Active Deep Learning Guided by Efficient Gaussian Process Surrogates
arxiv(2023)
摘要
The success of active learning relies on the exploration of the underlying data-generating distributions, populating sparsely labeled data areas, and exploitation of the information about the task gained by the baseline (neural network) learners. In this paper, we present a new algorithm that combines these two active learning modes. Our algorithm adopts a Bayesian surrogate for the baseline learner, and it optimizes the exploration process by maximizing the gain of information caused by new labels. Further, by instantly updating the surrogate learner for each new data instance, our model can faithfully simulate and exploit the continuous learning behavior of the learner without having to actually retrain it per label. In experiments with four benchmark classification datasets, our method demonstrated significant performance gain over state-of-the-arts.
更多查看译文
关键词
efficient gaussian process surrogates,deep learning,active
AI 理解论文
溯源树
样例
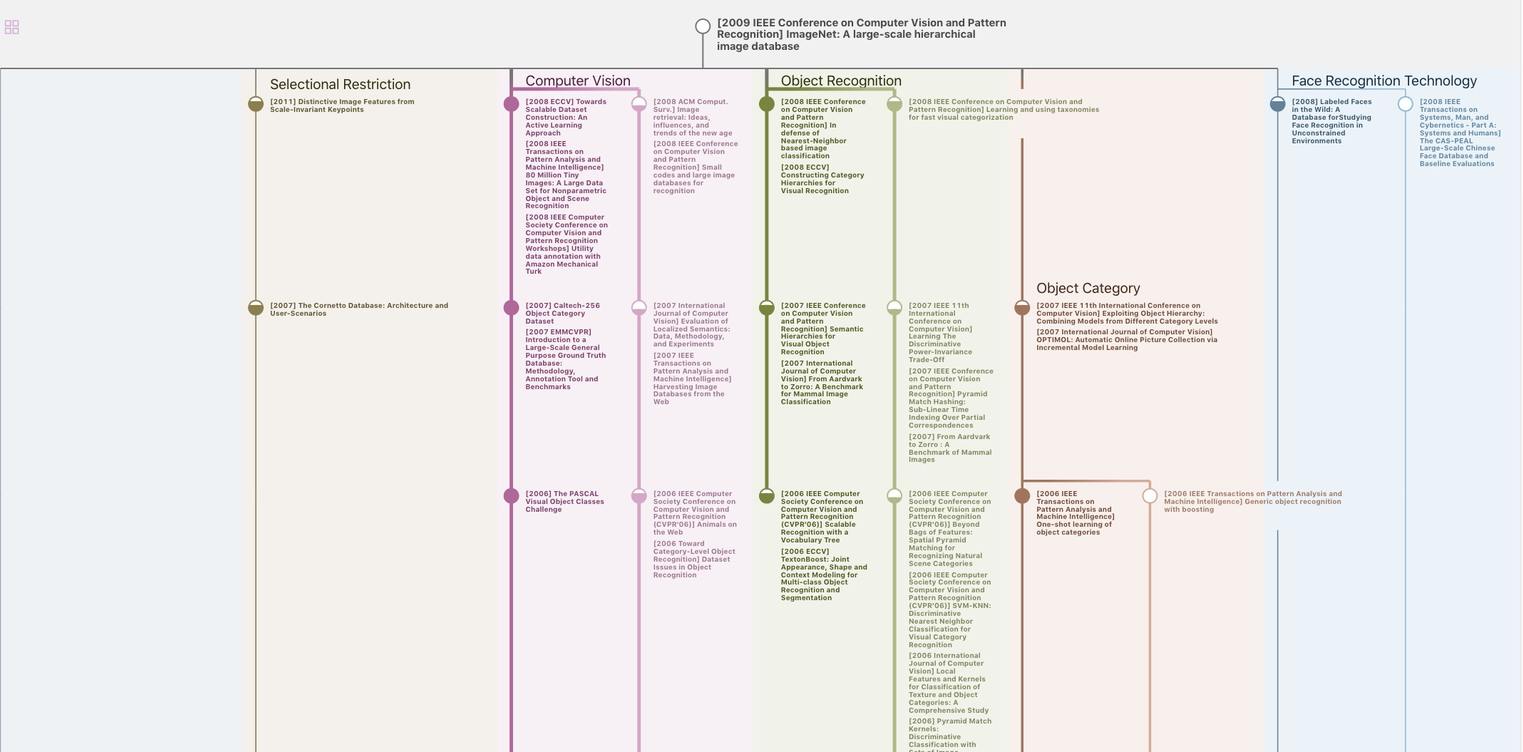
生成溯源树,研究论文发展脉络
Chat Paper
正在生成论文摘要