Prediction of extubation failure among low birthweight neonates using machine learning
Journal of perinatology : official journal of the California Perinatal Association(2023)
摘要
Objective To develop machine learning models predicting extubation failure in low birthweight neonates using large amounts of clinical data. Study design Retrospective cohort study using MIMIC-III, a large single-center, open-source clinical dataset. Logistic regression and boosted-tree (XGBoost) models using demographics, medications, and vital sign and ventilatory data were developed to predict extubation failure, defined as reintubation within 7 days. Results 1348 low birthweight (≤2500 g) neonates who received mechanical ventilation within the first 7 days were included, of which 350 (26%) failed a trial of extubation. The best-performing model was a boosted-tree model incorporating demographics, vital signs, ventilator parameters, and medications (AUROC 0.82). The most important features were birthweight, last FiO 2 , average mean airway pressure, caffeine use, and gestational age. Conclusions Machine learning models identified low birthweight ventilated neonates at risk for extubation failure. These models will need to be validated across multiple centers to determine generalizability of this tool.
更多查看译文
关键词
Epidemiology,Paediatrics,Respiratory tract diseases,Medicine/Public Health,general,Pediatrics,Pediatric Surgery
AI 理解论文
溯源树
样例
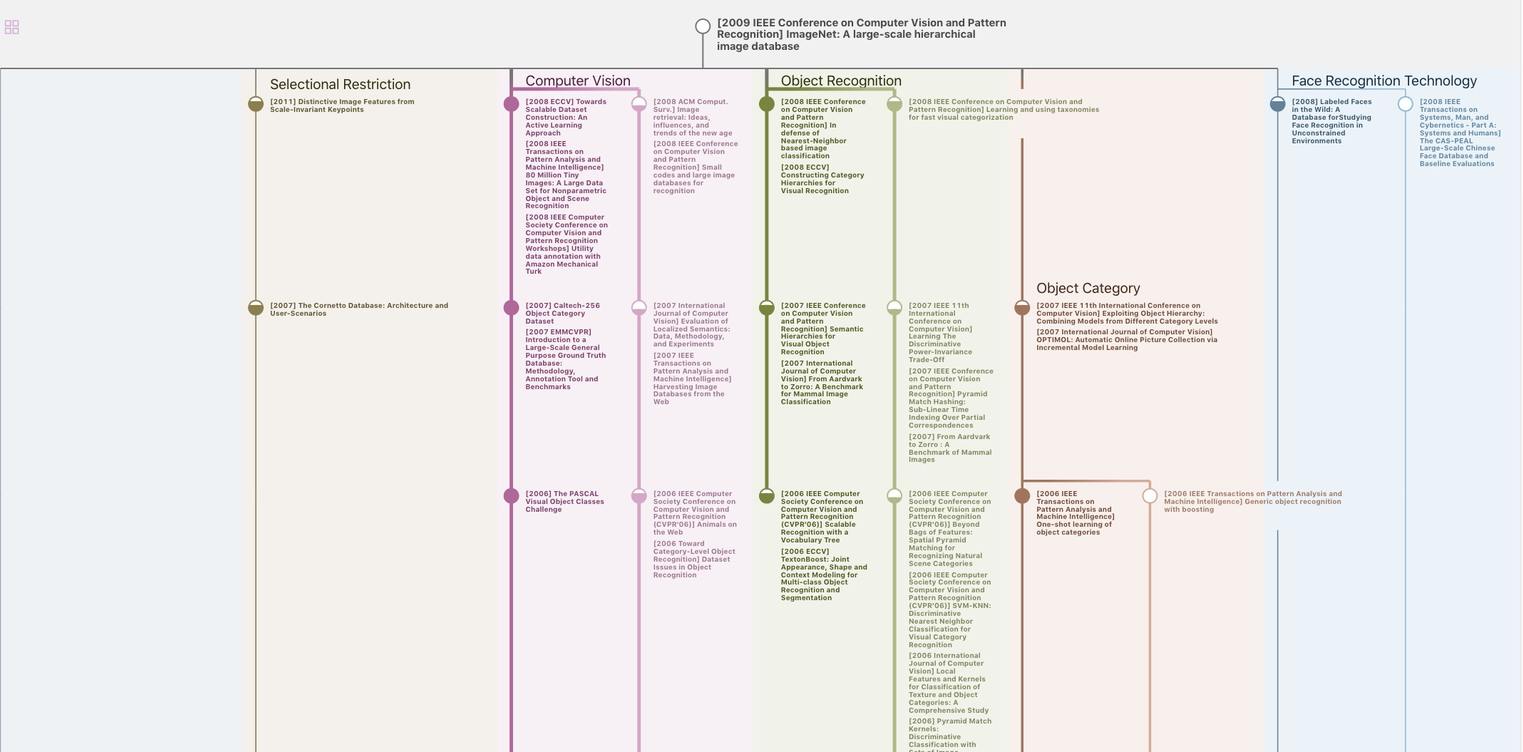
生成溯源树,研究论文发展脉络
Chat Paper
正在生成论文摘要