MAV Localization in Large-Scale Environments: A Decoupled Optimization/Filtering Approach.
Sensors(2023)
摘要
Developing new sensor fusion algorithms has become indispensable to tackle the daunting problem of GPS-aided micro aerial vehicle (MAV) localization in large-scale landscapes. Sensor fusion should guarantee high-accuracy estimation with the least amount of system delay. Towards this goal, we propose a linear optimal state estimation approach for the MAV to avoid complicated and high-latency calculations and an immediate metric-scale recovery paradigm that uses low-rate noisy GPS measurements when available. Our proposed strategy shows how the vision sensor can quickly bootstrap a pose that has been arbitrarily scaled and recovered from various drifts that affect vision-based algorithms. We can consider the camera as a "black-box" pose estimator thanks to our proposed optimization/filtering-based methodology. This maintains the sensor fusion algorithm's computational complexity and makes it suitable for MAV's long-term operations in expansive areas. Due to the limited global tracking and localization data from the GPS sensors, our proposal on MAV's localization solution considers the sensor measurement uncertainty constraints under such circumstances. Extensive quantitative and qualitative analyses utilizing real-world and large-scale MAV sequences demonstrate the higher performance of our technique in comparison to most recent state-of-the-art algorithms in terms of trajectory estimation accuracy and system latency.
更多查看译文
关键词
Kalman filter,MAV,calibration,localization,multimodal sensing,odometry,optimization,sensor fusion,visual drifts
AI 理解论文
溯源树
样例
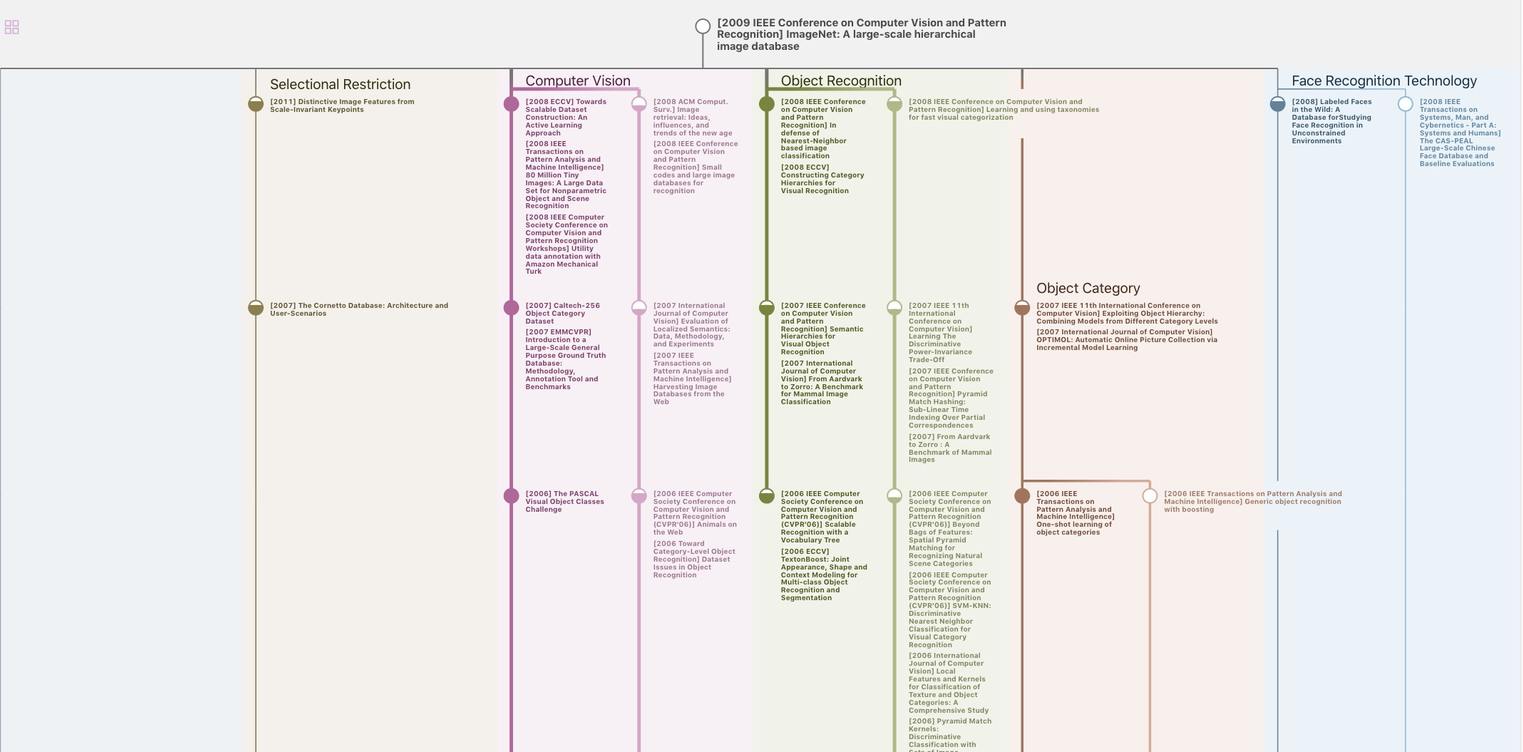
生成溯源树,研究论文发展脉络
Chat Paper
正在生成论文摘要