Profiling oocytes with neural networks from images and mechanical data
Journal of the Mechanical Behavior of Biomedical Materials(2023)
摘要
The success rate of assisted reproductive technologies could be greatly improved by selectively choosing egg cells (oocytes) with the greatest chance of fertilization. The goal of mechanical profiling is, thus, to improve predictive oocyte selection by isolating the mechanical properties of oocytes and correlating them to their reproductive potential. The restrictions on experimental platforms, however – including minimal invasiveness and practicality in laboratory implementation – greatly limits the data that can be acquired from a single oocyte. In this study, we perform indentation studies on human oocytes and characterize the mechanical properties of the zona pellucida, the outer layer of the oocyte. We obtain excellent fitting with our physical model when indenting with a flat surface and clearly illustrate localized shear-thinning behavior of the zona pellucida, which has not been previously reported. We conclude by outlining a promising methodology for isolating the mechanical properties of the cytoplasm using neural networks and optical images taken during indentation.
更多查看译文
关键词
Inverse problems,Neural networks,Mechanical characterization,Transient Network Theory,Mechanics
AI 理解论文
溯源树
样例
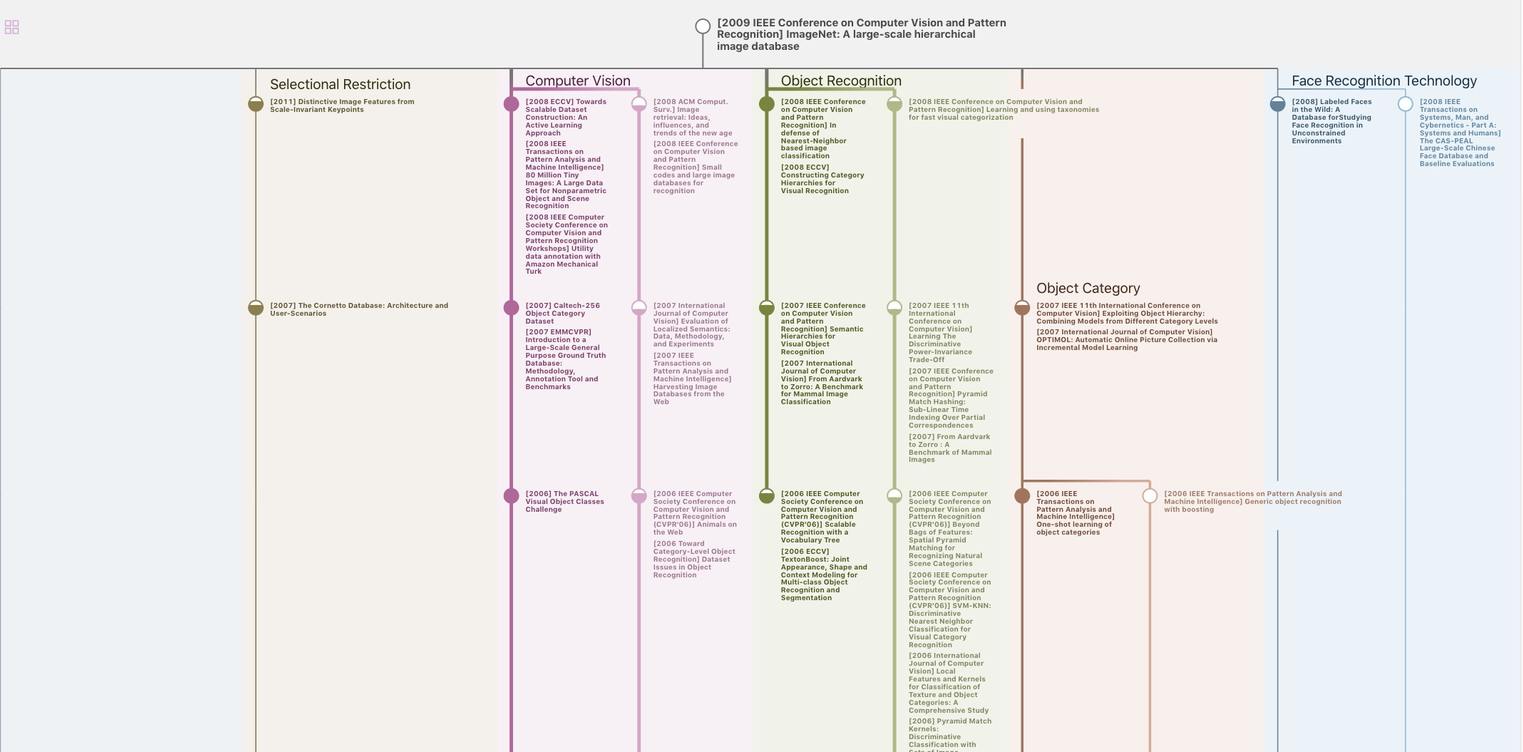
生成溯源树,研究论文发展脉络
Chat Paper
正在生成论文摘要