Accurate Segmentation Of Prostate Cancer Histomorphometric Features Using A Weakly Supervised Convolutional Neural Network
JOURNAL OF MEDICAL IMAGING(2020)
摘要
Purpose: Prostate cancer primarily arises from the glandular epithelium. Histomophometric techniques have been used to assess the glandular epithelium in automated detection and classification pipelines; however, they are often rigid in their implementation, and their performance suffers on large datasets where variation in staining, imaging, and preparation is difficult to control. The purpose of this study is to quantify performance of a pixelwise segmentation algorithm that was trained using different combinations of weak and strong stroma, epithelium, and lumen labels in a prostate histology dataset.Approach: We have combined weakly labeled datasets generated using simple morphometric techniques and high-quality labeled datasets from human observers in prostate biopsy cores to train a convolutional neural network for use in whole mount prostate labeling pipelines. With trained networks, we characterize pixelwise segmentation of stromal, epithelium, and lumen (SEL) regions on both biopsy core and whole-mount H&E-stained tissue.Results: We provide evidence that by simply training a deep learning algorithm on weakly labeled data generated from rigid morphometric methods, we can improve the robustness of classification over the morphometric methods used to train the classifier.Conclusions: We show that not only does our approach of combining weak and strong labels for training the CNN improve qualitative SEL labeling within tissue but also the deep learning generated labels are superior for cancer classification in a higher-order algorithm over the morphometrically derived labels it was trained on. (C) The Authors. Published by SPIE under a Creative Commons Attribution 4.0 Unported License.
更多查看译文
关键词
prostate cancer, deep learning, machine learning, histology, epithelium, segmentation
AI 理解论文
溯源树
样例
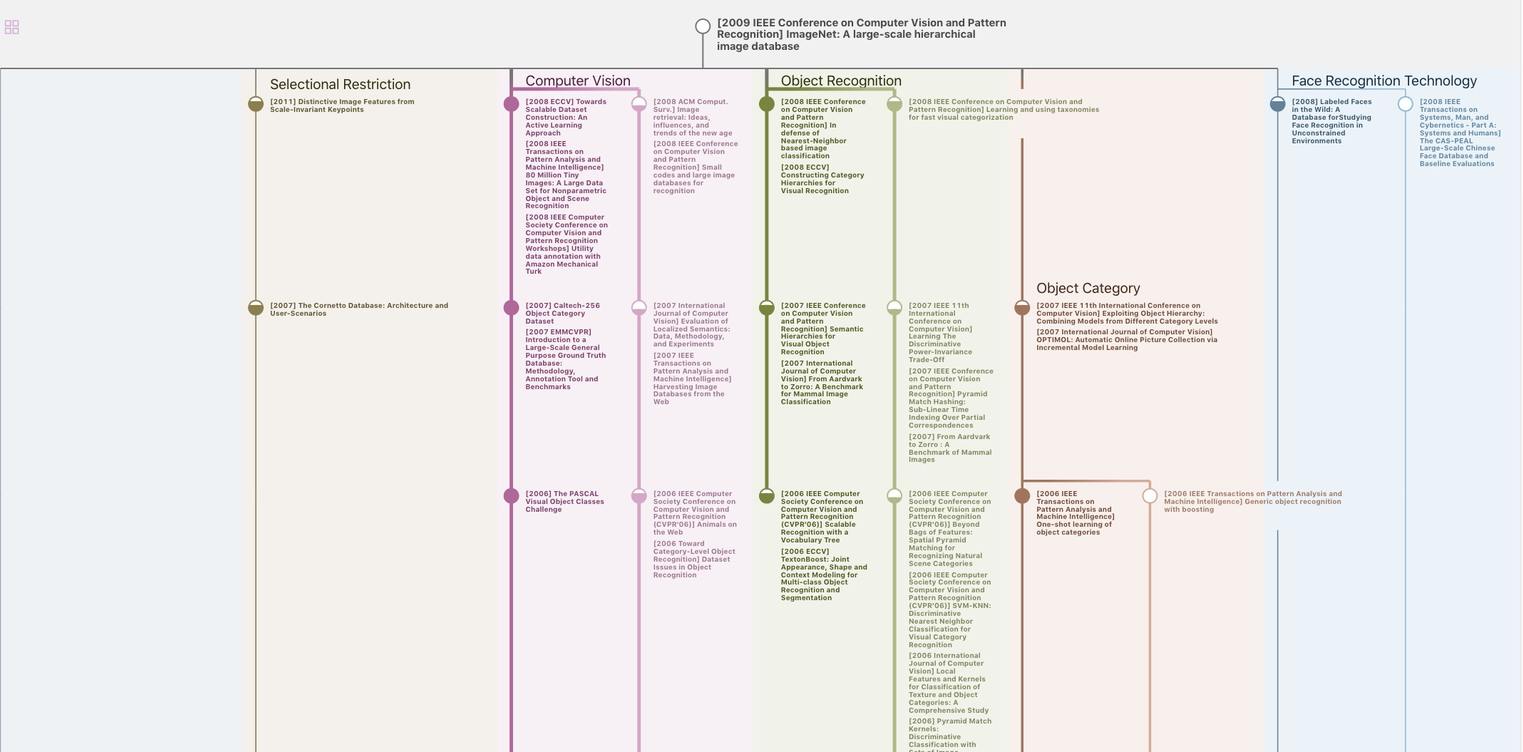
生成溯源树,研究论文发展脉络
Chat Paper
正在生成论文摘要