Urban road traffic flow prediction: A graph convolutional network embedded with wavelet decomposition and attention mechanism
Physica A: Statistical Mechanics and its Applications(2022)
摘要
Urban road traffic flow prediction is the key basis for the development of Intelligent Transportation System. The complex urban structure leads to irregular shape and layout of the road network, which poses a challenge to capture the spatio-temporal correlation of traffic flow at different nodes in the region. In this study, a graph convolutional network model framework embedded with wavelet decomposition and attention mechanism (WDA-GCN) is proposed to predict the traffic flow of each traffic monitor at the regional level by exploring the spatio-temporal correlation among traffic monitors. Specifically, the spatial correlation between different monitors is encoded into two graphs by Graph Convolutional Network (GCN): geographical neighbor graph and functional similarity graph. The Gated Recurrent Unit (GRU) is used to learn the spatial features extracted by GCN, and the attention mechanism is added to improve the prediction accuracy. Finally, the time series data and spatio-temporal correlation of traffic flow are input into the encoder–decoder based on GRU to realize regional traffic flow prediction. The model is validated and compared with the real traffic monitor data in Daxing District of Beijing, China, and the results show that the prediction accuracy of WDA-GCN model can reach 81.03% after embedding wavelet decomposition and attention mechanism, which is better than the traditional time series prediction methods and deep learning methods.
更多查看译文
关键词
Traffic flow prediction,Graph convolutional network,Gated recurrent unit,Deep learning
AI 理解论文
溯源树
样例
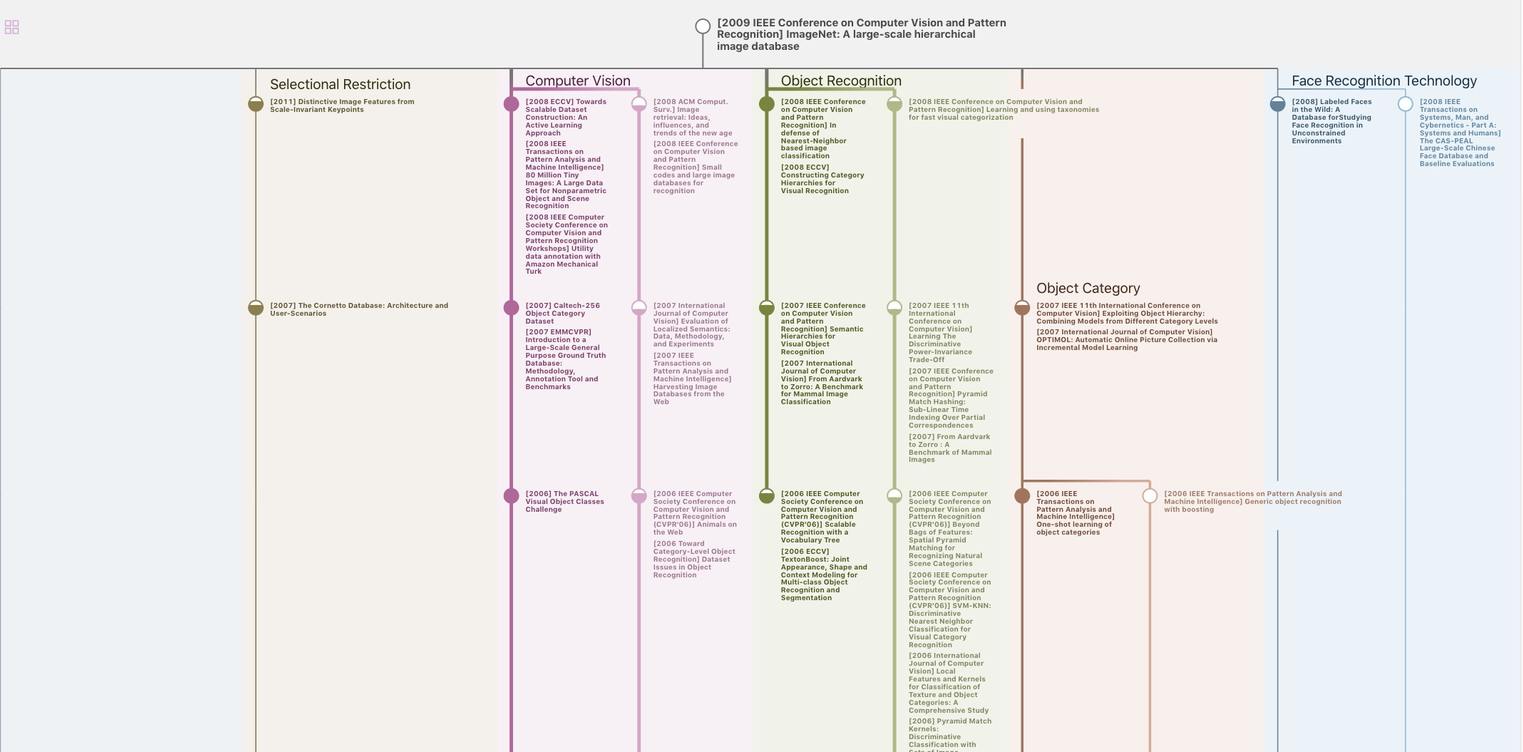
生成溯源树,研究论文发展脉络
Chat Paper
正在生成论文摘要