Utilizing machine learning and electronic medical records to predict postpartum preeclampsia
American Journal of Obstetrics and Gynecology(2023)
摘要
To improve preeclampsia risk prediction beyond standard guidelines, we aimed to create machine learning models using large-scale electronic health record (EHR) data to identify high-risk patients. Additionally, we sought to determine which factors were most influential in determining risk. A multi-center analysis of patients who received prenatal care from January 2017 to June 2022 was carried out. The data were sourced from the EHR system and data collected during the first sixteen weeks of pregnancy were used for the prediction models. The algorithms to predict preeclampsia (with and without severe features) are trained using LightGBM, a high-performance implementation of gradient boosted tree models in both weighted and unweighted versions. A total of 121,662 patients were used to train the algorithm and 6.3% were diagnosed with preeclampsia during their pregnancy. Our models have a mean area under the curve (AUC) of 0.743-0.744 for preeclampsia (95% CI: 0.74-0.77). The post-test likelihood ratio is 10 to 15 using our models. The negative predictive value is 93.9%-94.3% with a positive predictive value of 39.2-62.9% (Table 1). The factors most predictive of preeclampsia, in order of importance include number of prior term deliveries, body mass index (BMI), ZIP code, parity, chronic hypertension, maternal age, pre-pregnancy BMI, race, and alcohol use. Our model has predictive accuracy above that which has been previously reported and above that which is currently utilized (Figure 1). The 10 to 15-fold increase in likelihood of a patient developing preeclampsia after screening positive on our model highlights the potential diagnostic value of implementing this predictive model. It could be applied to personalize risk assessment following a routine first prenatal office visit, without additional workup. Utilizing this model could allow initiation of aspirin prophylaxis in a more precise patient population and targeting of high-risk patients with appropriate antepartum monitoring.View Large Image Figure ViewerDownload Hi-res image Download (PPT)
更多查看译文
关键词
electronic medical records,machine learning
AI 理解论文
溯源树
样例
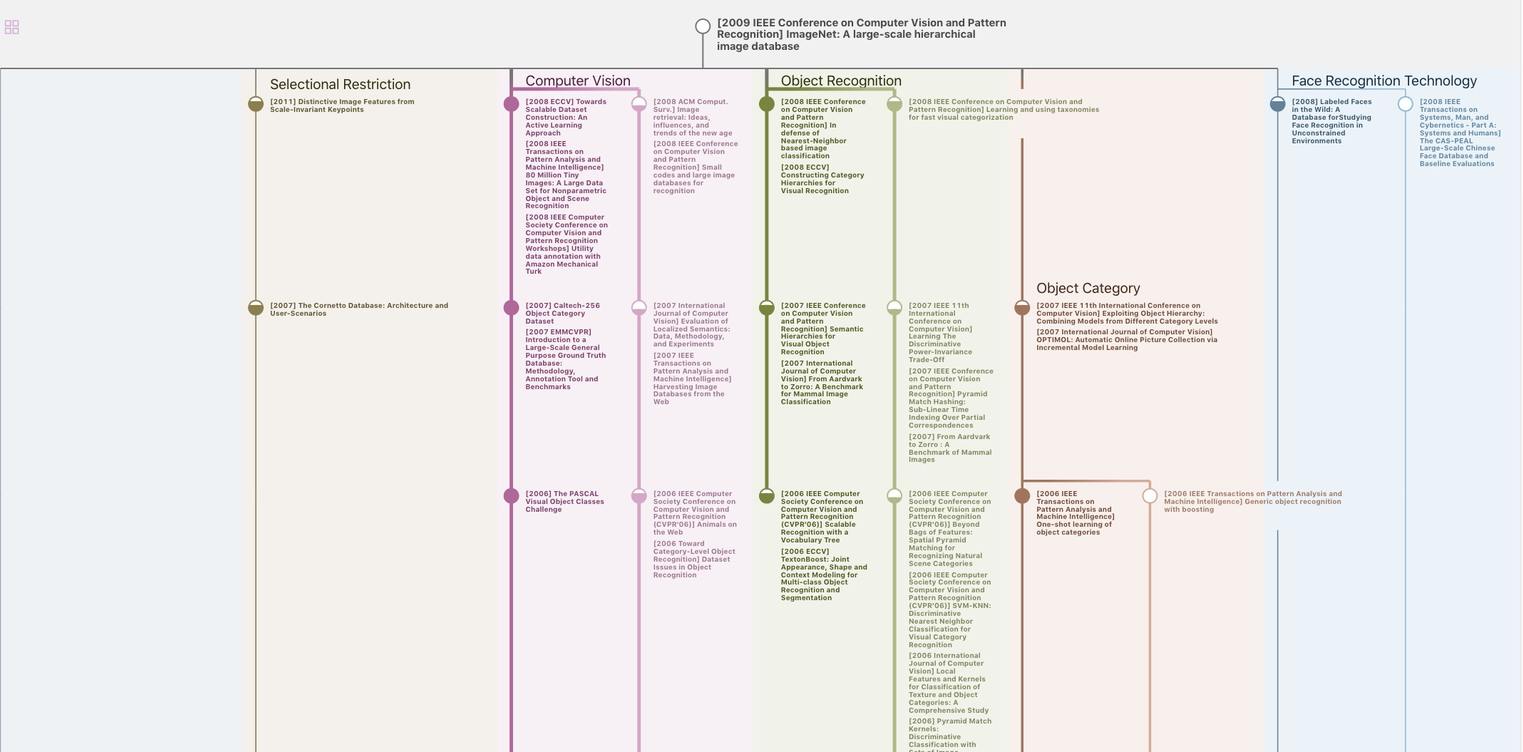
生成溯源树,研究论文发展脉络
Chat Paper
正在生成论文摘要