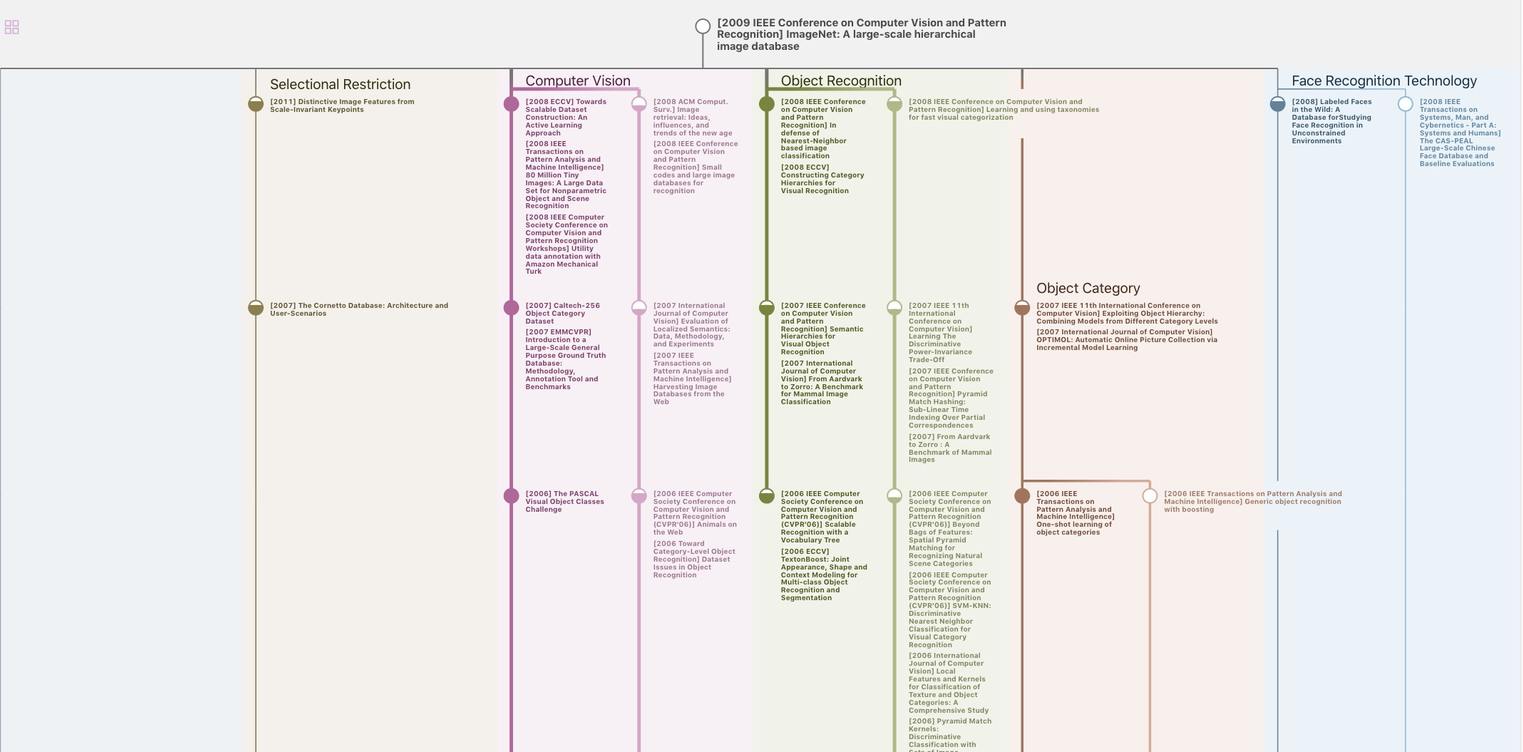
生成溯源树,研究论文发展脉络
Chat Paper
正在生成论文摘要