Model-Agnostic Hierarchical Attention for 3D Object Detection
arxiv(2023)
摘要
Transformers as versatile network architectures have recently seen great success in 3D point cloud object detection. However, the lack of hierarchy in a plain transformer makes it difficult to learn features at different scales and restrains its ability to extract localized features. Such limitation makes them have imbalanced performance on objects of different sizes, with inferior performance on smaller ones. In this work, we propose two novel attention mechanisms as modularized hierarchical designs for transformer-based 3D detectors. To enable feature learning at different scales, we propose Simple Multi-Scale Attention that builds multi-scale tokens from a single-scale input feature. For localized feature aggregation, we propose Size-Adaptive Local Attention with adaptive attention ranges for every bounding box proposal. Both of our attention modules are model-agnostic network layers that can be plugged into existing point cloud transformers for end-to-end training. We evaluate our method on two widely used indoor 3D point cloud object detection benchmarks. By plugging our proposed modules into the state-of-the-art transformer-based 3D detector, we improve the previous best results on both benchmarks, with the largest improvement margin on small objects.
更多查看译文
关键词
3d object detection,object detection,attention,model-agnostic
AI 理解论文
溯源树
样例
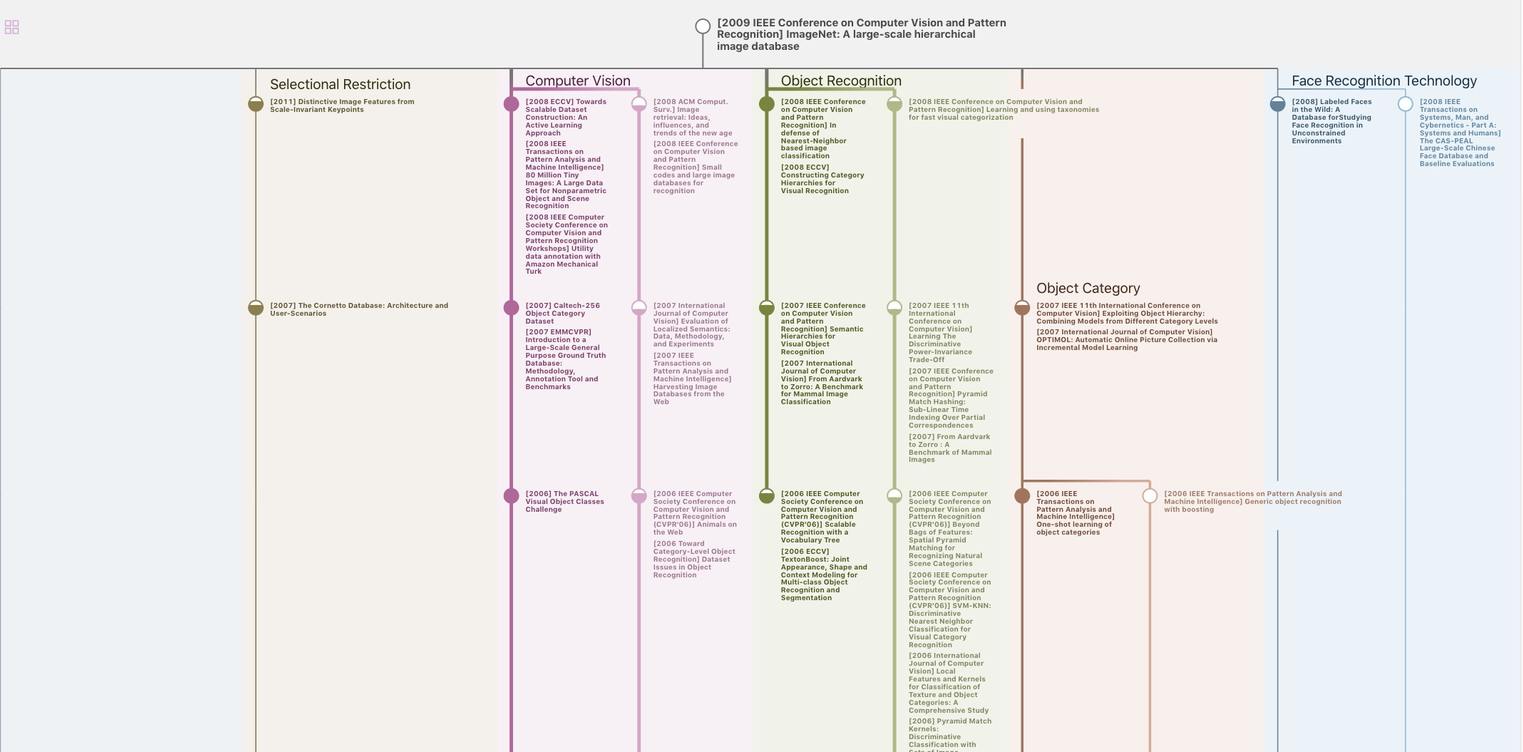
生成溯源树,研究论文发展脉络
Chat Paper
正在生成论文摘要