Unsupervised ensemble-based phenotyping helps enhance the discoverability of genes related to heart morphology
arxiv(2023)
摘要
Recent genome-wide association studies (GWAS) have been successful in identifying associations between genetic variants and simple cardiac parameters derived from cardiac magnetic resonance (CMR) images. However, the emergence of big databases including genetic data linked to CMR, facilitates investigation of more nuanced patterns of shape variability. Here, we propose a new framework for gene discovery entitled Unsupervised Phenotype Ensembles (UPE). UPE builds a redundant yet highly expressive representation by pooling a set of phenotypes learned in an unsupervised manner, using deep learning models trained with different hyperparameters. These phenotypes are then analyzed via (GWAS), retaining only highly confident and stable associations across the ensemble. We apply our approach to the UK Biobank database to extract left-ventricular (LV) geometric features from image-derived three-dimensional meshes. We demonstrate that our approach greatly improves the discoverability of genes influencing LV shape, identifying 11 loci with study-wide significance and 8 with suggestive significance. We argue that our approach would enable more extensive discovery of gene associations with image-derived phenotypes for other organs or image modalities.
更多查看译文
关键词
phenotyping,heart,genes,ensemble-based
AI 理解论文
溯源树
样例
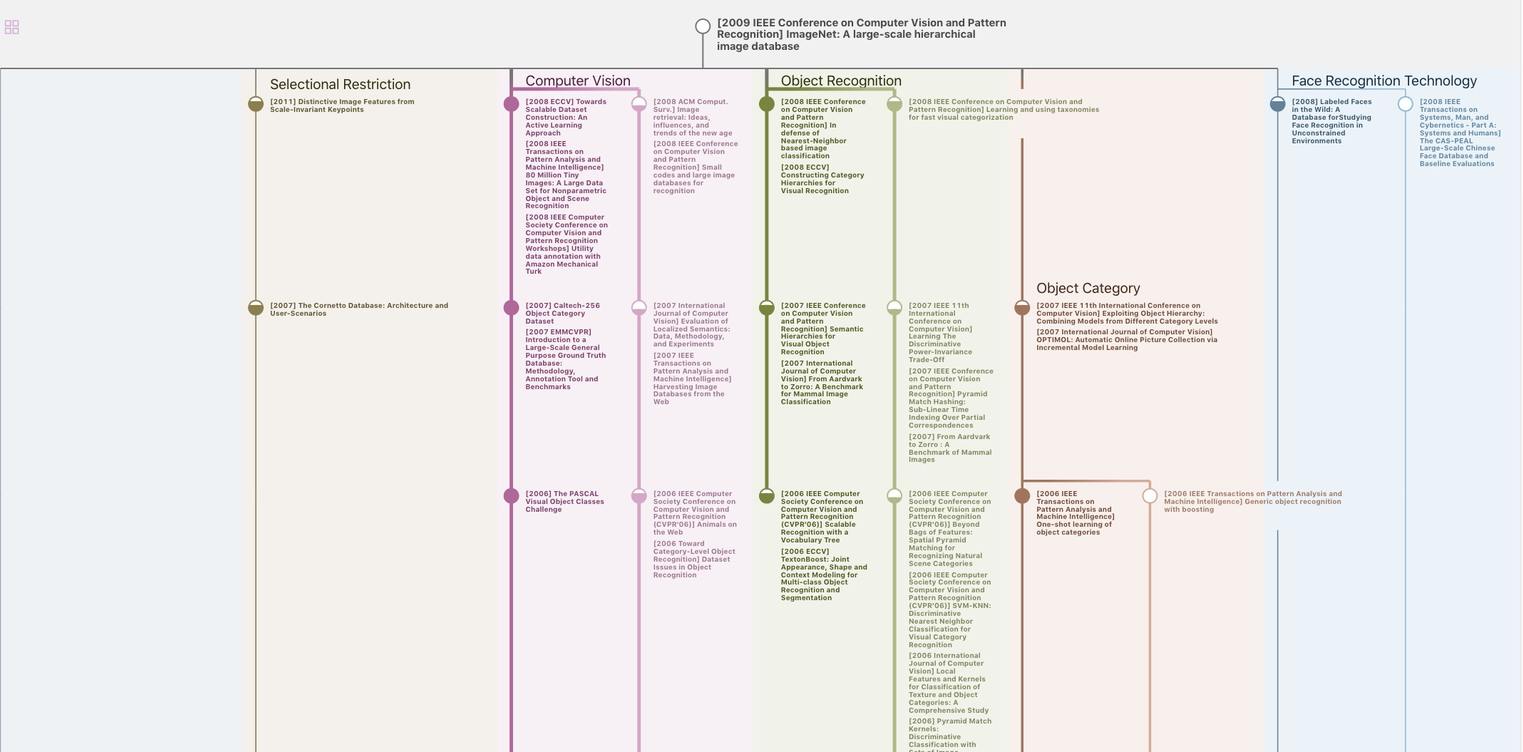
生成溯源树,研究论文发展脉络
Chat Paper
正在生成论文摘要