Generalization bounds for sparse random feature expansions ?
Applied and Computational Harmonic Analysis(2023)
摘要
Random feature methods have been successful in various machine learning tasks, are easy to compute, and come with theoretical accuracy bounds. They serve as an alternative approach to standard neural networks since they can represent similar function spaces without a costly training phase. However, for accuracy, random feature methods require more measurements than trainable parameters, limiting their use for data-scarce applications. We introduce the sparse random feature expansion to obtain parsimonious random feature models. We leverage ideas from compressive sensing to generate random feature expansions with theoretical guarantees even in the data-scarce setting. We provide generalization bounds for functions in a certain class depending on the number of samples and the distribution of features. By introducing sparse features, i.e. features with random sparse weights, we provide improved bounds for low order functions. We show that our method outperforms shallow networks in several scientific machine learning tasks. (c) 2022 The Author(s). Published by Elsevier Inc. This is an open access article under the CC BY license (http://creativecommons.org/licenses/by/4.0/).
更多查看译文
关键词
Random features,Sparse optimization,Generalization error,Compressive sensing
AI 理解论文
溯源树
样例
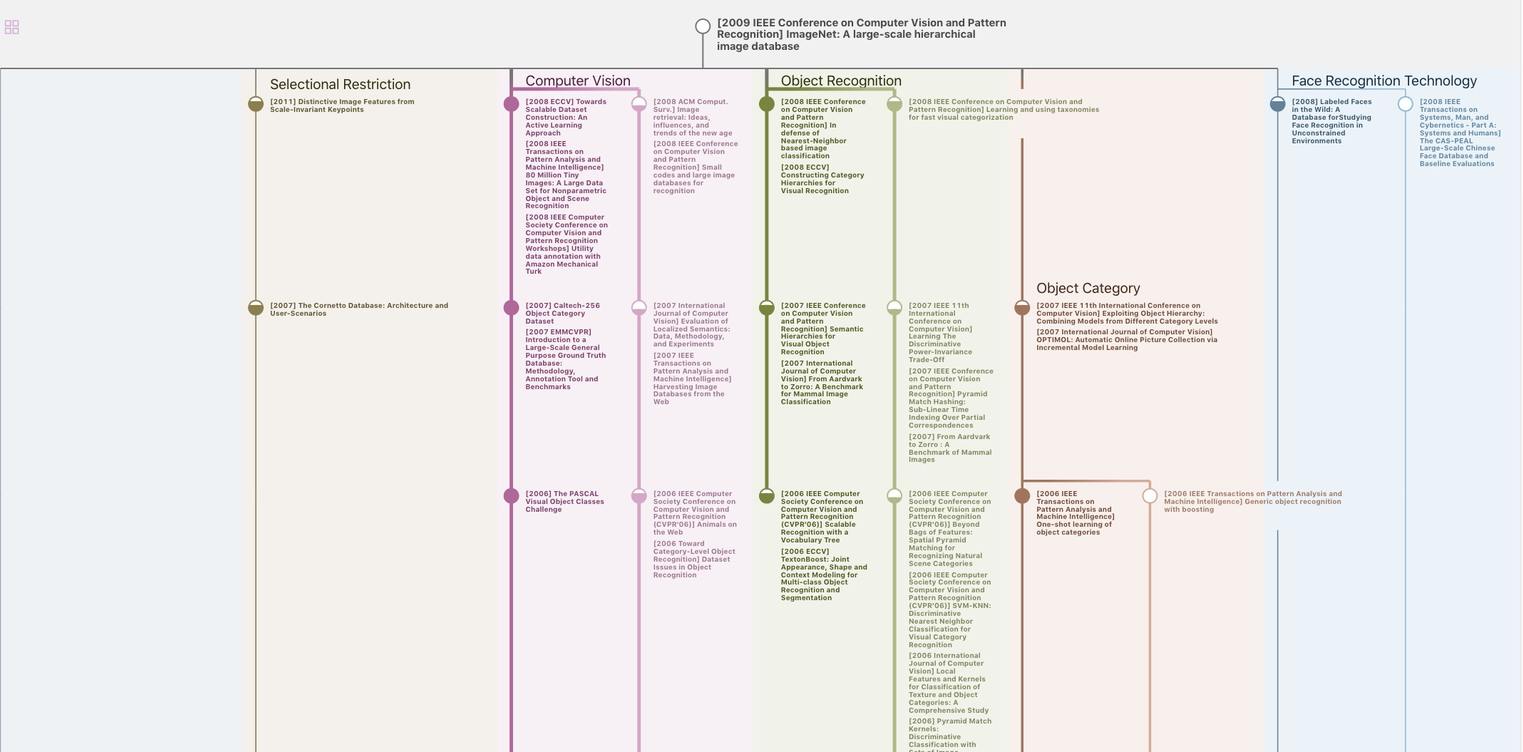
生成溯源树,研究论文发展脉络
Chat Paper
正在生成论文摘要