Vital sign-based detection of sepsis in neonates using machine learning.
Acta paediatrica (Oslo, Norway : 1992)(2023)
摘要
AIM:Sepsis is a leading cause of morbidity and mortality in neonates. Early diagnosis is key but difficult due to non-specific signs. We investigate the predictive value of machine learning-assisted analysis of non-invasive, high frequency monitoring data and demographic factors to detect neonatal sepsis.
METHODS:Single centre study, including a representative cohort of 325 infants (2866 hospitalisation days). Personalised event timelines including interventions and clinical findings were generated. Time-domain features from heart rate, respiratory rate and oxygen saturation values were calculated and demographic factors included. Sepsis prediction was performed using Naïve Bayes algorithm in a maximum a posteriori framework up to 24 h before clinical sepsis suspicion.
RESULTS:Twenty sepsis cases were identified. Combining multiple vital signs improved algorithm performance compared to heart rate characteristics alone. This enabled a prediction of sepsis with an area under the receiver operating characteristics curve of 0.82, up to 24 h before clinical sepsis suspicion. Moreover, 10 h prior to clinical suspicion, the risk of sepsis increased 150-fold.
CONCLUSION:The present algorithm using non-invasive patient data provides useful predictive value for neonatal sepsis detection. Machine learning-assisted algorithms are promising novel methods that could help individualise patient care and reduce morbidity and mortality.
更多查看译文
关键词
Naïve Bayes classifier,artificial intelligence,clinical decision support system,physiological monitoring,prediction,respiration-related
AI 理解论文
溯源树
样例
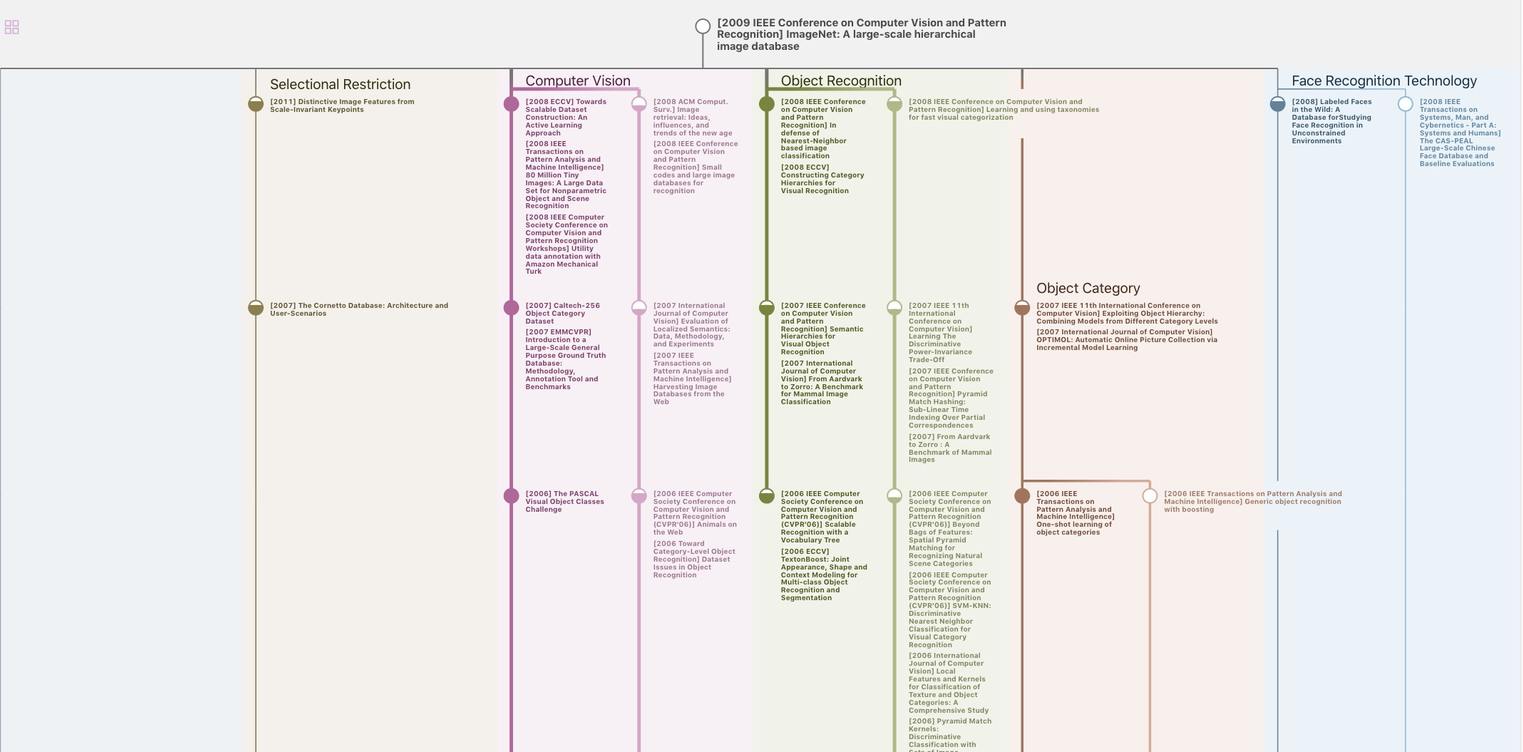
生成溯源树,研究论文发展脉络
Chat Paper
正在生成论文摘要