Noise-Net: Determining physical properties of HII regions reflecting observational uncertainties
arxiv(2023)
摘要
Stellar feedback, the energetic interaction between young stars and their birthplace, plays an important role in the star formation history of the universe and the evolution of the interstellar medium (ISM). Correctly interpreting the observations of star-forming regions is essential to understand stellar feedback, but it is a non-trivial task due to the complexity of the feedback processes and degeneracy in observations. In our recent paper, we introduced a conditional invertible neural network (cINN) that predicts seven physical properties of star-forming regions from the luminosity of 12 optical emission lines as a novel method to analyze degenerate observations. We demonstrated that our network, trained on synthetic star-forming region models produced by the WARPFIELD-Emission predictor (WARPFIELD-EMP), could predict physical properties accurately and precisely. In this paper, we present a new updated version of the cINN that takes into account the observational uncertainties during network training. Our new network named Noise-Net reflects the influence of the uncertainty on the parameter prediction by using both emission-line luminosity and corresponding uncertainties as the necessary input information of the network. We examine the performance of the Noise-Net as a function of the uncertainty and compare it with the previous version of the cINN, which does not learn uncertainties during the training. We confirm that the Noise-Net outperforms the previous network for the typical observational uncertainty range and maintains high accuracy even when subject to large uncertainties.
更多查看译文
关键词
methods: statistical,ISM: clouds,H II regions,galaxies: star formation
AI 理解论文
溯源树
样例
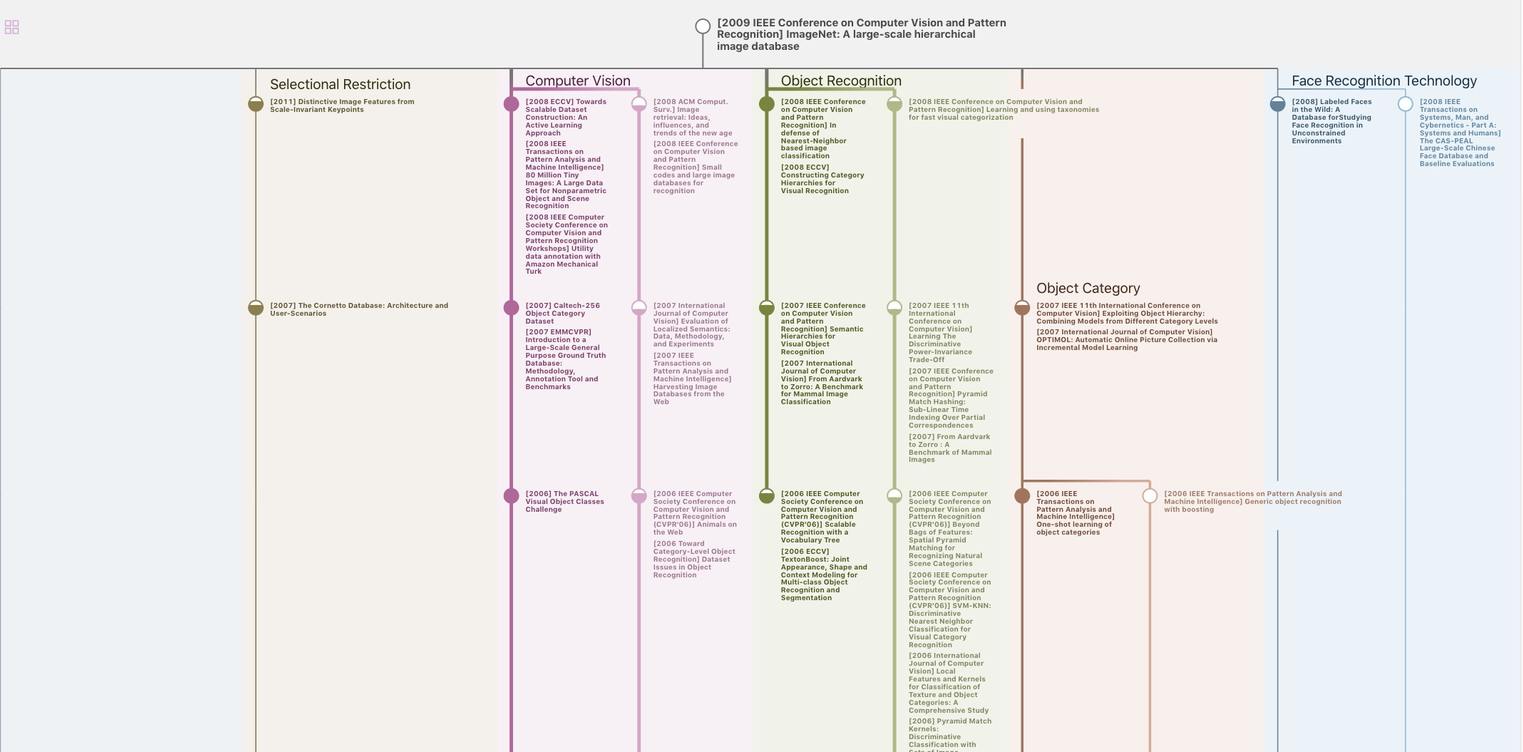
生成溯源树,研究论文发展脉络
Chat Paper
正在生成论文摘要