Inferring the interaction rules of complex systems with graph neural networks and approximate Bayesian computation.
Journal of the Royal Society, Interface(2023)
摘要
Inferring the underlying processes that drive collective behaviour in biological and social systems is a significant statistical and computational challenge. While simulation models have been successful in qualitatively capturing many of the phenomena observed in these systems in a variety of domains, formally fitting these models to data remains intractable. Recently, approximate Bayesian computation (ABC) has been shown to be an effective approach to inference if the likelihood function for a model is unavailable. However, a key difficulty in successfully implementing ABC lies with the design, selection and weighting of appropriate summary statistics, a challenge that is especially acute when modelling high dimensional complex systems. In this work, we combine a Gaussian process accelerated ABC method with the automatic learning of summary statistics via graph neural networks. Our approach bypasses the need to design a model-specific set of summary statistics for inference. Instead, we encode relational inductive biases into a neural network using a graph embedding and then extract summary statistics automatically from simulation data. To evaluate our framework, we use a model of collective animal movement as a test bed and compare our method to a standard summary statistics approach and a linear regression-based algorithm.
更多查看译文
关键词
Bayesian inference,Gaussian processes,collective movement,emergent properties,machine learning
AI 理解论文
溯源树
样例
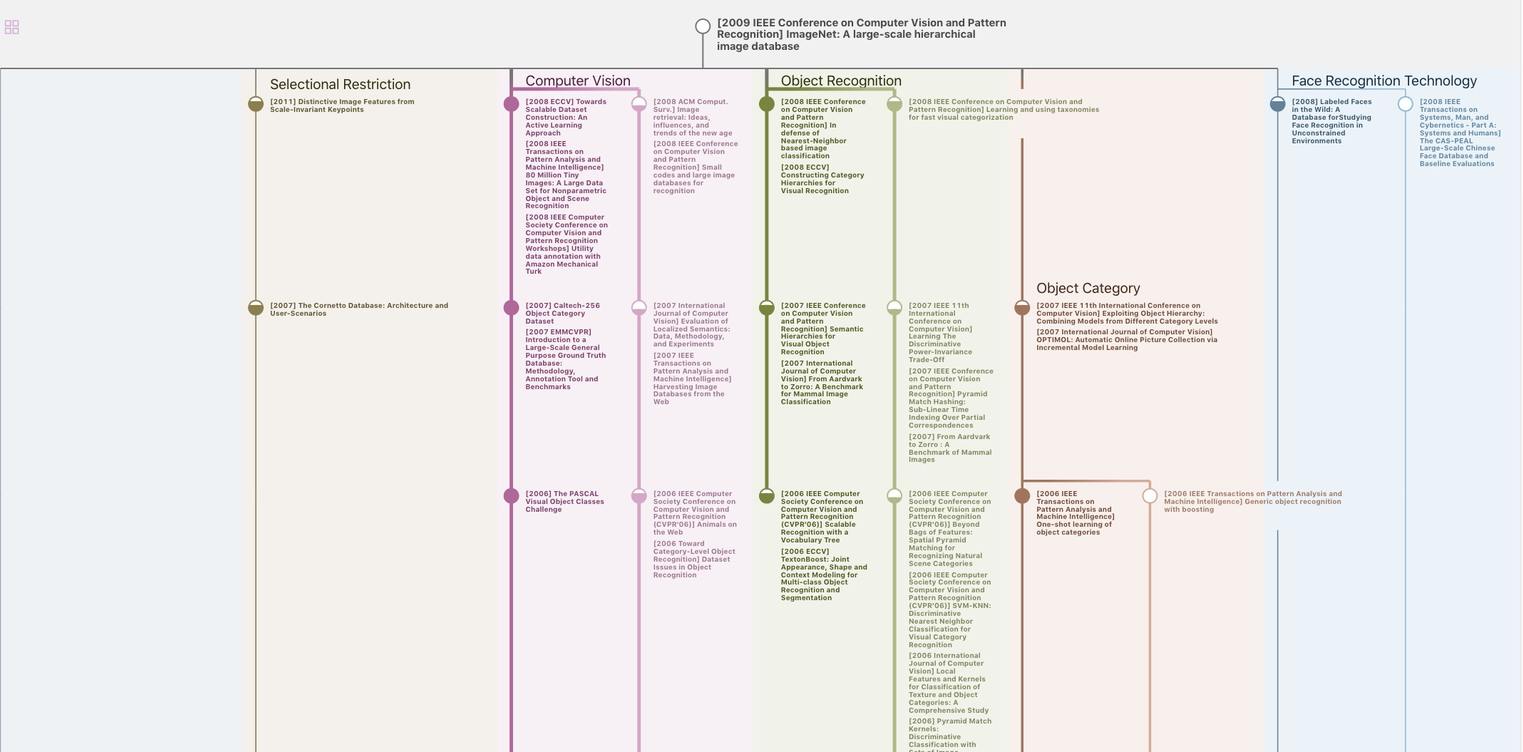
生成溯源树,研究论文发展脉络
Chat Paper
正在生成论文摘要