A drive-by bridge inspection framework using non-parametric clusters over projected data manifolds
Mechanical Systems and Signal Processing(2022)
摘要
Developing robust bridge health monitoring (BHM) frameworks based on the vehicle-mounted sensing, or so-called indirect structural health monitoring (SHM) or Drive-by Bridge Inspection is currently a rapidly growing research area. The goal is to monitor any change in the structural integrity of a bridge using the vibration responses measured from sensors installed on a vehicle passing over the bridge. To this aim, we present a novel data-driven approach by integrating a nonlinear dimensionality-reduction technique using Uniform Manifold Approximation and Projection (UMAP) together with a non-parametric clustering technique using Hierarchical Density-Based Spatial Clustering of Applications with Noise (HDBSCAN). Ultimately, through the combined use of UMAP and HDBSCAN, it is demonstrated that such methods align perfectly well with the problems and restrictions present when analyzing drive-by bridge inspection data. That is, in the drive-by bridge inspection context data sets may have low cardinality, and the individual clusters may have different sizes and densities and do not need to have a spherical shape. Furthermore, new clusters may emerge over time as new damage cases evolve. The pairing of UMAP and HDBSCAN will be shown to assist in these issues in a principled manner. For the validation, we first make use of the theoretical formulation of the vehicle-bridge interaction system and conduct a finite element simulation of this system to obtain the moving vehicle response once traveling over five different bridge states. Further, a simply supported bridge model in the laboratory is considered for the experimental validation. The structure of the bridge model is gradually changed by adding multiple stiffener plates, and by gradually increasing a concentrated mass at mid-span. The proposed method is then applied to identify changes that occur in the laboratory bridge model. The experimental results for damage characterization demonstrate that various bridge states can successfully be separated from one another. The contributions of the work are three-fold; (1) First, a novel feature extraction and classification framework based on the combined use of UMAP and HDBSCAN is developed and validated. (2) Second, this paper demonstrates one of the early successful attempts of drive-by bridge inspection for monitoring the progressive change in the structure of a bridge based on the extensive numerical and experimental investigations. (3) Finally, the research presented in this work can open up new opportunities for condition monitoring of bridge network.
更多查看译文
关键词
Indirect SHM,Damage identification,Vehicle bridge interaction (VBI),Dimensionality reduction,Clustering,UMAP,HDBSCAN
AI 理解论文
溯源树
样例
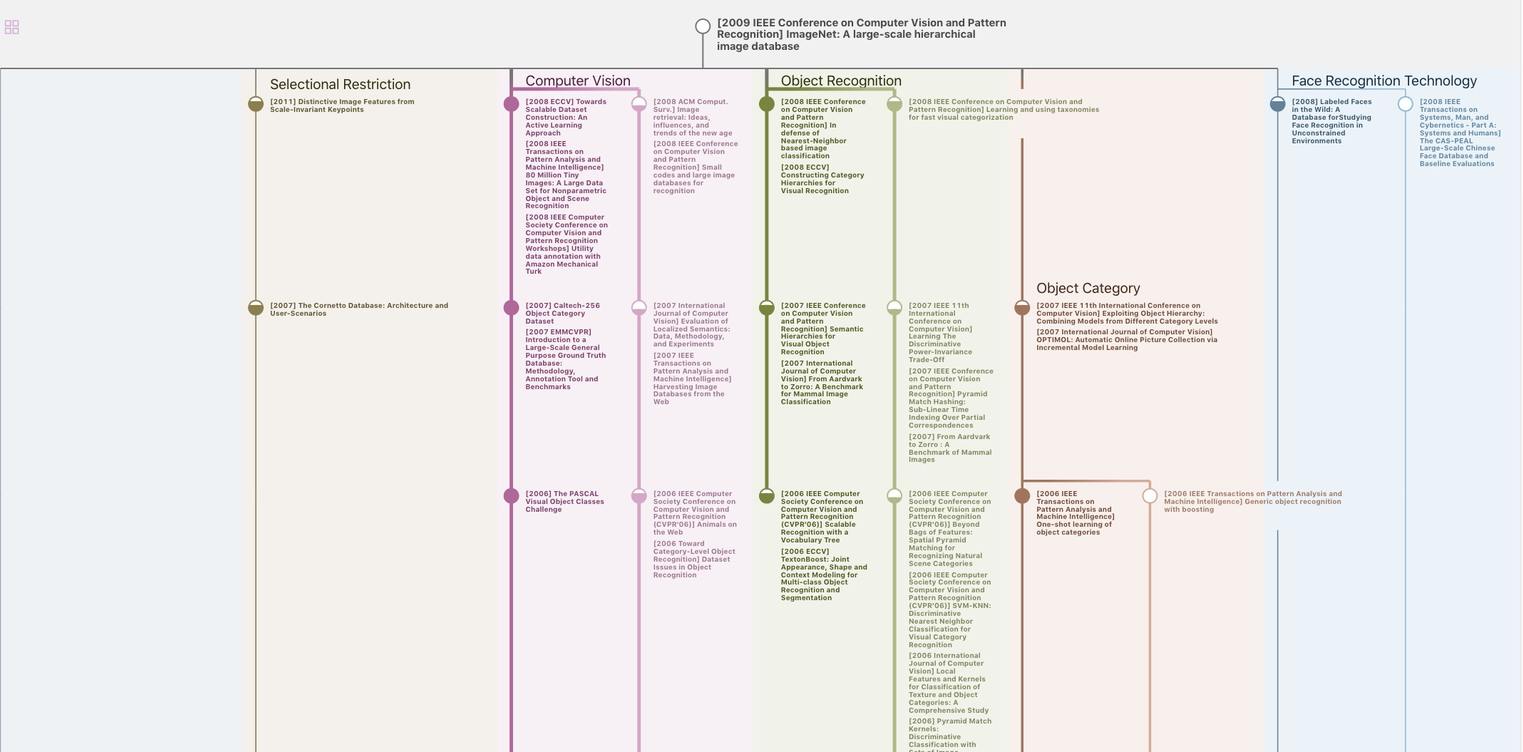
生成溯源树,研究论文发展脉络
Chat Paper
正在生成论文摘要